Deep learning-based restaining of histopathological images.
BIBM(2022)
摘要
Pathology entails the visual examination of digital slides created by various staining techniques based on the chemical properties of tissue histopathology. Hematoxylin and eosin stain reveals the morphological features of tissue structure. However, in modern histopathology, immunohistochemistry provides additional information on different tissue components through the antibody-mediated visualization of specific proteins. For this, the tissue sample must be stained with multiple dyes to provide the extra information that specialists need for diagnosis. A significant l imitation of this process–restaining–is that it requires a long time in the conventional laboratory flow. Deep learning-based virtual staining techniques are a considerable alternative for rapid diagnosis and treatment by minimizing time expenditure. In this study, we present a deep neural network-based restaining method, which we call StainGAN, to replace the time-consuming and expensive traditional laboratory flow. StainGAN builds upon the self-attention GAN, supplemented by contrastive learning that provides improved segmentation performance as well as a color-based loss function for extracting recessive T-cell signals in the immunohistochemistry stained image. We demonstrate the quantitative performance of StainGAN against state-of-the-art methods, such as CycleGAN and Pix2Pix as well as its qualitative performance using the multiscale structural similarity index measure (MSSIM) and universal image quality index (UQI) metrics. Although StainGAN produced much higher qualitative and quantitative results than the Pix2Pix approach, it showed only slightly higher performance than the CycleGAN approach. According to the visual evaluation by pathologists, virtual CD8-stained images produced by the proposed model show a high similarity to real CD8-stained images. Moreover, numerical similarities were quantified by comparing the presence of immune cells in virtual CD8 WSIs to real CD8 WSIs.
更多查看译文
关键词
contrastive learning,general adversarial network,stain translation,immunohistochemistry,whole slide images
AI 理解论文
溯源树
样例
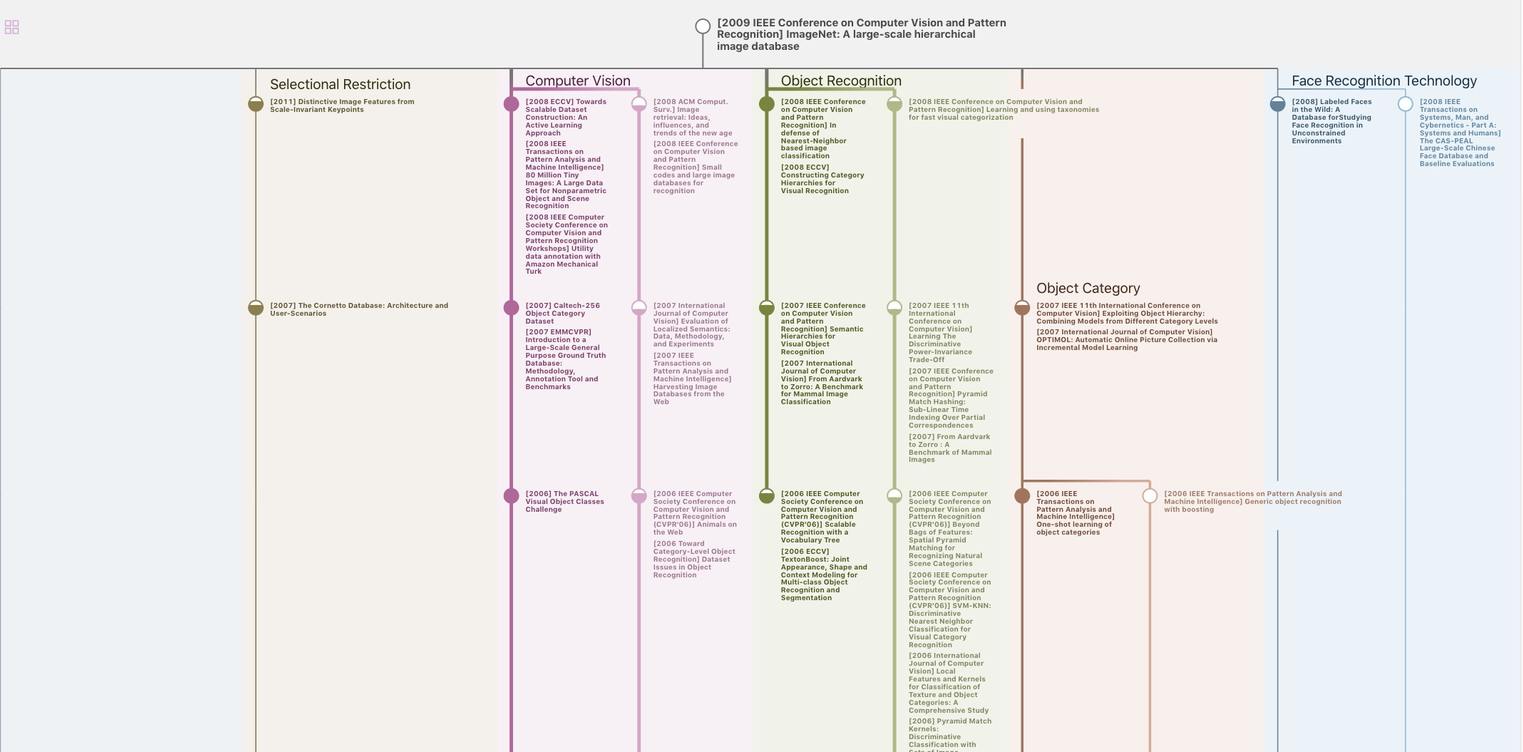
生成溯源树,研究论文发展脉络
Chat Paper
正在生成论文摘要