Active Learning for Hyperspectral Image Classification via Hypergraph Neural Network.
ICIP(2022)
摘要
Graph convolution network (GCN) has been extensively applied to the area of hyperspectral image (HSI) classification. However, the graph can not effectively describe the complex relationships between HSI pixels and the GCN still faces the challenge of insufficient labeled pixels. In order to alleviate the above two issues faced by the GCN in HSI classification, we propose a novel framework that integrates the active learning and the hypergraph neural network. First, we construct a hypergraph that can reveal the complex non-pairwise relationships embedded in the hyperspectral images. Next, we train a semi-supervised hypergraph neural network (GNN) with the fewer labeled training set. Then, exploiting the local structural properties of the hypergraph, the most useful HSI pixels are actively selected for labeling. Finally, we fine-tune the GNN with original training set along with the newly labeled pixels. And the last three steps are iteratively carried on. Compared with the other traditional and active learning approaches of HSI classification, the proposed active hypergraph neural network (ACGNN) can achieve better performance on the three HSI datasets.
更多查看译文
关键词
hyperspectral image classification,hypergraph neural network,neural network,learning
AI 理解论文
溯源树
样例
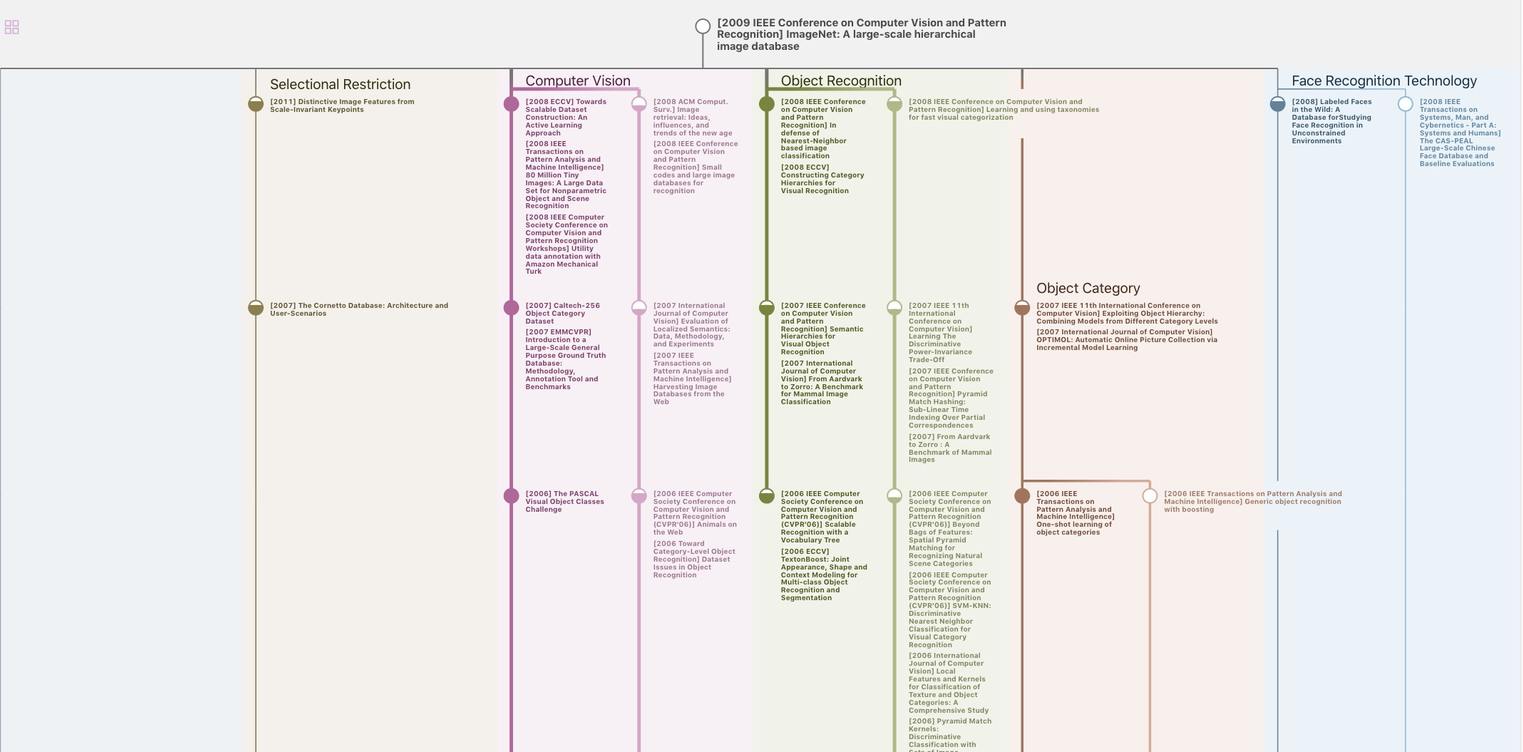
生成溯源树,研究论文发展脉络
Chat Paper
正在生成论文摘要