Mixture of Teacher Experts for Source-Free Domain Adaptive Object Detection.
ICIP(2022)
摘要
Unsupervised domain adaptive object detection methods transfer knowledge from the labelled source domain to a visually distinct and unlabeled target domain. Most methods achieve this by training the detector model with the help of both labeled source and unlabeled target data. However, in real-world scenarios, gaining access to source data is not practical due to privacy concerns, legal issues and inefficient data transmission. To this end, we tackle the problem of Source-Free Domain Adaptive Object Detection, where during adaptation, we do not have access to the source data but only the source trained model. Specifically, we introduce Mixture of Teacher Experts (MoTE) method, where our key idea is to exploit the prediction uncertainty through a mixture of teacher models and progressively train the student model. We evaluate the proposed method by conducting extensive experiments on several object detection benchmark datasets to demonstrate the effectiveness of the proposed mixture of teacher expert based student-teacher training, specifically for source-free adaptation.
更多查看译文
关键词
Object detection, Domain adaptation, Source-Free domain adaptation, Student-teacher, Pseudo-labels
AI 理解论文
溯源树
样例
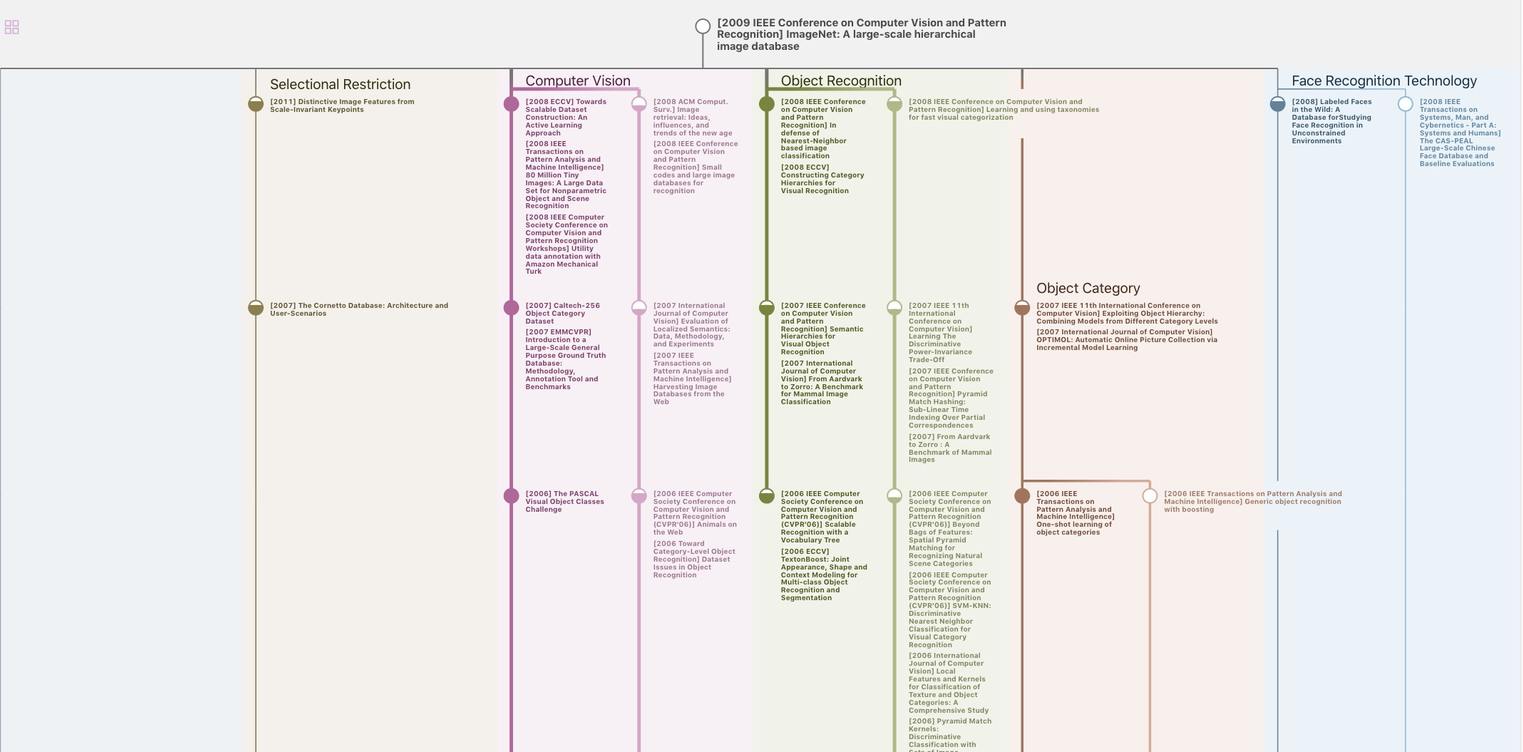
生成溯源树,研究论文发展脉络
Chat Paper
正在生成论文摘要