Power Awareness in Low Precision Neural Networks.
ECCV Workshops (7)(2022)
摘要
Existing approaches for reducing DNN power consumption rely on quite general principles, including avoidance of multiplication operations and aggressive quantization of weights and activations. However, these methods do not consider the precise power consumed by each module in the network and are therefore not optimal. In this paper we develop accurate power consumption models for all arithmetic operations in the DNN, under various working conditions. We reveal several important factors that have been overlooked to date. Based on our analysis, we present PANN (power-aware neural network), a simple approach for approximating any full-precision network by a low-power fixed-precision variant. Our method can be applied to a pre-trained network and can also be used during training to achieve improved performance. Unlike previous methods, PANN incurs only a minor degradation in accuracy w.r.t. the full-precision version of the network and enables to seamlessly traverse the power-accuracy trade-off at deployment time.
更多查看译文
关键词
power awareness,low precision neural networks,neural networks
AI 理解论文
溯源树
样例
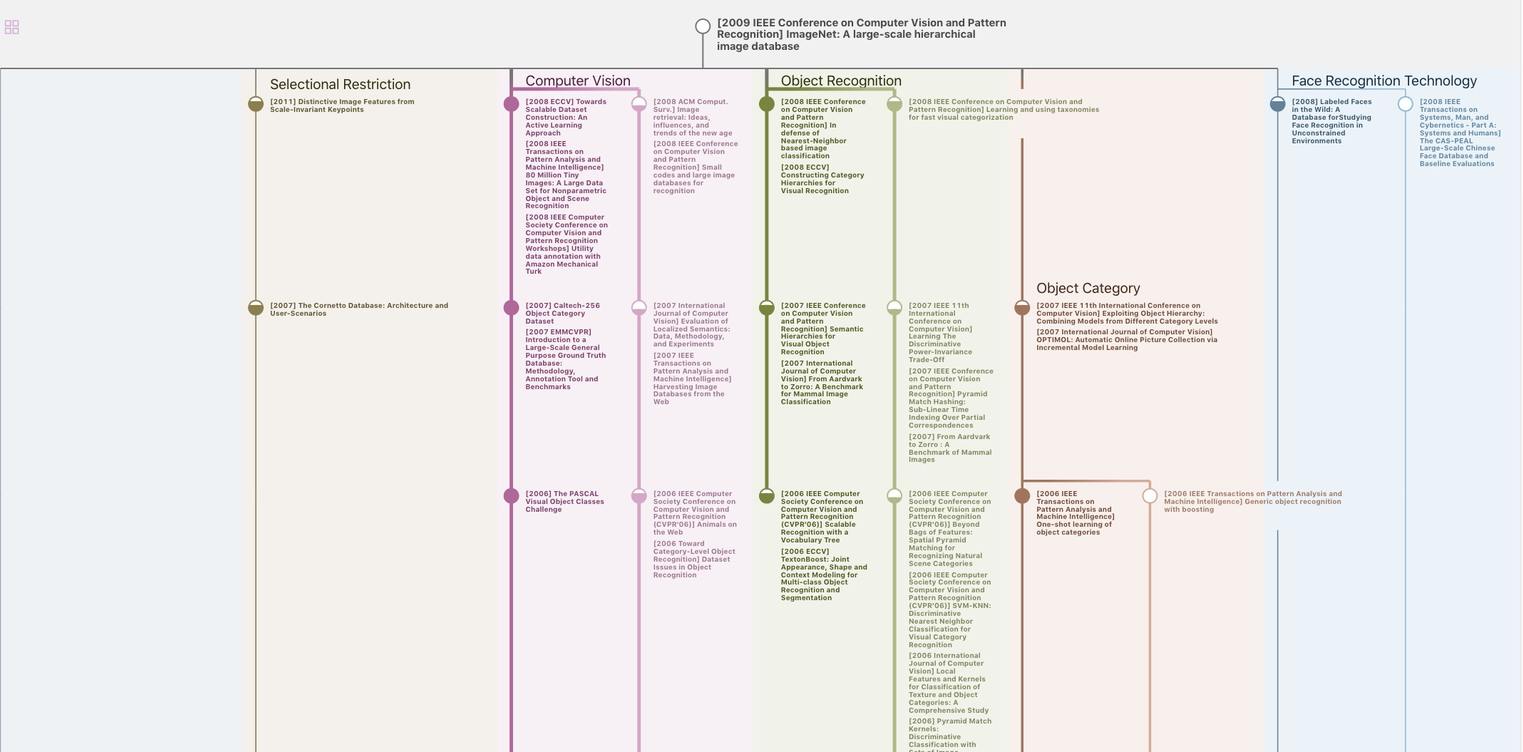
生成溯源树,研究论文发展脉络
Chat Paper
正在生成论文摘要