U-Deepdig: Scalable Deep Decision Boundary Instance Generation.
2022 IEEE International Conference on Image Processing (ICIP)(2022)
摘要
For more than a decade, deep learning algorithms have consistently achieved and improved upon the state-of-the-art performance on image classification tasks. However, there is a general lack of understanding and knowledge about the decision boundaries carved by these modern deep neural network architectures. Recently, an algorithm called DeepDIG was introduced to generate boundary instances between two classes based on the decision regions defined by any deep neural network classifier. Although it is very effective in generating boundary instances, the underlying algorithm was designed to work with two classes at a time in a non-commutative fashion which makes it ill-suited for multi-class problems with hundreds of classes. In this work, we extend the DeepDIG algorithm so that it scales linearly with the number of classes. We show that the proposed U-DeepDIG algorithm maintains the efficacy of the original DeepDIG algorithm while being scalable and more efficient when applied to larger classification problems. We demonstrate this by applying our algorithm on MNIST, Fashion-MNIST and CIFAR10 datasets. In addition to qualitative comparisons, we also perform extensive quantitative comparison by analyzing the margin between the class boundaries and the instances generated.
更多查看译文
关键词
boundary instances,class boundaries,classification problems,decision regions,deep learning algorithms,deep neural network architectures,deep neural network classifier,image classification tasks,multiclass problems,original DeepDIG algorithm,scalable deep decision boundary instance generation,U-DeepDIG algorithm
AI 理解论文
溯源树
样例
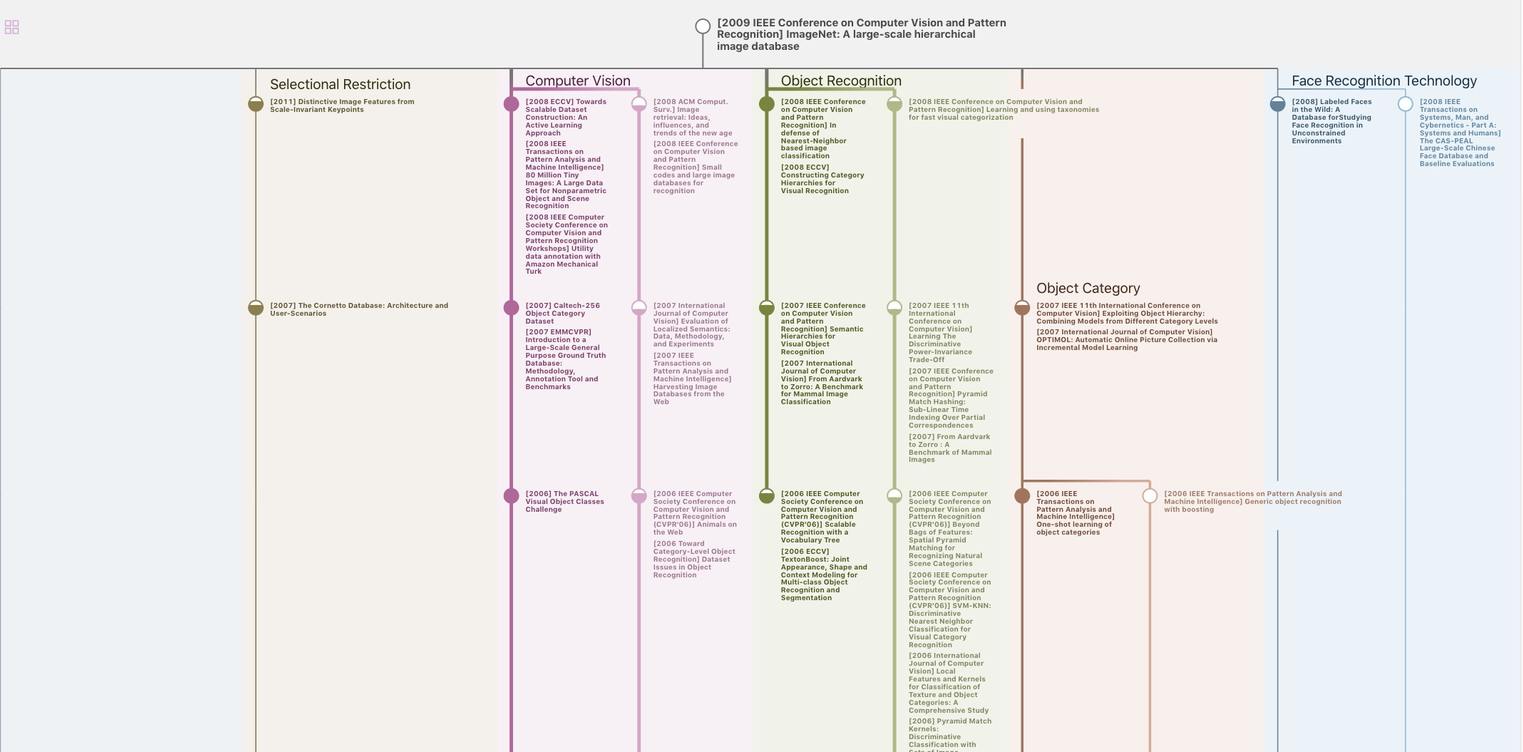
生成溯源树,研究论文发展脉络
Chat Paper
正在生成论文摘要