Weakly Unpaired Image Translation from Hematoxylin and Eosin Staining Image to Immunohistochemistry Staining Image.
BIBM(2022)
摘要
Histopathological imaging is one of the most critical disease diagnosis tools. Hematoxylin and eosin (H&E) staining images are routinely acquired and show all cells in the tissue. In contrast, immunohistochemistry (IHC) staining of cell type markers reveals spatial information of specific cell types of interest, and their use is limited by more complex laboratory processing and high cost. As an example, CD3 IHC images provide critical spatial information on the tumor-infiltrating lymphocytes (TILs), but for most tumor specimens, only H&E images are available. It is of high interest to predict the localization of CD 3 + cells based on H&E images without performing CD3 IHC, which may be achieved through image translation. Deep learning methods have been developed to perform image translation from exactly matched image pairs, but it is hard to acquire matched pairs of H&E and CD3 IHC images because they are typically from close but different slices of one tissue. In this paper, we propose a Registration Generative Adversarial Network (R-GAN) to translate H&E images into CD3 IHC images using weakly unpaired training samples. A registration module and a novel patch-level L
1
loss function are incorporated into Pix2PixGAN to address the challenges arising from weakly unpaired training samples. We conduct benchmarking experiments on a dataset constructed with tissue microarrays (TMAs) of liver cancer tissues, which contains H&E images and the corresponding CD3 IHC images for 1,073 tissue cores. The proposed R-GAN outperforms two commonly used translation methods in terms of both image quality and style relevance metrics.
更多查看译文
关键词
H&E image,CD3 IHC image,image translation,deep learning,weakly supervised learning
AI 理解论文
溯源树
样例
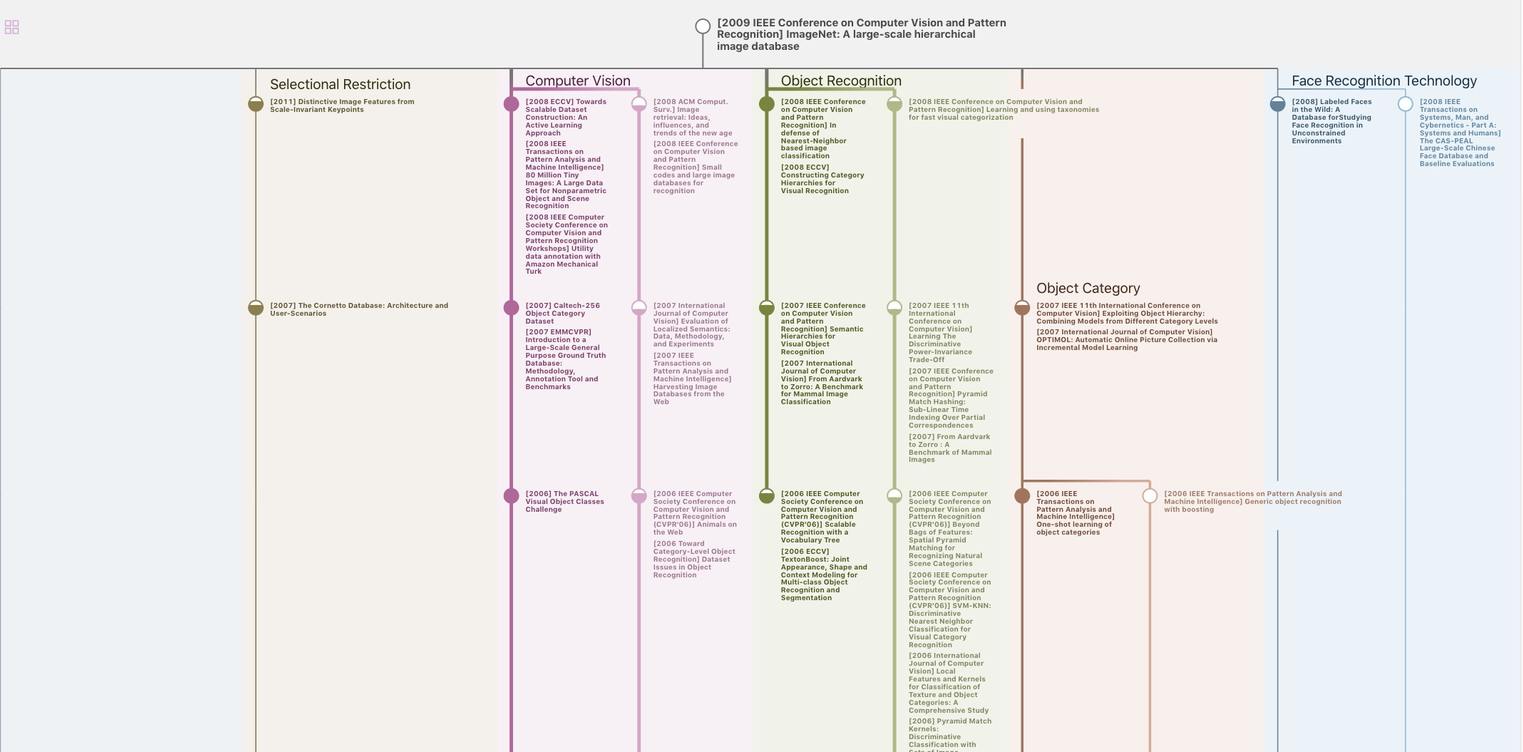
生成溯源树,研究论文发展脉络
Chat Paper
正在生成论文摘要