System Call Processing Using Lightweight NLP for IoT Behavioral Malware Detection.
UbiSec(2022)
摘要
Although much of the work in behaviorally detecting malware lies in collecting the best explanatory data and using the most efficacious machine learning models, the processing of the data can sometimes prove to be the most important step in the data pipeline. In this work, we collect kernel-level system calls on a resource-constrained Internet of Things (IoT) device, apply lightweight Natural Language Processing (NLP) techniques to the data, and feed this processed data to two simple machine learning classification models: Logistic Regression (LR) and a Neural Network (NN). For the data processing, we group the system calls into n-grams that are sorted by the timestamp in which they are recorded. To demonstrate the effectiveness, or lack thereof, of using n-grams, we deploy two types of malware onto the IoT device: a Denial-of-Service (DoS) attack, and an Advanced Persistent Threat (APT) malware. We examine the effects of using lightweight NLP on malware like the DoS and the stealthy APT malware. For stealthier malware, such as the APT, using more advanced, but far more resource-intensive, NLP techniques will likely increase detection capability, which is saved for future work.
更多查看译文
关键词
system call processing,malware,iot,lightweight nlp
AI 理解论文
溯源树
样例
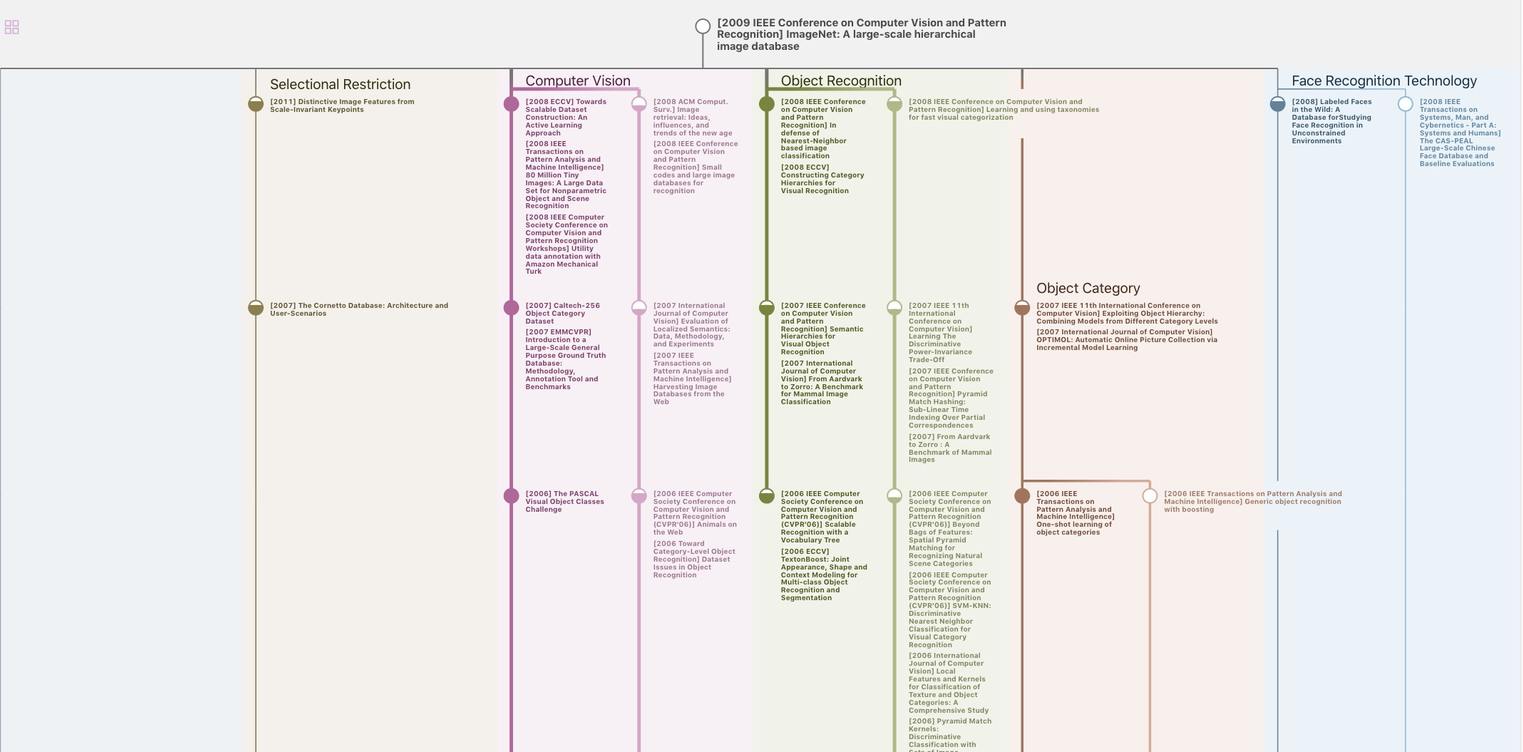
生成溯源树,研究论文发展脉络
Chat Paper
正在生成论文摘要