Aero-Engine Remaining Useful Life Prediction via Tensor Decomposition Method.
CICAI (2)(2022)
摘要
Aiming at the problems of low accuracy and long calculation time of remaining useful life (RUL) prediction of aero-engine by the data-driven method, a method for mining aero-engine information in tensor mode is proposed in this paper. Different from the traditional vector mode of data feature extraction, the tensor form of data can reflect the structure and correlation information among data. It has certain advantages when dealingwith data that has strong coupling such as aero-engine signals. A large turbofan engine degradation simulation dataset (C-MAPSS dataset) with strong coupling provided by NASA is used to validate the method proposed in this paper. The results show that the data extracted by tensor decomposition method has better training effect than the unprocessed data in convolutional neural network (CNN), with the average prediction accuracy improved by 28.39%, and the average learning time shortened by 24.25%.
更多查看译文
关键词
Aero-engine remaining useful life prediction, Tensor mode, Tensor decomposition, Convolutional neural network
AI 理解论文
溯源树
样例
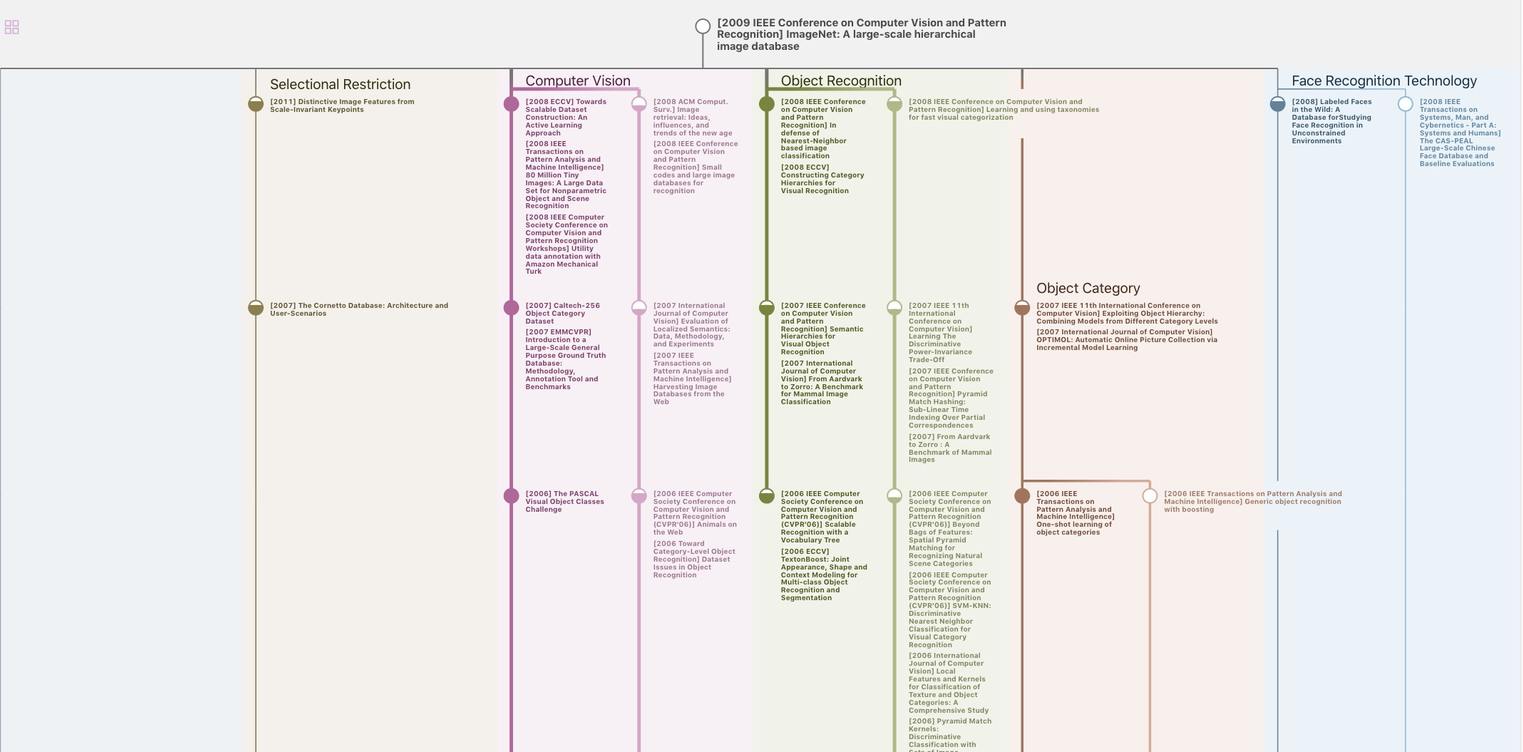
生成溯源树,研究论文发展脉络
Chat Paper
正在生成论文摘要