Topology-Aware Learning for Semi-supervised Cross-domain Retinal Artery/Vein Classification.
CGI(2022)
摘要
Pixel-level Artery/Vein (A/V) classification of retinal blood vessels is important in diagnosing and understanding a wide spectrum of diseases. Deep learning-based methods have achieved promising results, but manual image annotation is a labor-intensive and time-consuming process and annotations between different datasets cannot be fully utilized. The model performance might be significantly decreased when training on one dataset but testing on another. Some unsupervised domain adaptation (UDA) methods can alleviate this problem. However, annotating a few target samples is usually very manageable and worthwhile especially if it improves the adaptation performance substantially. Consequently, we propose a novel semi-supervised retinal A/V classification method where a few labeled target samples and the labeled publicly-accessible source dataset are available. We first employ the teacher-student framework to achieve semi-supervised learning. Then, a new regional mixing method is proposed to reduce the domain gap at the region level, which takes into account the topological features of retinal vasculature. Furthermore, we combine spatial transformation with regional mixing to generate additional synthetic images, thus improving the generalization ability of the model. We evaluate the proposed method on a publicly accessible (DRIVE-AV) dataset and a private dataset, and the results show that the proposed method achieves state-of-the-art performance for A/V classification.
更多查看译文
关键词
artery/vein classification,topology-aware,semi-supervised,cross-domain
AI 理解论文
溯源树
样例
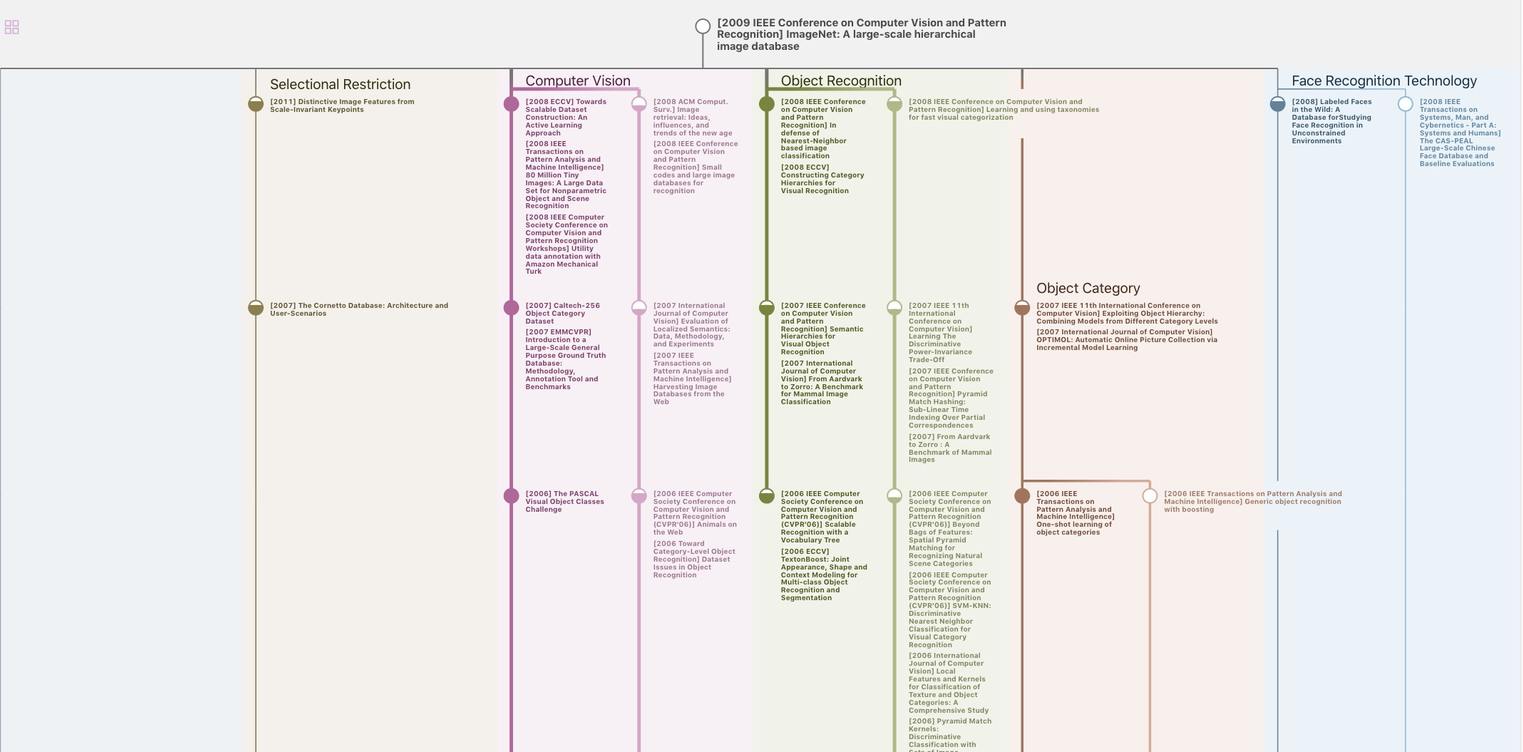
生成溯源树,研究论文发展脉络
Chat Paper
正在生成论文摘要