Curriculum Contrastive Learning for COVID-19 FAQ Retrieval.
BIBM(2022)
摘要
Medical Frequently Asked Question (FAQ) retrieval aims to find the most relevant question-answer pairs for a given user query, which is of great significance for enhancing people medical health awareness and knowledge. However, due to medical data privacy and labor-intensive labeling, there is a lack of large-scale question-matching training datasets. Previous methods directly use the collected question-answer pairs on search engines to train retrieval models, which achieved poor performance. Inspired by recent advances in contrastive learning, we propose a novel contrastive curriculum learning framework for modeling user medical queries. First, we design different data augmentation methods to generate positive samples and different types of negative samples. Second, we propose a curriculum learning strategy that associates difficulty levels with negative samples. Through a contrastive learning process from easy to hard, our method achieves excellent results on two medical datasets.
更多查看译文
关键词
Information retrieval,FAQ system,Question Generation,Contrastive Learning,Curriculum Learning
AI 理解论文
溯源树
样例
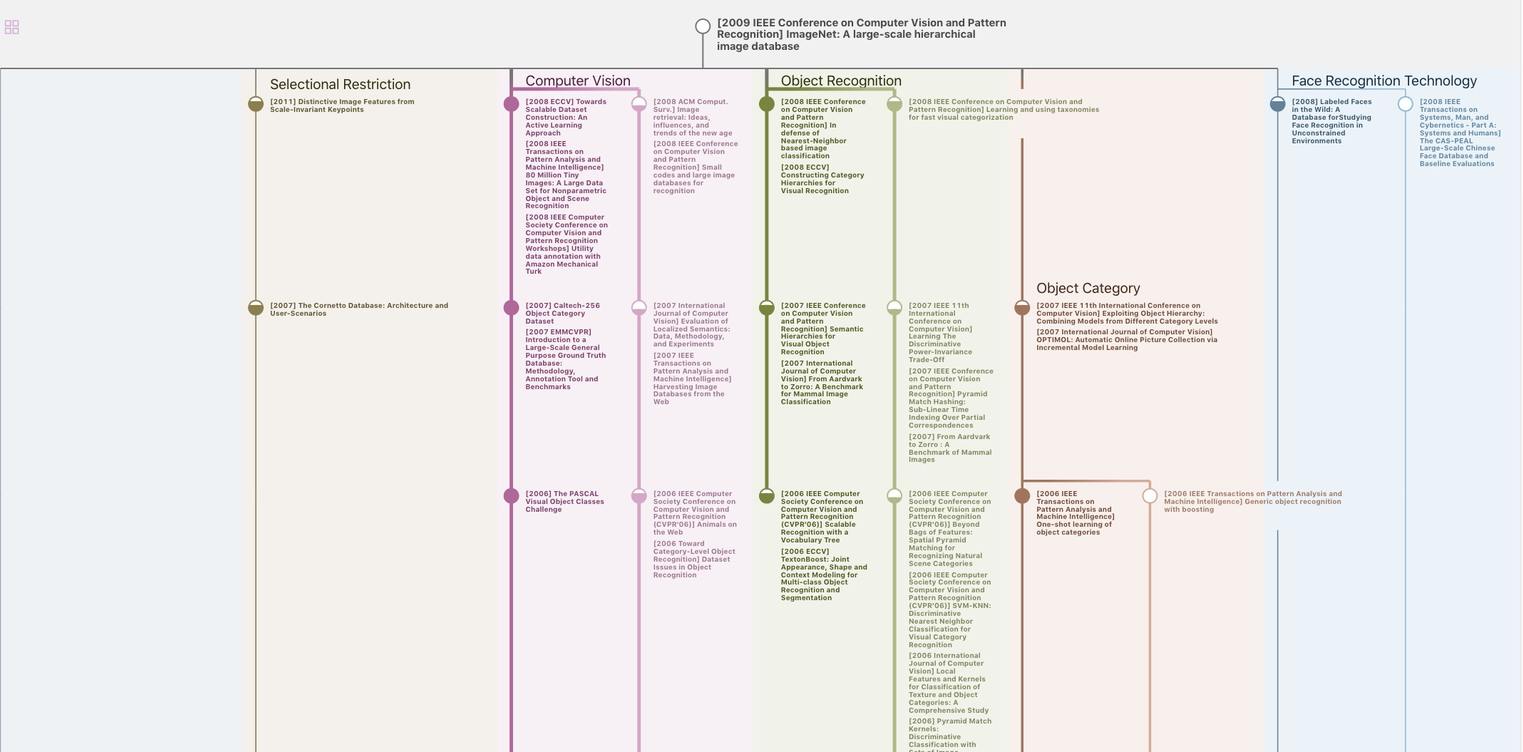
生成溯源树,研究论文发展脉络
Chat Paper
正在生成论文摘要