Data Fusion Methods with Graded Relevance Judgment.
WISA(2022)
摘要
Data fusion methods have been widely used in many information retrieval tasks. Its performance is affected by many factors including the data fusion algorithm used, the component retrieval systems involved, relevance judgment, the metrics used for evaluation, and others. Previously, data fusion research mainly focused on the data fusion methods and the component retrieval systems involved, but other factors such as relevance judgment and the metrics used for evaluation have not been addressed. As a matter of fact, relevance judgment is an important issue that affects many aspects of information retrieval and data fusion. The assumption of binary relevance judgment has been taken for all the previous research work in data fusion. However, this assumption is simplified and not satisfactory in many cases. Instead, graded relevance judgment is more general and able to deal with more complicated requirements. In this paper, we investigate data fusion methods, especially linear combination, to work with graded relevance judgment. Necessary updates are given for using those methods in the new situation. Experimented with two data sets in TREC, we find that data fusion is still an effective technology for performance improvement in general. Many of them are very competitive in a controlled environment, and linear combination with weights trained by multiple linear regression is the most stable in a more complicated environment.
更多查看译文
关键词
fusion,relevance
AI 理解论文
溯源树
样例
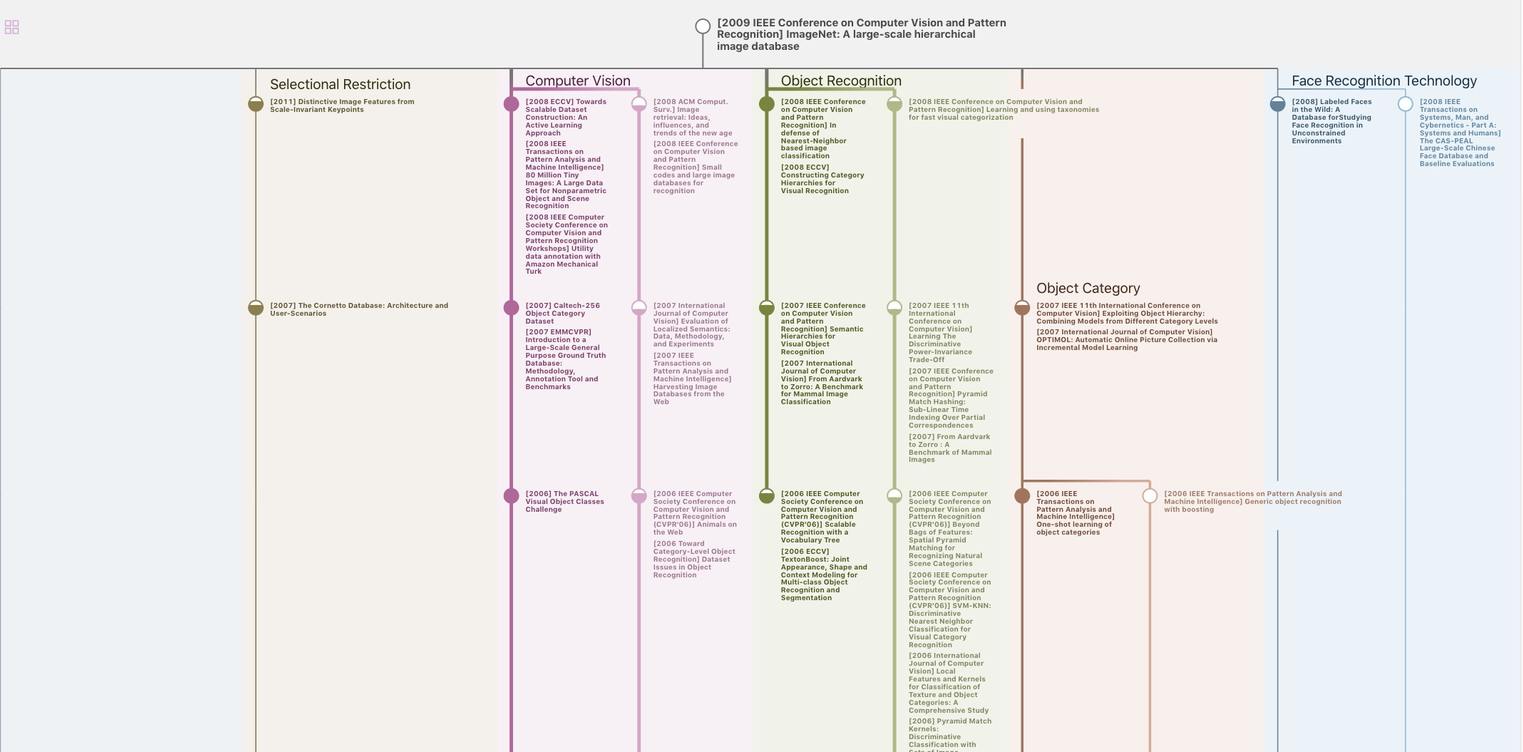
生成溯源树,研究论文发展脉络
Chat Paper
正在生成论文摘要