A Hybrid Secure Two-Party Protocol for Vertical Federated Learning.
UbiSec(2022)
摘要
Federated learning (FL) is a promising distributed machine learning technique for solving the privacy leakage problem in machine learning training process. Multiple parties collaborate to train a machine learning model, while the data is kept locally, so as to achieve privacy-preservation of users. However, some studies have shown that an attacker can infer some sensitive information from the interacted data, even if the raw data is not available. It is necessary to adopt some privacy-preserving techniques such as secure multi-party computation and homomorphic encryption to prevent the above problem. Most existing solutions have focused on FL over horizontally partitioned data but ignore the research on FL over vertically partitioned data. In this paper, we present a vertical federated learning scheme with enhanced privacy preservation. Split learning is used to enable cooperative neural network training between multiple parties with vertically partitioned datasets, and a hybrid secure two-party computation protocol is adopted for protecting users’ privacy. The experimental results demonstrate the feasibility of our scheme.
更多查看译文
关键词
Vertical federated learning, Split learning, Secure two-party computation, Privacy-preservation
AI 理解论文
溯源树
样例
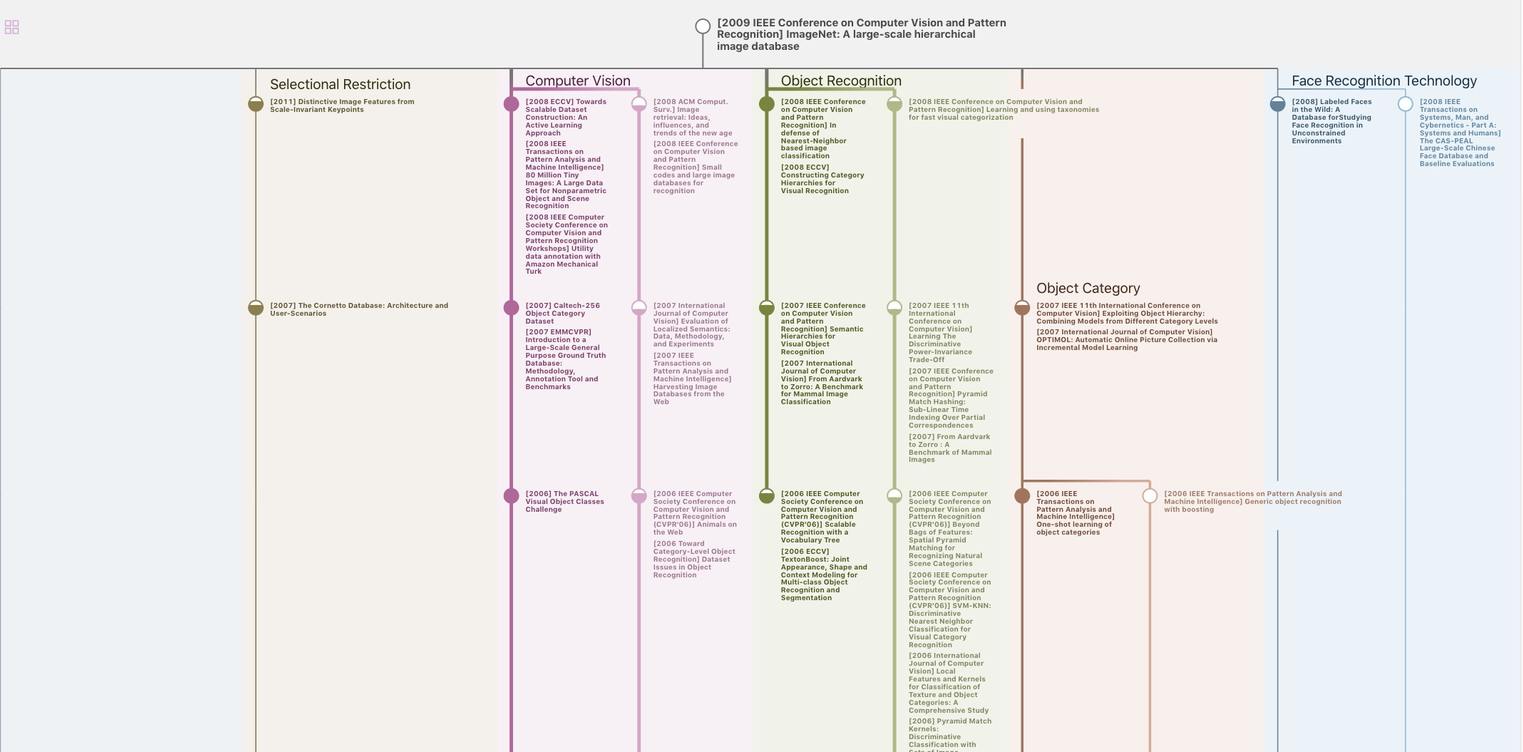
生成溯源树,研究论文发展脉络
Chat Paper
正在生成论文摘要