Bi-Directional Inter-Prediction For Geometry-Based Point Cloud Compression.
ICIP(2022)
摘要
Efficient 3D point cloud compression plays critical role in immersive multimedia presentation and autonomous driving. The temporal redundancy between consecutive point cloud frames is obvious, but the exploration of inter-prediction is insufficient in the geometry-based point cloud compression (G-PCC) framework. In inter exploration model (Inter-EM) of G-PCC, the reference information can only come from one previous frame and a consistent quantization parameter (QP) value is used for all frames. To perform a more efficient interprediction for geometry and attribute, a bidirectional interprediction (bi-prediction) scheme is proposed for G-PCC based on Inter-EM. With the bi-prediction scheme, the reference information can come from two reference frames. For attribute compression, neighboring search is started from one search center derived by a Morton code distance and a two-threshold method is applied to constrain inter-prediction. Meanwhile, the dependencies between frames are designed according to a hierarchical group of frames (GOF) structure with corresponding hierarchical QP values. Experimental results demonstrate that our method outperforms G-PCC and Inter-EM for both lossless and lossy compression. More specifically, the average coding gain over G-PCC is 14.1% on attribute and 11.8% on geometry under lossy condition. The designs on attribute compression have been adopted to the latest Inter-EM.
更多查看译文
关键词
point cloud compression, Inter-EM, bi-prediction G-PCC, hierarchical GOF structure, hierarchical QP values
AI 理解论文
溯源树
样例
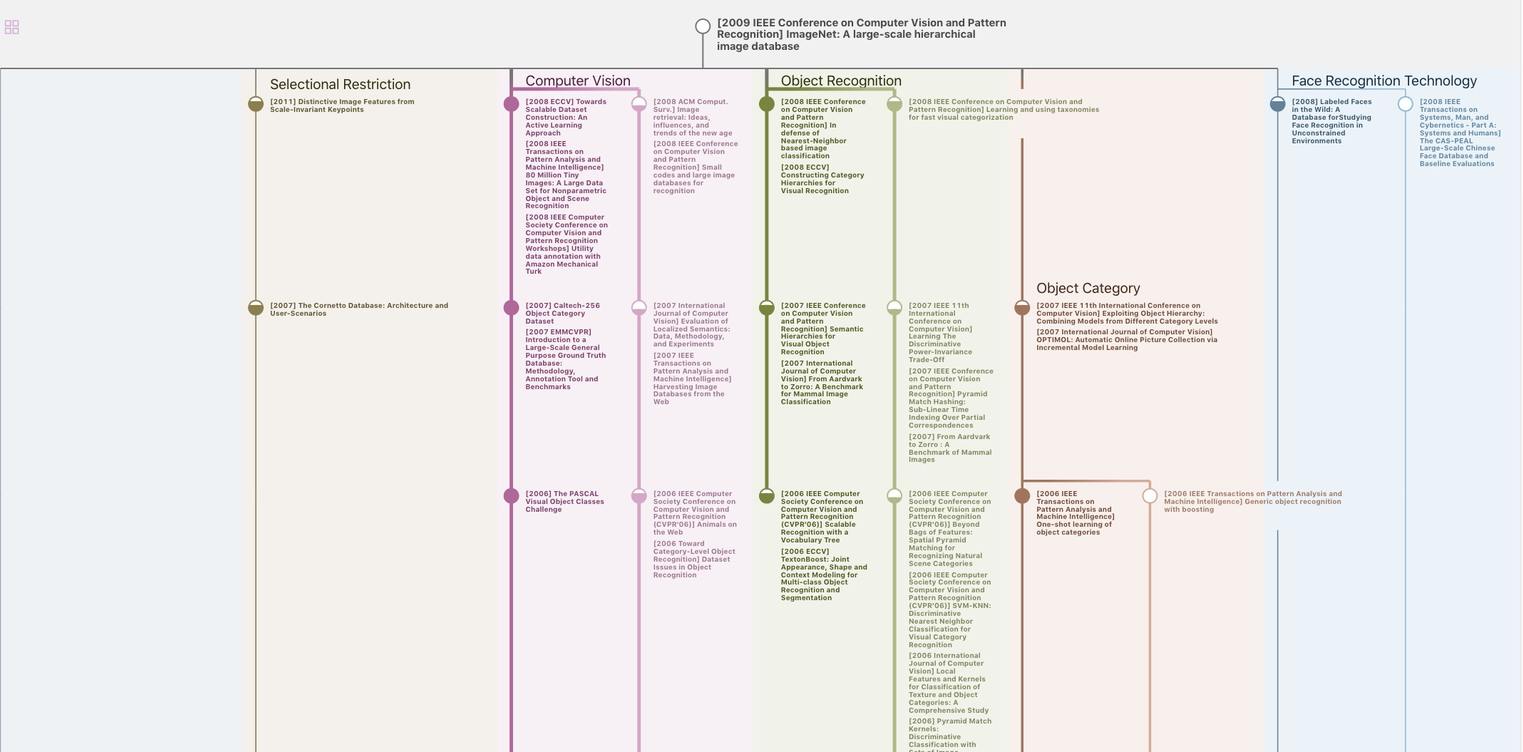
生成溯源树,研究论文发展脉络
Chat Paper
正在生成论文摘要