Knowledge Enhanced BERT Based on Corpus Associate Generation.
ML4CS (3)(2022)
摘要
The pre-training model represented by BERT has limited accuracy due to a lack of professional domain knowledge support. The knowledge-enhanced BERT model effectively improves the lack of knowledge in downstream tasks in different fields by introducing external professional knowledge and has achieved better results than the BERT model. However, the knowledge-enhanced BERT injected large-scale external knowledge, resulting in a sharp increase in computing resource requirements and training time. To solve this problem, a knowledge enhancement method based on corpus associate generation (CAG) is proposed. The input text corpus is disassembled into a sequential sequence of word sets and entity noun sets through entity noun recognition, and the word sets are associated with external knowledge triples through entity nouns. Using semantic splicing to generate corpus with external knowledge. Finally, the original BERT model structure is used to inject the corpus containing knowledge into the downstream tasks for knowledge enhancement, avoiding the introduction of additional model structures to increase the model complexity, to achieve the purpose of reducing the amount of calculation. Experimental analysis with 6 public data sets such as LCQMC and XNLI, the results show that compare to K-BERT, the average calculation time of knowledge introduction and training is reduced by 53.5% and 37.4%.
更多查看译文
关键词
corpus,knowledge
AI 理解论文
溯源树
样例
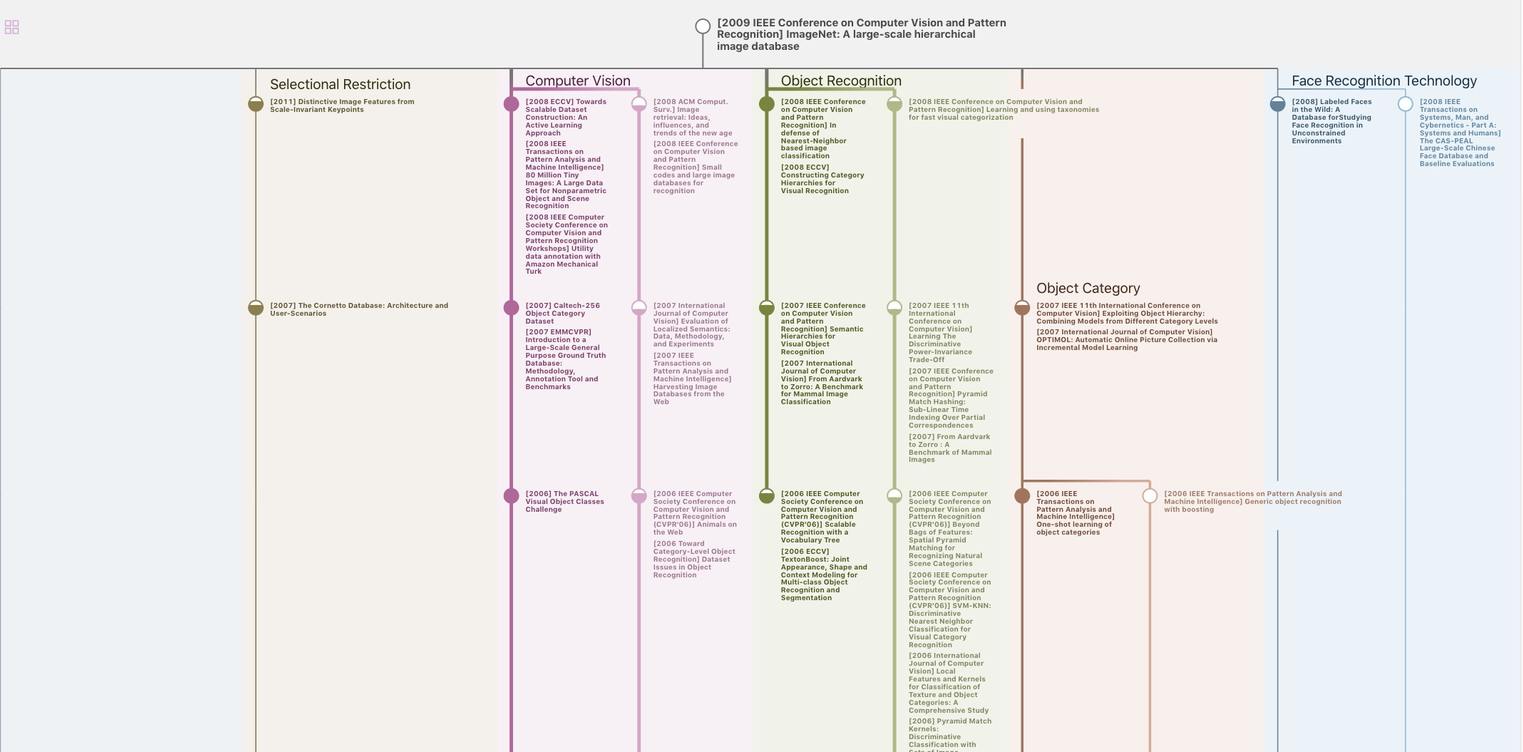
生成溯源树,研究论文发展脉络
Chat Paper
正在生成论文摘要