An In-Depth Analysis of Cooperative Multi-Robot Hierarchical Reinforcement Learning.
SIET(2022)
摘要
In most cases, it is known that Multi-Robot System (MRS) performs more effectively than a Single-Robot System (SRS) in complex environments. Therefore, Deep Reinforcement Learning (DRL) has been gaining interest in training robots to solve tasks in complex environments cooperatively. However, Deep Reinforcement Learning continues to be hampered by challenges in complex environments. Recently, the Multi-Agent Hierarchical Deep Deterministic Policy Gradient (MH-DDPG) has been proposed for implementing hierarchical learning for a group of robots engaged in collaboratively problem-solving. This study provides a more in-depth investigation of the performance of the MH-DDPG algorithm by examining the influence of variations in the number of robots, the sensitivity settings, and the hierarchical levels. The experimental results reveal performance tradeoffs regarding these parameters, which should be considered following the task and environment complexity.
更多查看译文
AI 理解论文
溯源树
样例
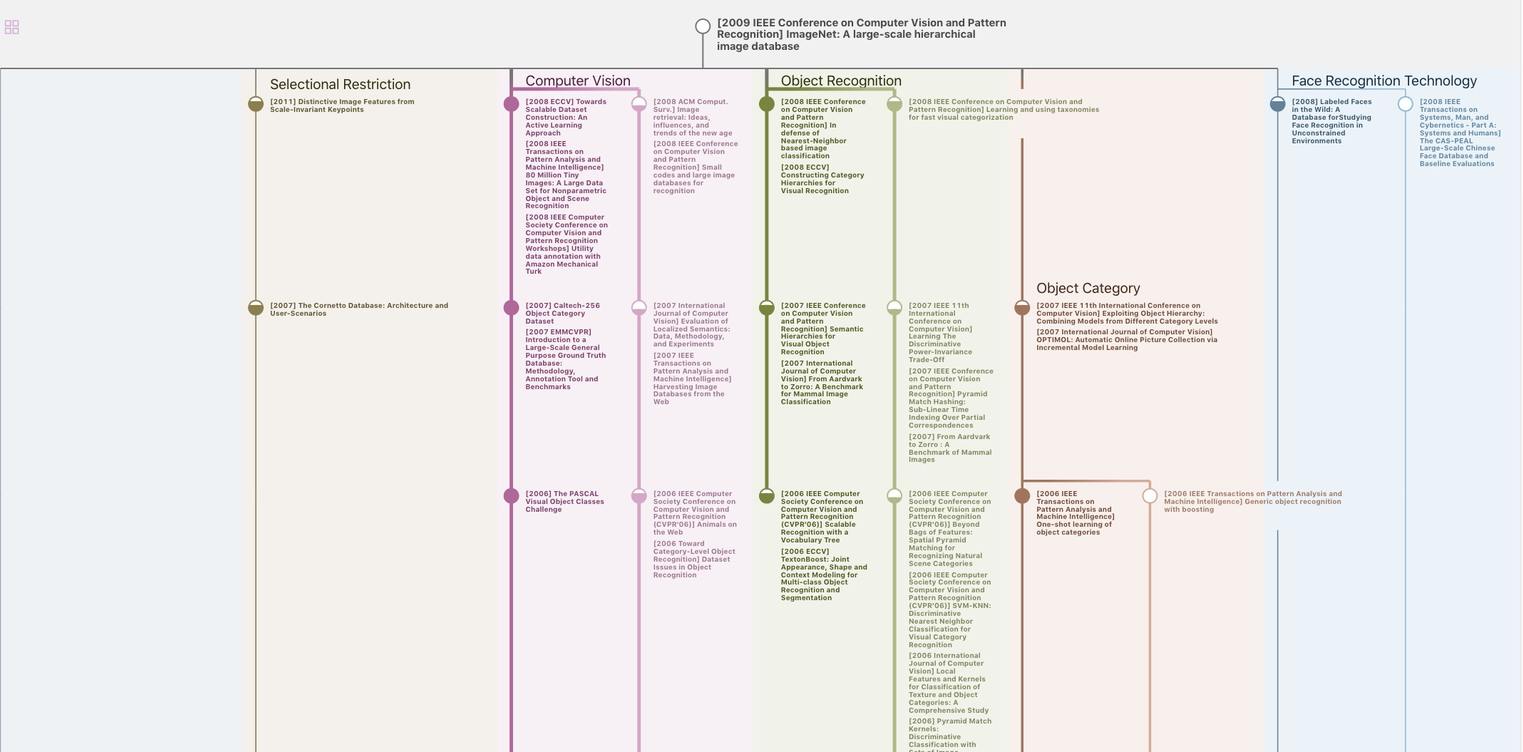
生成溯源树,研究论文发展脉络
Chat Paper
正在生成论文摘要