Visual Perception Inference on Raven's Progressive Matrices by Semi-supervised Contrastive Learning
CICAI (2)(2022)
摘要
The current deep neural networks (DNNs) in mimicking human perception remain challenges for solving visual reasoning tasks. Human perception does not merely involve a passive observer labeling sensory signals, but also contains an active inference about object attributes and their relationships towards an intended output (e.g., an action). In this work, we propose a variational autoencoder (VAE) model to discriminate the ranking relationships between object attribute values by semi-supervised contrastive learning, dubbed as SSCL-VAE. This perception-based model solves the visual reasoning task of Raven's Progressive Matrices (RPM) in three benchmarks (RAVEN, I-RAVEN and RAVEN-Fair), with high accuracy close to humans, as well as many end-to-end supervised models. The current work thus suggests that constructions of general cognitive abilities like human perception may empower the perceptron with DNN to solve high-level cognitive tasks such as abstract visual reasoning in a human-like manner.
更多查看译文
关键词
Perception,Inference,Contrastive learning,Semi-supervised,Autoencoder
AI 理解论文
溯源树
样例
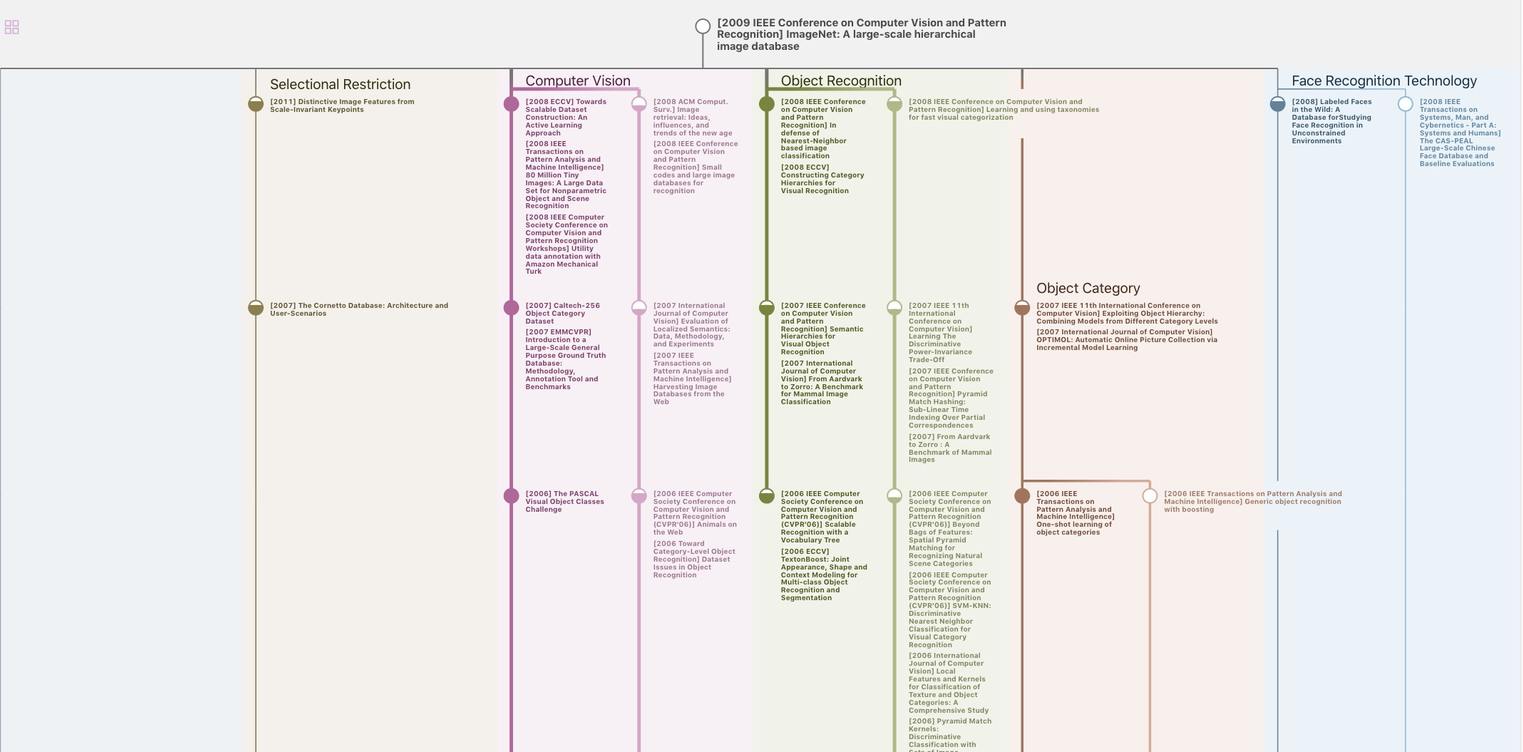
生成溯源树,研究论文发展脉络
Chat Paper
正在生成论文摘要