Adversarial Attacks Against Reinforcement Learning Based Tactical Networks: A Case Study.
MILCOM(2022)
摘要
Dynamic changes caused by conditions such as challenging terrain or hostile encounters force tactical networks to be highly adaptable. To tackle this problem, new proposals implement Reinforcement Learning (RL) based solutions for routing in such complex environments. As high security is another crucial demand for tactical networks, we examine the vulnerability of one such solution against the novel attack vector of adversarial attacks specifically targeting RL algorithms. Utilizing a suite of varying attack methods, we find the targeted solution to be vulnerable to multiple attacks. Further, we found the best attacks to exploit knowledge about the victim agent to a high degree. Lastly, we outline the need for additional research exploring more complex attack strategies to expose the vulnerabilities of other RL proposals for tactical networks. This investigation may also ignite the design/implementation of defensive measures to increase robustness in vulnerable systems.
更多查看译文
关键词
tactical networks,reinforcement learning
AI 理解论文
溯源树
样例
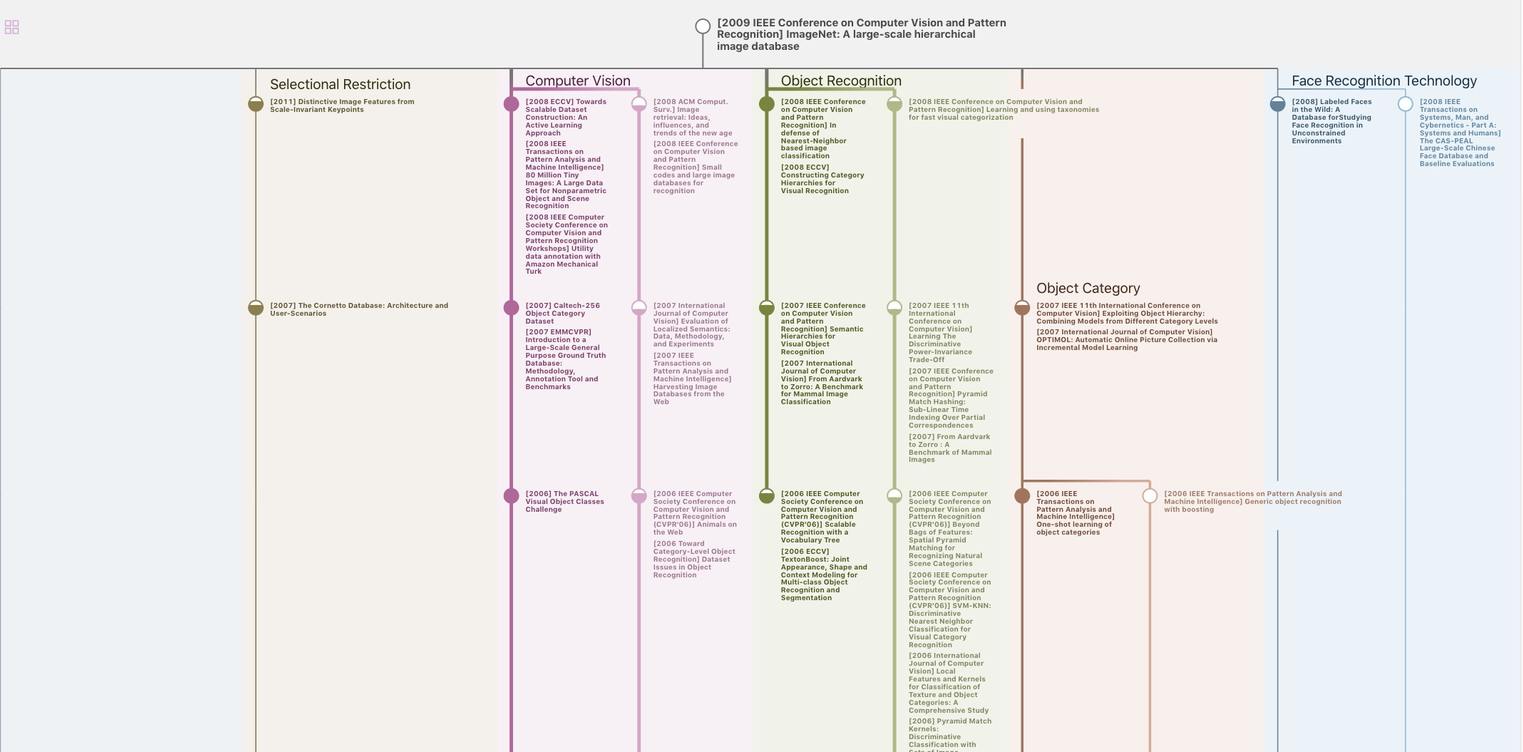
生成溯源树,研究论文发展脉络
Chat Paper
正在生成论文摘要