Deep Ensemble Model for Detecting Attacks in Industrial IoT
International journal of information security and privacy(2022)
摘要
In this research work, a novel IIoT attack detection framework is designed by following four major phases: pre-processing, imbalance processing, feature extraction, and attack detection. The attack detection is carried out using the projected ensemble classification framework. The projected ensemble classification framework encapsulates the recurrent neural network, CNN, and optimized bi-directional long short-term memory (BI-LSTM). The RNN and CNN in the ensemble classification framework is trained with the extracted features. The outcome acquired from RNN and CNN is utilized for training the optimized BI-LSTM model. The final outcome regarding the presence/absence of attacks in the industrial IoT is portrayed by the optimized BI-LSTM model. Therefore, the weight of BI-LSTM model is fine-tuned using the newly projected hybrid optimization model referred as cat mouse updated slime mould algorithm (CMUSMA). The projected hybrids the concepts of both the standard slime mould algorithm (SMA) and cat and mouse-based optimizer(CMBO), respectively.
更多查看译文
关键词
Attack Detection,Cat Mouse Updated Slime Mould Algorithm,Ensemble Classifier,IIoT,Improved K-Means-Based Cluster Oversampling Technique,Security
AI 理解论文
溯源树
样例
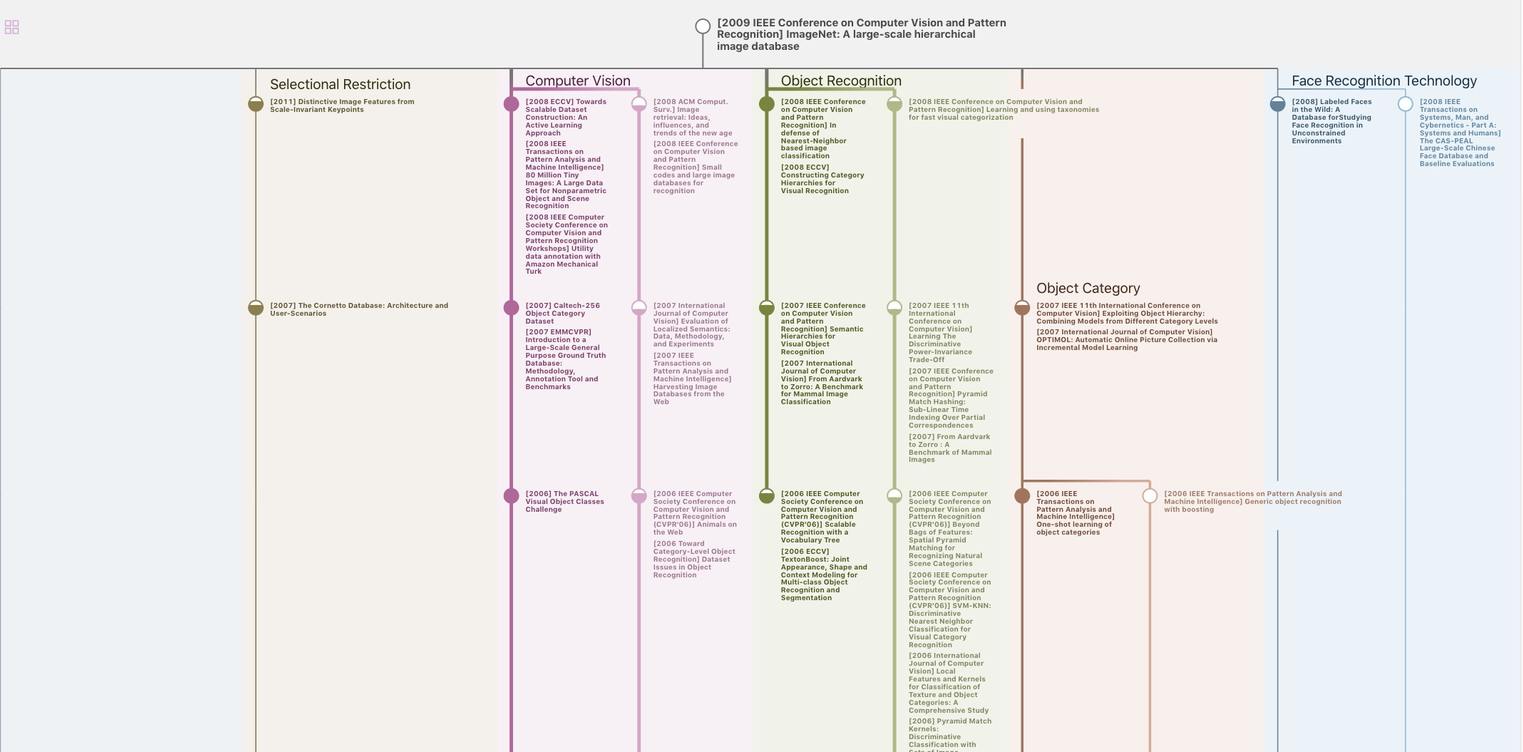
生成溯源树,研究论文发展脉络
Chat Paper
正在生成论文摘要