Early multi-class ensemble-based fake news detection using content features
Soc. Netw. Anal. Min.(2022)
摘要
Nowadays, social media plays an essential role in spreading the news with low cost and high speed in publishing, and easy availability. Given that, anyone can publish any news on social networks, with some of them to be fake. These fake stories should be detected as soon as possible since they might have negative impacts on the society. To address this issue, most researches consider fake news detection as a binary classification problem. However, as some news are half-true, recently, multi-class detection has gained more attention. This paper investigates an early detection of fake news using multi-class classification. This is achieved by extracting the content features, such as sentiment and semantic features, from the news. The proposed model employs five classifiers (Random Forest, Support Vector Machine, Decision Tree, LightGBM, and XGBoost) as primary classifiers. Furthermore, AdaBoost is used for the meta-learning algorithm to develop a stacking generalization model. Stacking generalization is an ensemble learning method that uses all data produced by the first-level algorithms. We trained our model with PolitiFact data for the evaluation, and the model performance was evaluated by Accuracy, Precision, Recall, and F1 score. Excremental evaluation of the real-world datasets showed that our proposed model outperformed all previous works in both binary and multi-class classifications.
更多查看译文
关键词
Natural language processing,Machine learning,Fake news detection,Ensemble learning
AI 理解论文
溯源树
样例
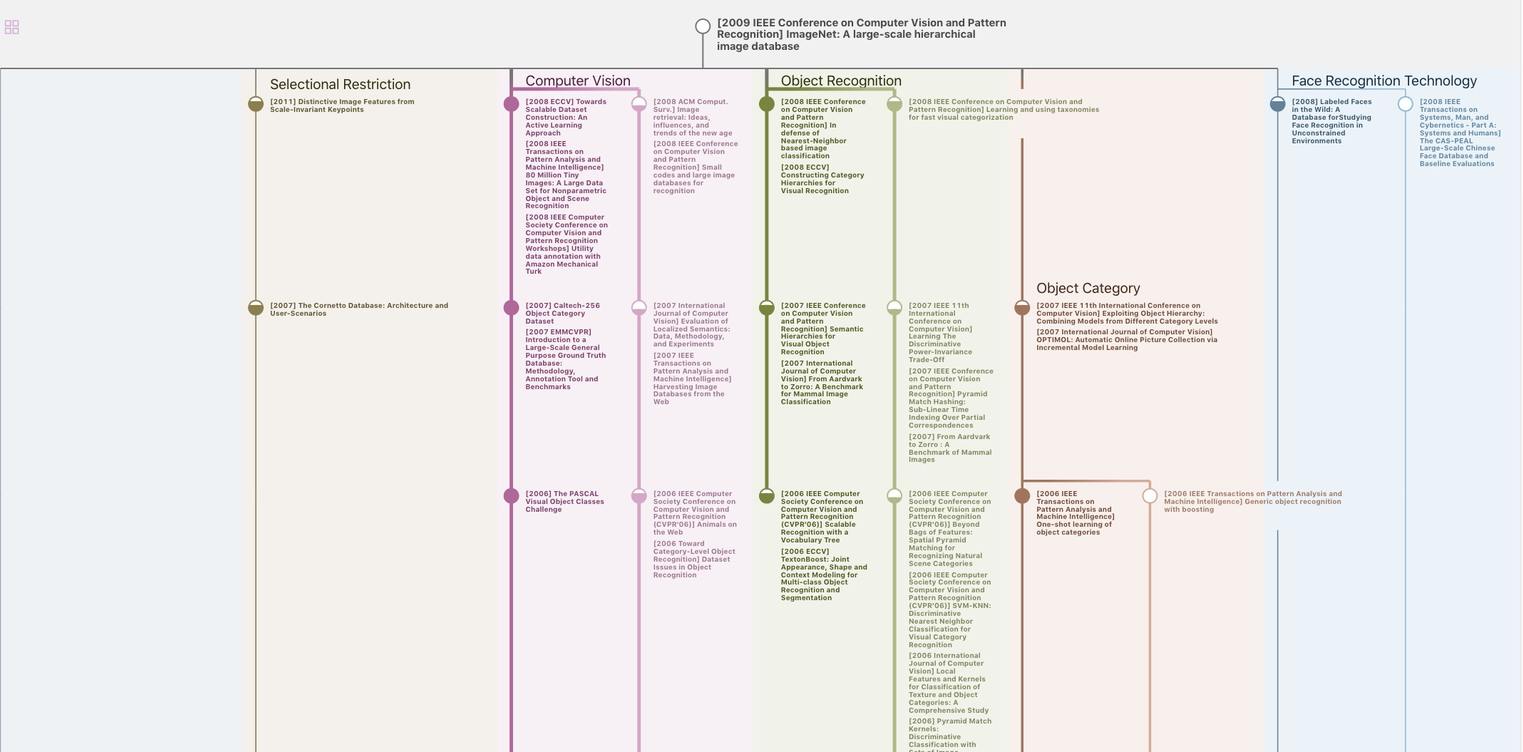
生成溯源树,研究论文发展脉络
Chat Paper
正在生成论文摘要