Joint Matrix Factorization and Structure Preserving for Domain Adaptation
CGI(2022)
摘要
Domain adaptation aims to learn robust classifiers for the target domain by transferring knowledge from the labeled source domain. However, most of the existing studies emphasize learning domain-invariant feature representations by employing distribution alignment on the feature space, which ignores the influence of data noise and structure knowledge. To address these issues, we propose a new domain adaptation approach, which can effectively reduce the impact of data noise and simultaneously thoroughly exploit the manifold data structures to transfer discriminative knowledge. Specifically, we jointly model matrix factorization, maximum entropy, distribution alignment in a unified framework, which can effectively alleviate the negative transfer of the outliers. Furthermore, we devise graph dual regularization to thoroughly explore the intrinsic manifold data structures, which can significantly reduce structure discrepancy across domains. Experimental results on various domain adaptation tasks demonstrate the superiority of the proposed method.
更多查看译文
关键词
Domain adaptation,Matrix factorization,Maximum entropy,Graph dual regularization
AI 理解论文
溯源树
样例
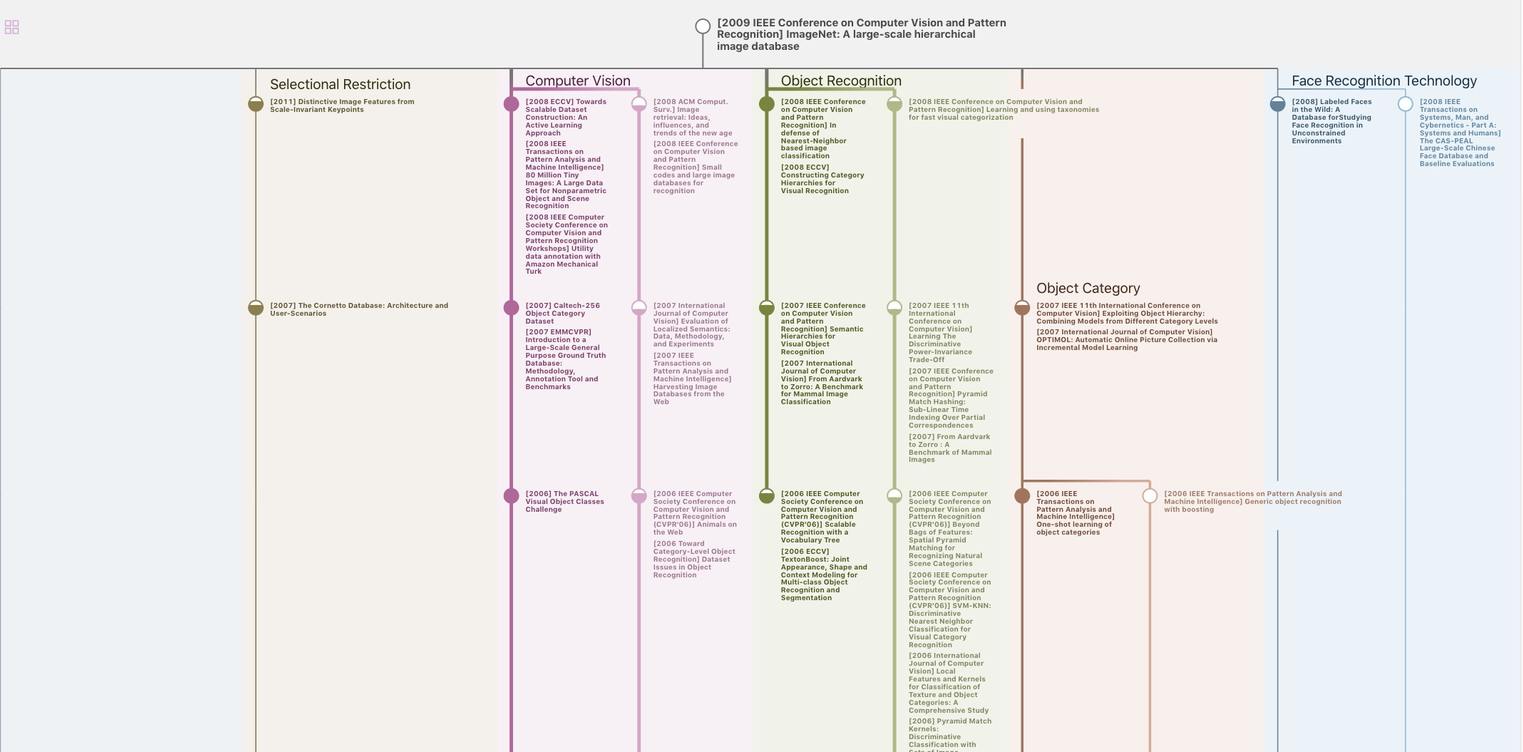
生成溯源树,研究论文发展脉络
Chat Paper
正在生成论文摘要