Self-supervised Out-of-Distribution Detection with Dynamic Latent Scale GAN.
S+SSPR(2022)
摘要
Dynamic latent scale GAN is a learning-based GAN inversion method with maximum likelihood estimation. In this paper, we propose a self-supervised out-of-distribution detection method using the encoder of dynamic latent scale GAN. When the dynamic latent scale GAN converges, since the entropy of the scaled latent random variable is optimal to represent in-distribution data, indistribution data are densely mapped to latent codes with high likelihood. Therefore, out-of-distribution data can only be mapped to latent codes with low likelihood. Also, since the latent random variable of GAN is i.i.d. random variable, it is easy to calculate the log-likelihood of predicted latent code. These characteristics of dynamic latent scale GAN enable the log-likelihood of the predicted latent code to be used for out-of-distribution detection. The proposed method does not require a pre-trained model, mutual information of in-distribution data, and additional hyperparameters for prediction. The proposed method showed better out-of-distribution detection performance than the previous state-of-art method.
更多查看译文
关键词
dynamic latent scale gan,detection,self-supervised,out-of-distribution
AI 理解论文
溯源树
样例
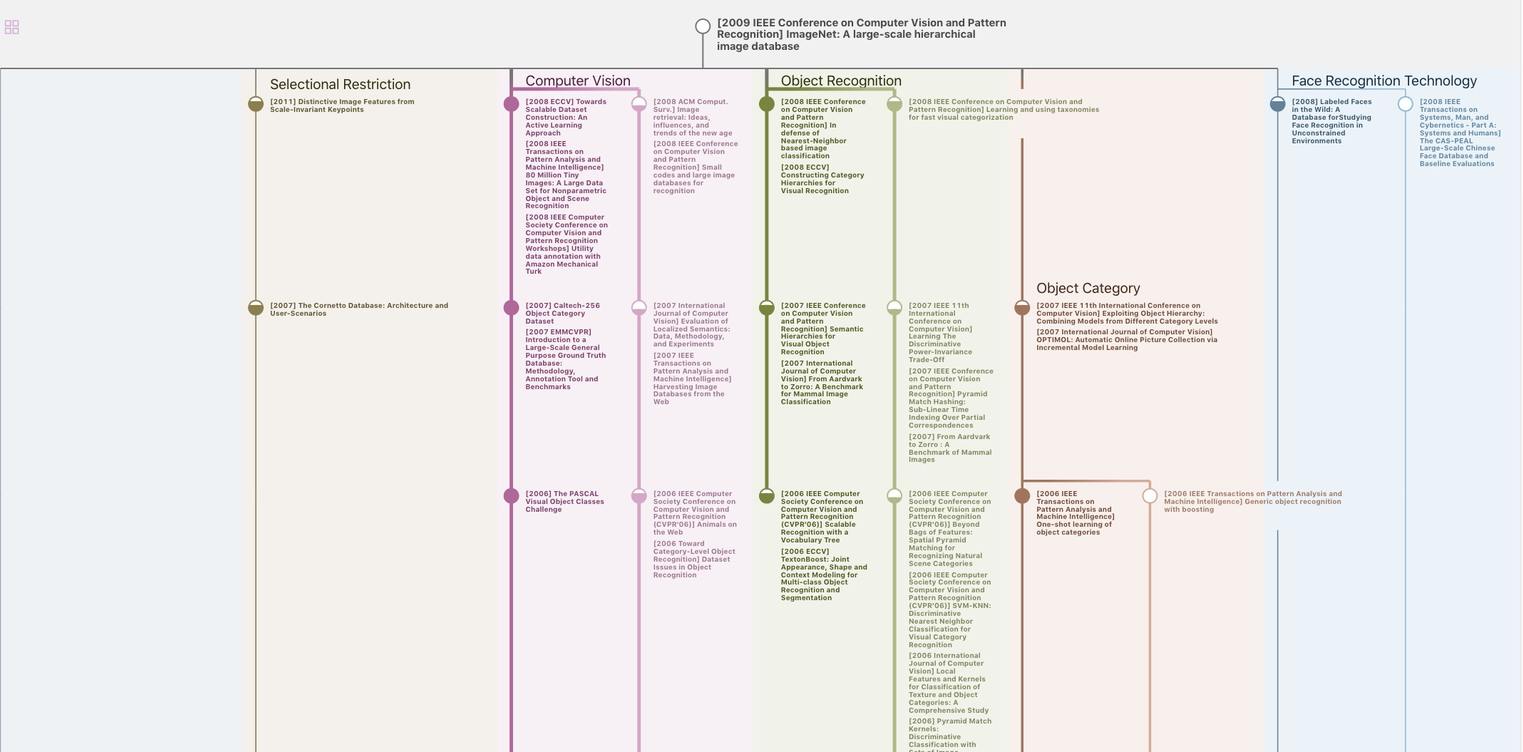
生成溯源树,研究论文发展脉络
Chat Paper
正在生成论文摘要