A Hybrid Hierarchical Navigation Architecture for Highly Dynamic Environments Using Time-Space Optimization
2023 IEEE/SICE INTERNATIONAL SYMPOSIUM ON SYSTEM INTEGRATION, SII(2023)
关键词
baseline navigation systems,collision-free paths,common navigation approaches,complex long-range navigation problems,crowded environments,Deep Reinforcement Learning,different observations,different planning horizons,dynamic obstacle avoidance,dynamic obstacles,extended hybrid A-Star approach,global planners,health care,hierarchical motion,highly dynamic environments,hybrid hierarchical navigation architecture,intermediate planner,learning-based local planner,Learning-based local planners,long planning horizons,mobile robots,myopic nature,optimal solution,standalone system,static environments,subsequent modules,time-space optimization,time-state space,unknown obstacles
AI 理解论文
溯源树
样例
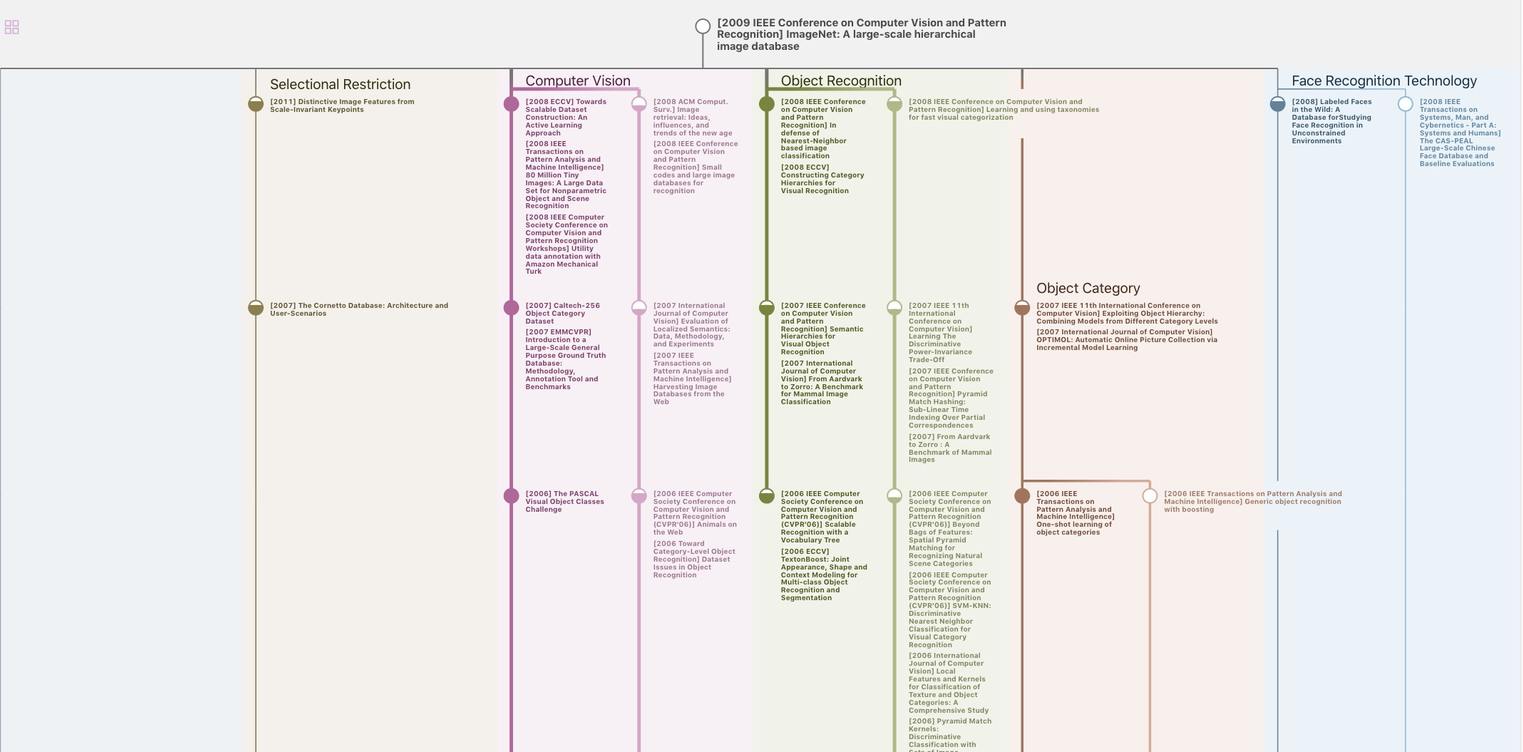
生成溯源树,研究论文发展脉络
Chat Paper
正在生成论文摘要