Investigating Neural Network Training on a Feature Level Using Conditional Independence.
ECCV Workshops (6)(2022)
摘要
There are still open questions about how the learned representations of deep models change during the training process. Understanding this process could aid in validating the training. Towards this goal, previous works analyze the training in the mutual information plane. We use a different approach and base our analysis on a method built on Reichenbach’s common cause principle. Using this method, we test whether the model utilizes information contained in human-defined features. Given such a set of features, we investigate how the relative feature usage changes throughout the training process. We analyze multiple networks training on different tasks, including melanoma classification as a real-world application. We find that over the training, models concentrate on features containing information relevant to the task. This concentration is a form of representation compression. Crucially, we also find that the selected features can differ between training from-scratch and finetuning a pre-trained network.
更多查看译文
关键词
neural network training,conditional independence,neural network,feature level
AI 理解论文
溯源树
样例
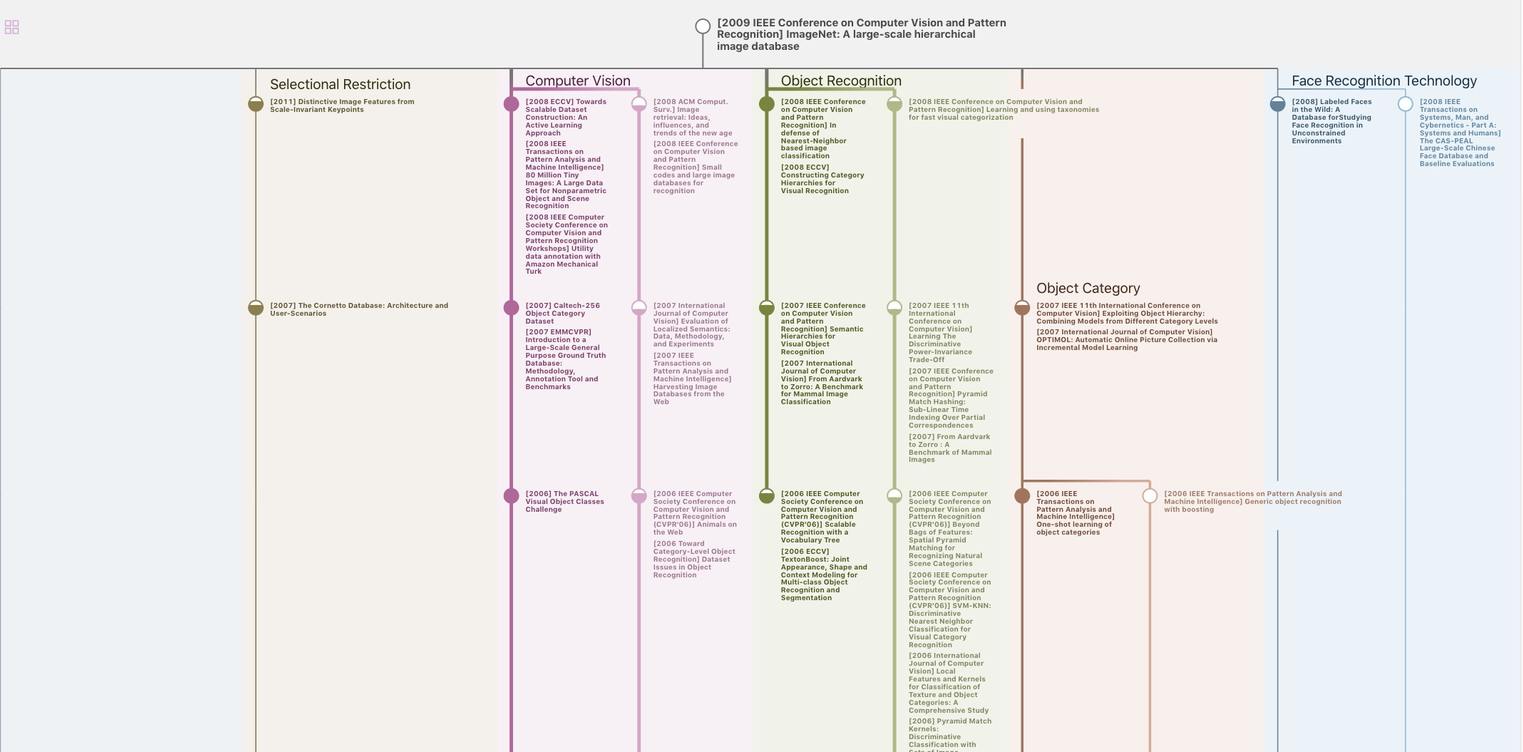
生成溯源树,研究论文发展脉络
Chat Paper
正在生成论文摘要