Multi-Relational Cognitive Diagnosis for Intelligent Education.
CICAI (2)(2022)
摘要
In intelligent education, cognitive diagnosis is a fundamental but important task, which aims to discover students' mastery of different knowledge concepts. Plenty of methods have been proposed to exploit student-exercise interactions, especially graph-based methods. However, most of them treat student behaviors to exercises as a binary interaction (i.e., interacted or not), neglecting diverse behavior patterns (i.e., correct and incorrect interactions). Moreover, the number of concepts is much smaller than exercises, presenting a challenge for measuring student proficiency. Therefore, in this paper, we propose a novel Multi-Relational Cognitive Diagnosis (MRCD) framework. Specifically, we first divide students' answer behaviors into correct and incorrect interactions with exercises, and form the corresponding two student-exercise relation graphs. We then leverage Graph Convolutional Network to learn exercise-level representations of students and exercises based on different relation graphs. Since dividing operation exacerbate the data sparsity problem, we employ graph contrastive learning to enhance MRCD on representation learning. Moreover, considering the relatively small number of concepts, we directly employ attention mechanism to generate student and exercise representations based on relevant concepts. After that, we fuse exercise-level and concept-level representations, and send them to a cognitive diagnosis model to predict student performance. Extensive experiments over two real-world datasets demonstrate the effectiveness of our proposed model.
更多查看译文
关键词
cognitive,diagnosis,education,multi-relational
AI 理解论文
溯源树
样例
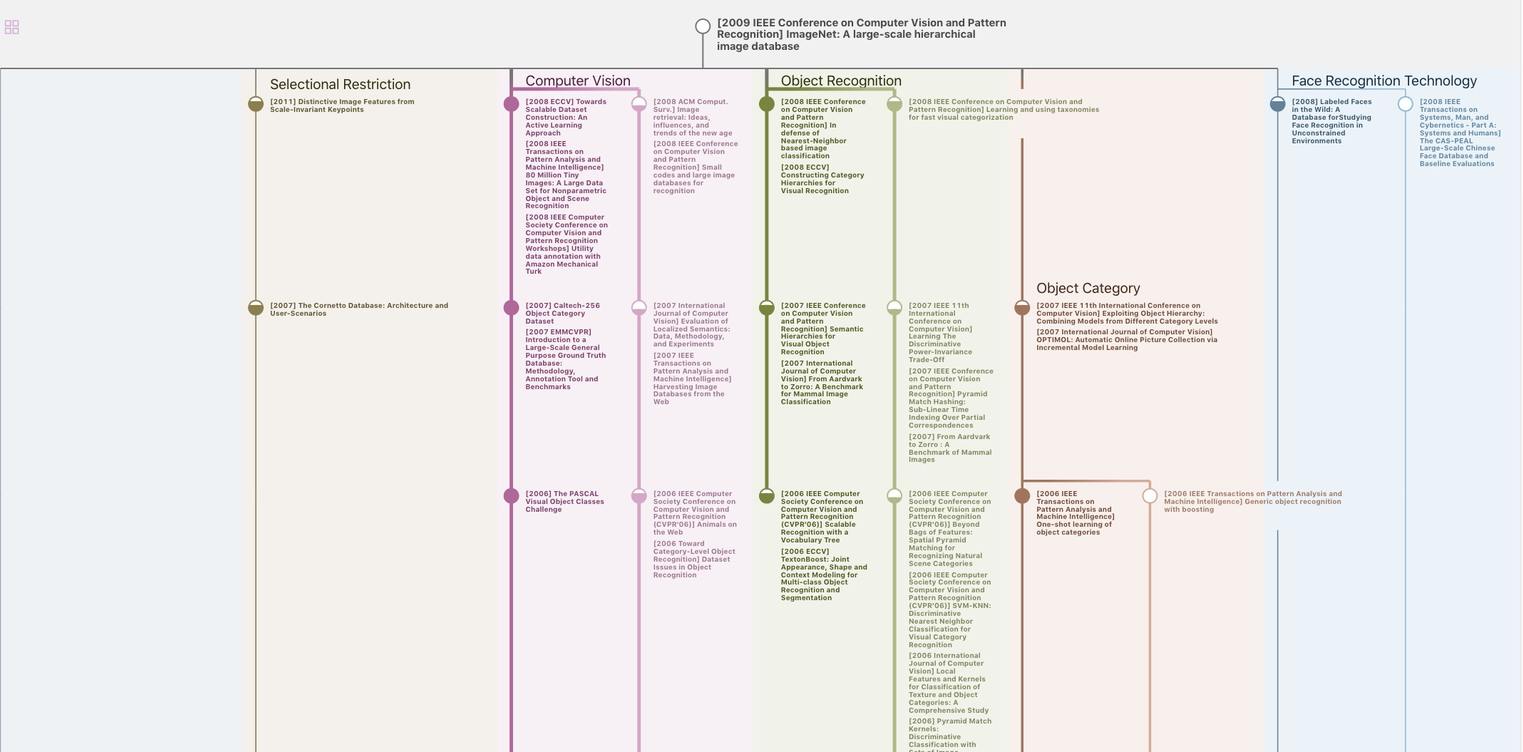
生成溯源树,研究论文发展脉络
Chat Paper
正在生成论文摘要