Shuffle Attention Multiple Instances Learning for Breast Cancer Whole Slide Image Classification.
ICIP(2022)
摘要
Multiple instance learning (MIL) has recently become a powerful tool to solve the weakly supervised classification problem on whole slide image (WSI) pathological diagnosis. However, current MIL methods lie in two drawbacks: 1) seldom depicting feature dependencies in multiple dimensions, 2) limitation on capturing dependencies of selected instances for predicting bag-level results. To address these issues, this work presents a novel two-stage shuffle attention MIL (SAMIL) model for breast cancer WSI classification. SAMIL first introduces shuffle attention to extract important features from both spatial and channel dimensions, which well includes pixel-level pairwise relationships and channel dependencies, thus helping select more discriminant breast cancer instances for bag-level prediction. Additionally, it stacks multi-head attention with long short-term memory (LSTM) to construct an aggregator, and this adaptively highlights the most distinctive instance features while exploring the correlation between selected breast cancer instances more effectively. Experiment results on the Camelyon-16 dataset demonstrate its superior performance compared with the state-of-the-art MIL methods. The code is available at https://github.com/CunqiaoHou/SAMIL.
更多查看译文
关键词
WSI classification,breast cancer,MIL,attention mechanism,LSTM
AI 理解论文
溯源树
样例
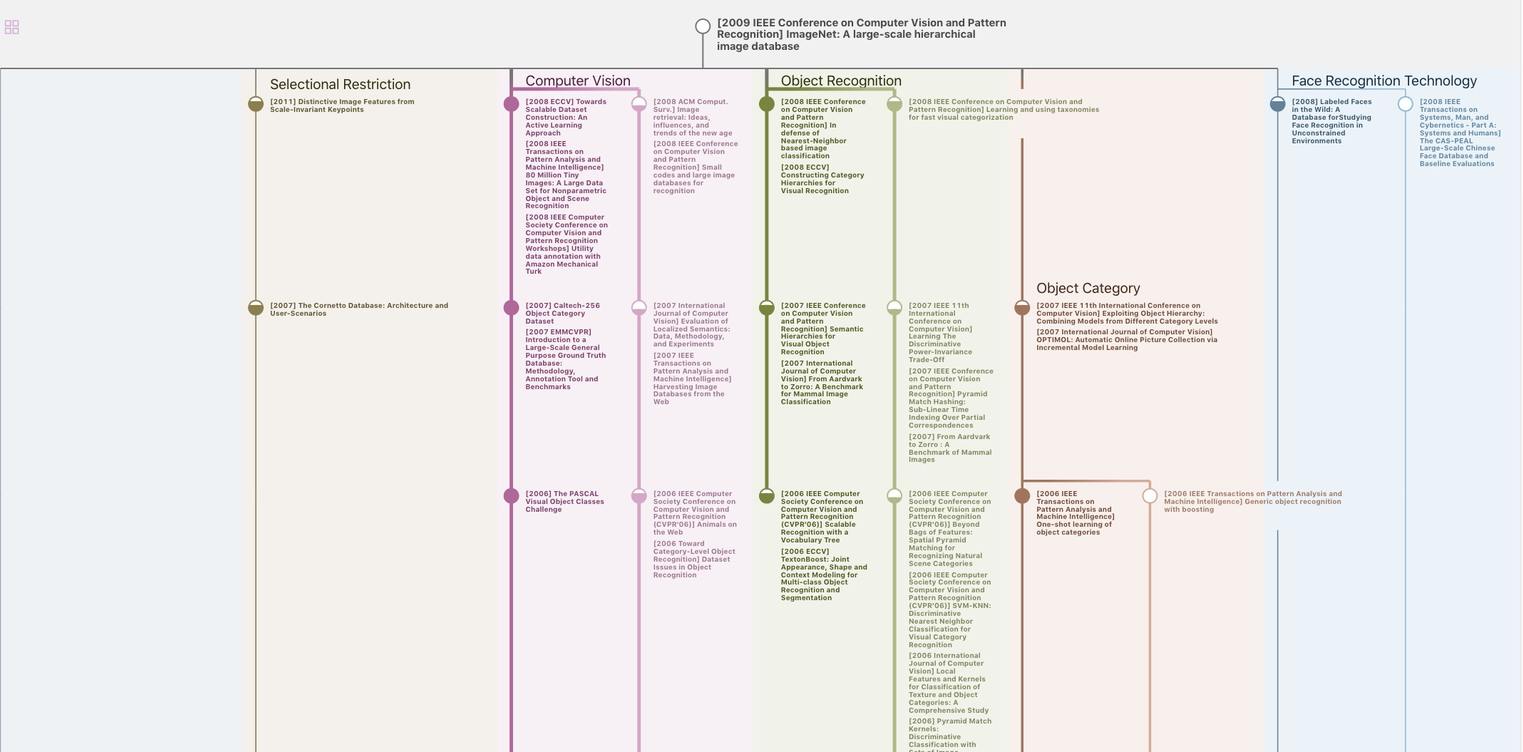
生成溯源树,研究论文发展脉络
Chat Paper
正在生成论文摘要