Intent-aware Prompt Learning for Medical Question Summarization.
2022 IEEE International Conference on Bioinformatics and Biomedicine (BIBM)(2022)
摘要
Consumer health question summarization aims to generate concise questions expressing the minimum information needed to find the correct answer to the original user queries, which can significantly improve the success rate of retrieving relevant answers in automated medical question answering systems. Recently, prompt learning has emerged as a new paradigm of leveraging pre-trained language models (PLMs) with promising results in downstream tasks. Existing prompt-based methods assume that all samples in a task share the same prompt. However, the health questions raised by consumers in search engines focus on different types of medical entities and cover different purposes. It is not sufficient to simply use the same prompt regardless of consumer intent awareness, which likely leads to meaningless or substandard quality summaries. In this paper, we propose an intent-aware prompt learning (InPL) method, which consists of a question intent recognition module, a dynamic prompt module and a medical summarization generation module to model the medical summarization task in a unified transformer. Our InPL architecture generates high-quality summaries capturing more consumer health intent and encourages machines to generate more factually correct summaries. Extensive experiments on the popular medical summarization datasets demonstrate the superiority of our approach.
更多查看译文
关键词
Consumer health question,Text summarization,Pre-training language model,Prompt learning
AI 理解论文
溯源树
样例
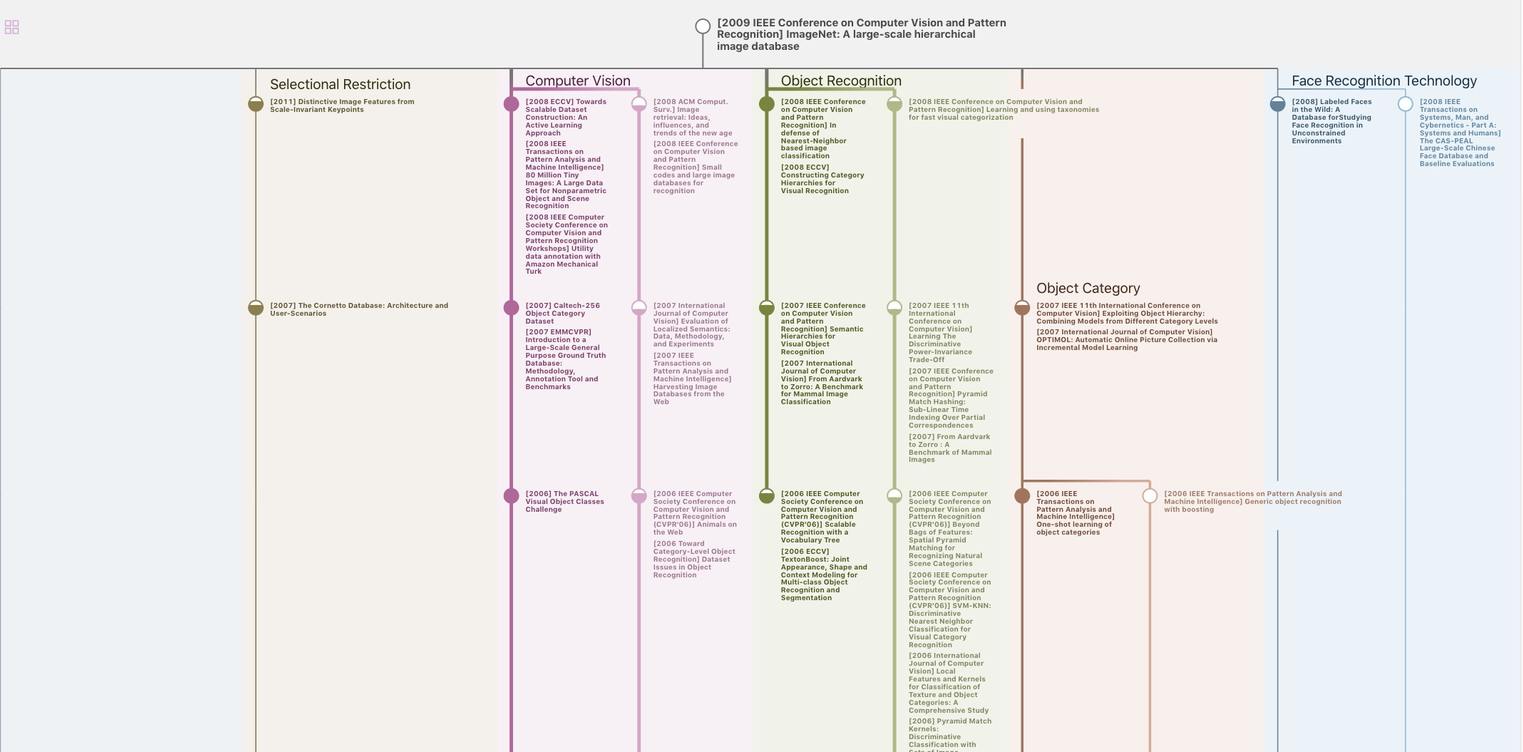
生成溯源树,研究论文发展脉络
Chat Paper
正在生成论文摘要