Robot navigation in crowds via deep reinforcement learning with modeling of obstacle uni-action.
Adv. Robotics(2023)
摘要
Mobile robots operating in public environments require the ability to navigate among humans and obstacles in a socially compliant and safe manner. Previous work has shown the power of deep reinforcement learning (DRL) techniques by employing them to train efficient policies for robot navigation. However, most DRL-based robot navigation methods only consider dynamic pedestrians and do not take static obstacles into account. The ability to approach pedestrians and obstacles differently will improve a robot's navigation efficiency. In this work, we propose a novel network, the obstacle-robot uni-action (ORU) network, to encode the one-way direct effects of obstacles on the robot. Obstacles' indirect effects on the robot, represented by the obstacle-human uni-action (OHU), together with human-human interaction (HHI), are concatenated to a human-robot interaction (HRI) network to obtain the features of a crowd's effects on the robot. We also implement a variable reaching goal reward and an approaching goal reward in our model, which can enhance the method's performance in terms of navigation time. Results of test experiments in both simulation and real datasets demonstrate that the proposed method outperforms state-of-the-art methods.
更多查看译文
关键词
Robot navigation,deep reinforcement learning,human-robot interaction,obstacle-robot uni-action,reward shaping
AI 理解论文
溯源树
样例
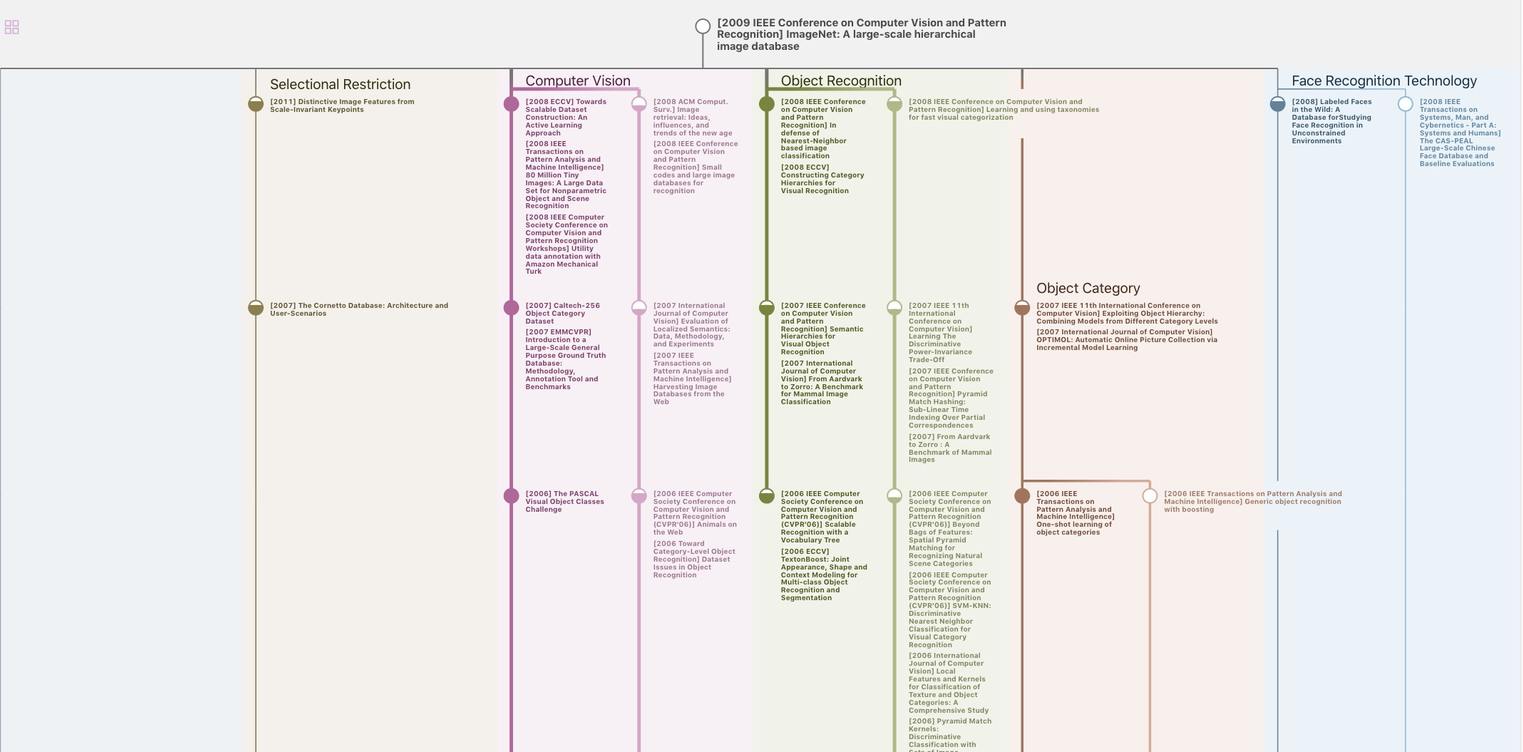
生成溯源树,研究论文发展脉络
Chat Paper
正在生成论文摘要