Phenotype Prediction by Heterogeneous Molecular Network Embedding.
BIBM(2022)
摘要
Phenotype prediction aims to infer the traits of living organisms based on genomics data, which has important applications in biology such as cancer subtype diagnosis and crop breedings. Traditional phenotype prediction approaches only learn the low-dimensional representation of samples, but ignore the interaction between biomolecules and cannot use the topology structure of heterogeneous molecular networks. Furthermore, most of them lack interpretability and do not effectively identify key biomolecules associated with phenotypes. In this paper, we propose a heterogeneous network embedding based solution (PhenoHNE) to predict phenotype by fusing topology information of molecules. PhenoHNE firstly utilizes variational graph autoencode (VGAE) to obtain the embedding representation of molecules in the heterogeneous network. Secondly, PhenoHNE adopts multilayer perceptron (MLP) with attention mechanism to learn sample representation. Finally, it fuses molecular embedding representation and sample representation to predict the phenotype of samples. In this way, the genetics information of heterogeneous molecular network can further guide the feature learning of samples and improve the prediction performance. Experimental results on human and maize datasets confirm that PhenoHNE outperforms competitive methods by a large margin under different evaluation protocols, and it also can effectively identify the key molecules associated with phenotypes of interests.
更多查看译文
关键词
Phenotype Prediction,Heterogeneous Molecular Network,Multi-Omics Data Fusion,Variational Graph AutoEncode,Multilayer Perceptron
AI 理解论文
溯源树
样例
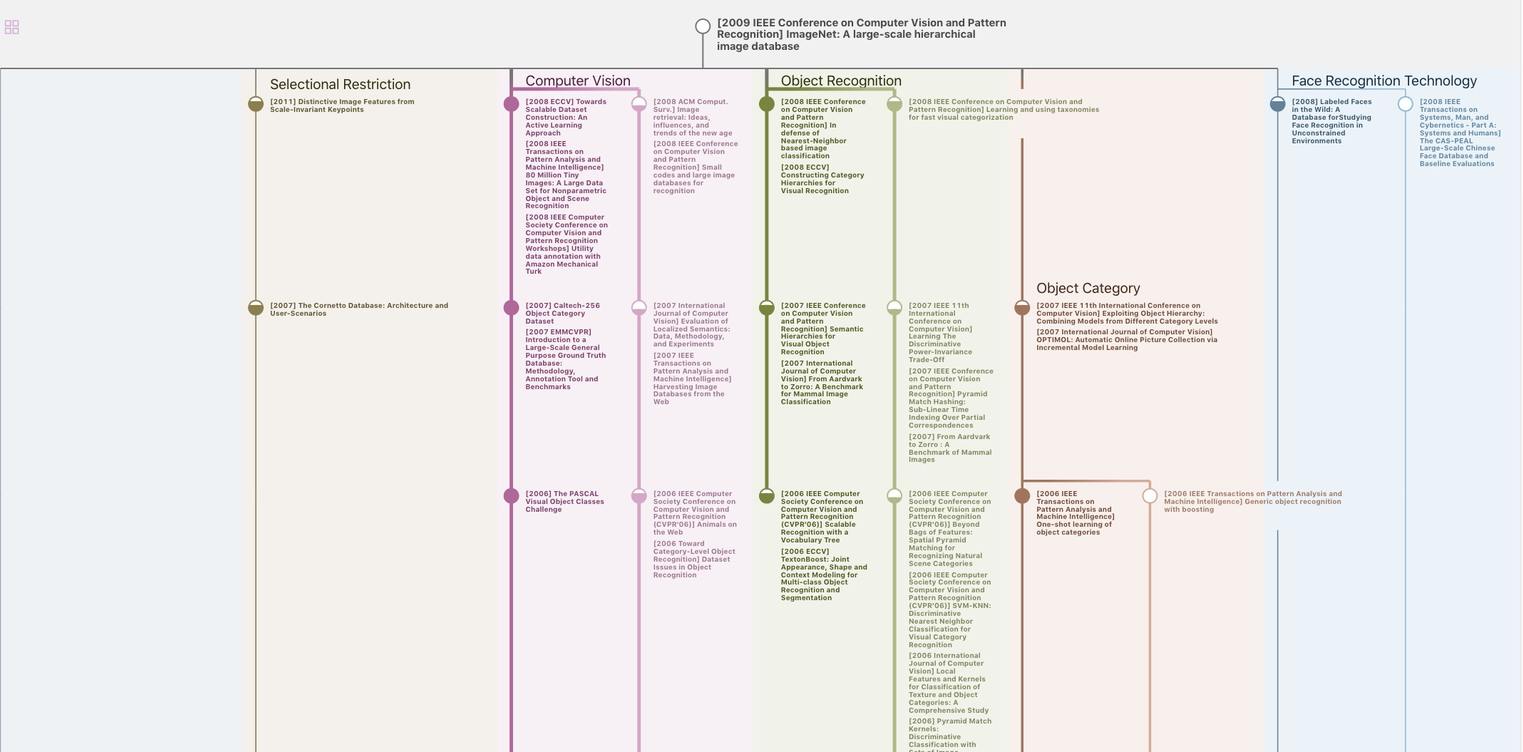
生成溯源树,研究论文发展脉络
Chat Paper
正在生成论文摘要