Self-attention Capsule Network for Tissue Classification in Case of Challenging Medical Image Statistics.
ECCV Workshops (3)(2022)
摘要
We propose the first Self-Attention Capsule Network that was designed to deal with unique core challenges of medical imaging, specifically for tissue classification. These challenges are - significant data heterogeneity with statistics variability across imaging domains, insufficient spatial context and local fine-grained details, and limited training data. Moreover, our proposed method solves limitations of the baseline Capsule Networks (CapsNet) such as handling complicated challenging data and limited computational resources. To cope with these challenges, our method is composed of a self-attention module that simplifies the complexity of the input data such that the CapsNet routing mechanism can be efficiently used, while extracting much richer contextual information, compared with CNNs. To demonstrate the strengths of our method, it was extensively evaluated on three diverse medical datasets and three natural benchmarks. The proposed method outperformed other methods we compared with in classification accuracy but also in robustness, within and across different datasets and domains.
更多查看译文
关键词
tissue classification,capsule,self-attention
AI 理解论文
溯源树
样例
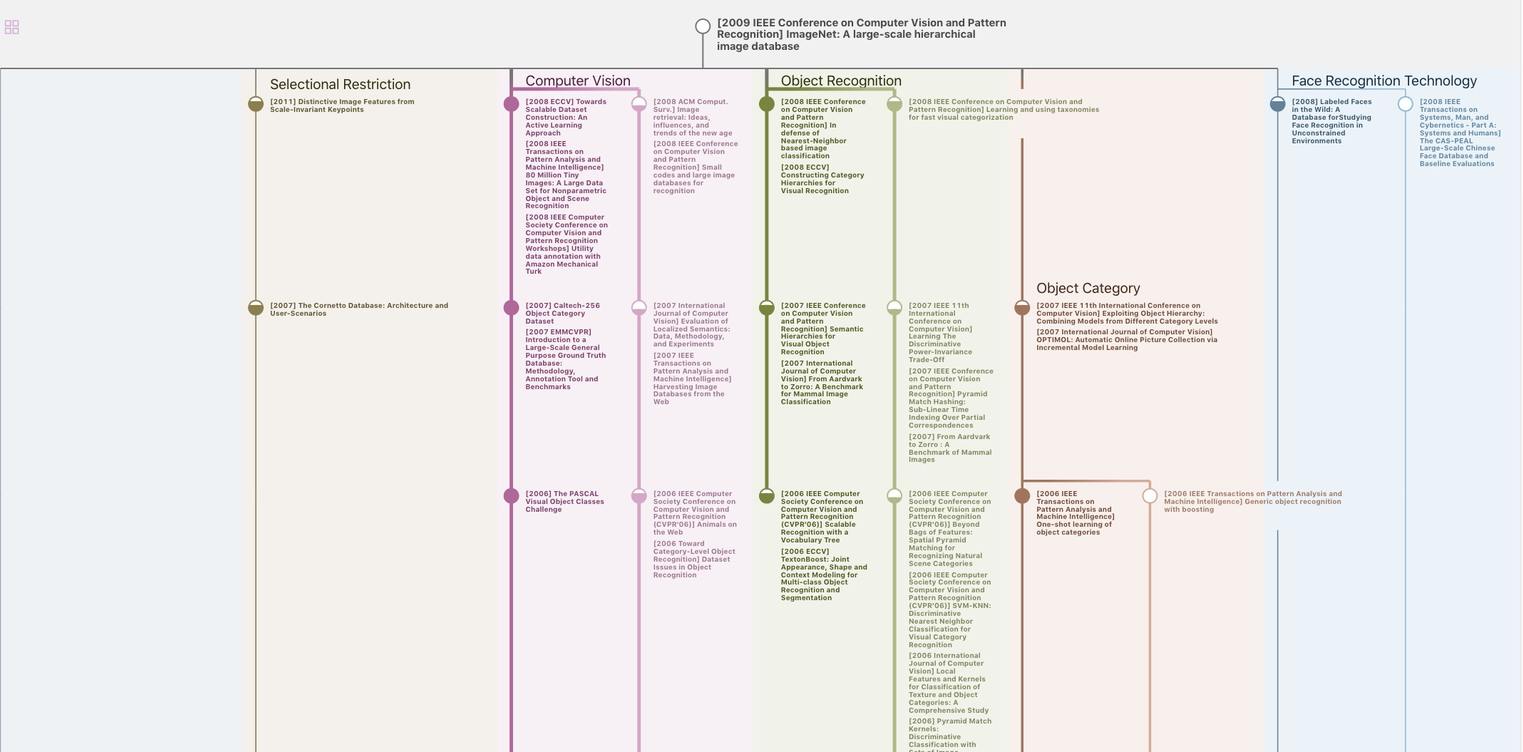
生成溯源树,研究论文发展脉络
Chat Paper
正在生成论文摘要