Searching for N:M Fine-grained Sparsity of Weights and Activations in Neural Networks
ECCV Workshops (7)(2023)
摘要
Sparsity in deep neural networks has been extensively studied to compress and accelerate models for environments with limited resources. The general approach of pruning aims at enforcing sparsity on the obtained model, with minimal accuracy loss, but with a sparsity structure that enables acceleration on hardware. The sparsity can be enforced on either the weights or activations of the network, and existing works tend to focus on either one for the entire network. In this paper, we suggest a strategy based on Neural Architecture Search (NAS) to sparsify both activations and weights throughout the network, while utilizing the recent approach of N:M fine-grained structured sparsity that enables practical acceleration on dedicated GPUs. We show that a combination of weight and activation pruning is superior to each option separately. Furthermore, during the training, the choice between pruning the weights of activations can be motivated by practical inference costs (e.g., memory bandwidth). We demonstrate the efficiency of the approach on several image classification datasets.
更多查看译文
关键词
Neural architecture search,Weight pruning,Activation pruning,N:M fine-grained Sparsity
AI 理解论文
溯源树
样例
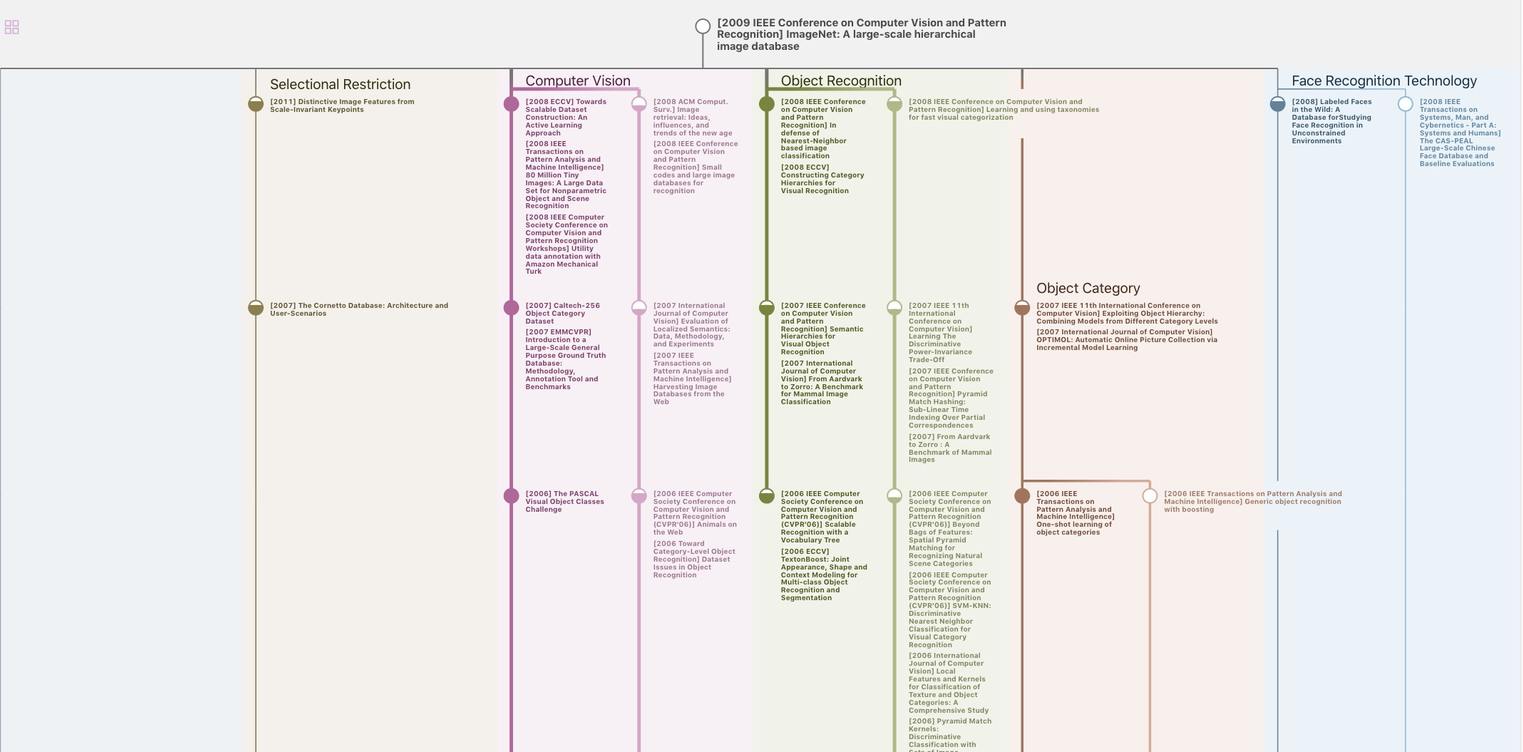
生成溯源树,研究论文发展脉络
Chat Paper
正在生成论文摘要