Towards Improved Intermediate Layer Variational Inference for Uncertainty Estimation.
ECCV Workshops (5)(2022)
摘要
DNNs have been excelling on many computer vision tasks, achieving many milestones and are continuing to prove their validity. It is important for DNNs to express their uncertainties to be taken into account for the whole application output, whilst maintaining their performance. The intermediate layer variational inference has been a promising approach to estimate uncertainty in real-time beating state-of-the-art approaches. In this work, we propose an enhancement of the intermediate layer variational inference by adding Dirichlet distribution to the network architecture. This improves, on one hand, the uncertainty estimation reliability and on the other hand can detect out-of-distribution samples. Results show that with the addition of Dirichlet distributions the DNN is able to maintain its segmentation performance whilst boosting its uncertainty estimation capabilities.
更多查看译文
关键词
Deep neural networks, Uncertainty estimation, Trustworthiness, Out-of-distribution detection
AI 理解论文
溯源树
样例
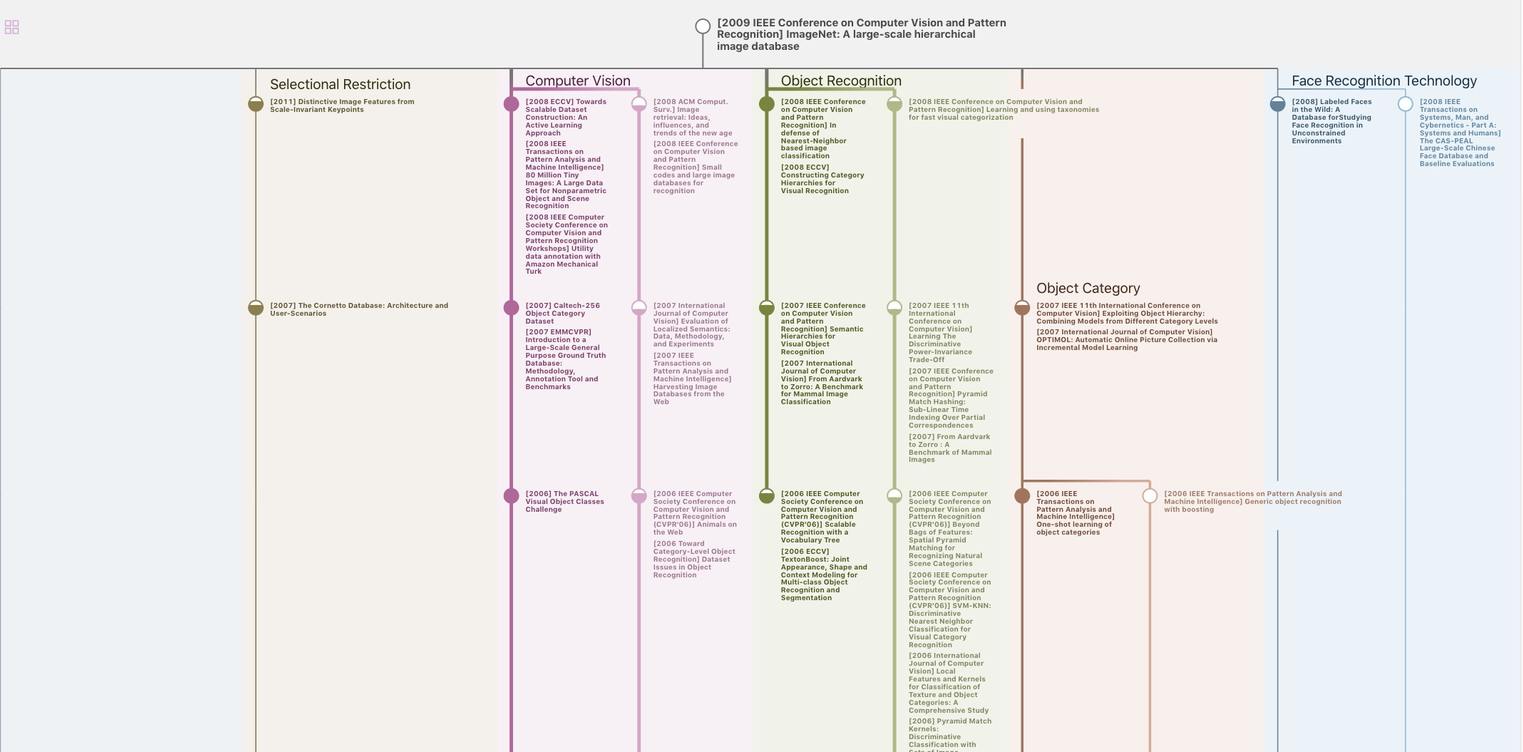
生成溯源树,研究论文发展脉络
Chat Paper
正在生成论文摘要