Multi-task Learning with Consistent Prediction for Efficient Breast Ultrasound Tumor Detection.
BIBM(2022)
摘要
Segmentation and classification a re h ighly correlated tasks in tumor detection from breast ultrasound images. Recent studies have successfully applied multi-task learning to breast ultrasound image analysis to explore the correlation between tasks. However, there exists potential inconsistency between individual tasks that critically affect the overall performance of breast ultrasound image analysis. Therefore, this study designs a consistency branch for harmonizing the segmentation and classification t ask 0 ptimization. T he c onsistency b ranch characterizes the outputs of individual task-specific models to maintain consistency during training, thereby generating highly consistent results. Specifically, the consistency branch outputs a consistency probability while determining the inconsistency types predicted by both tasks. Subsequently, the segmentation and classification loss weights are reconciled using consistency probabilities based on the inconsistent prediction behavior for each sample, thus constraining the two tasks to produce consistent predictions close to the ground truth. The evaluation using private and public breast ultrasound image datasets indicates that the proposed method can effectively remedy the inconsistent predictions between tasks for improved computerized breast ultrasound image analysis.
更多查看译文
关键词
breast ultrasound,tumor detection,segmentation and classification,multi-task learning,consistent predictions
AI 理解论文
溯源树
样例
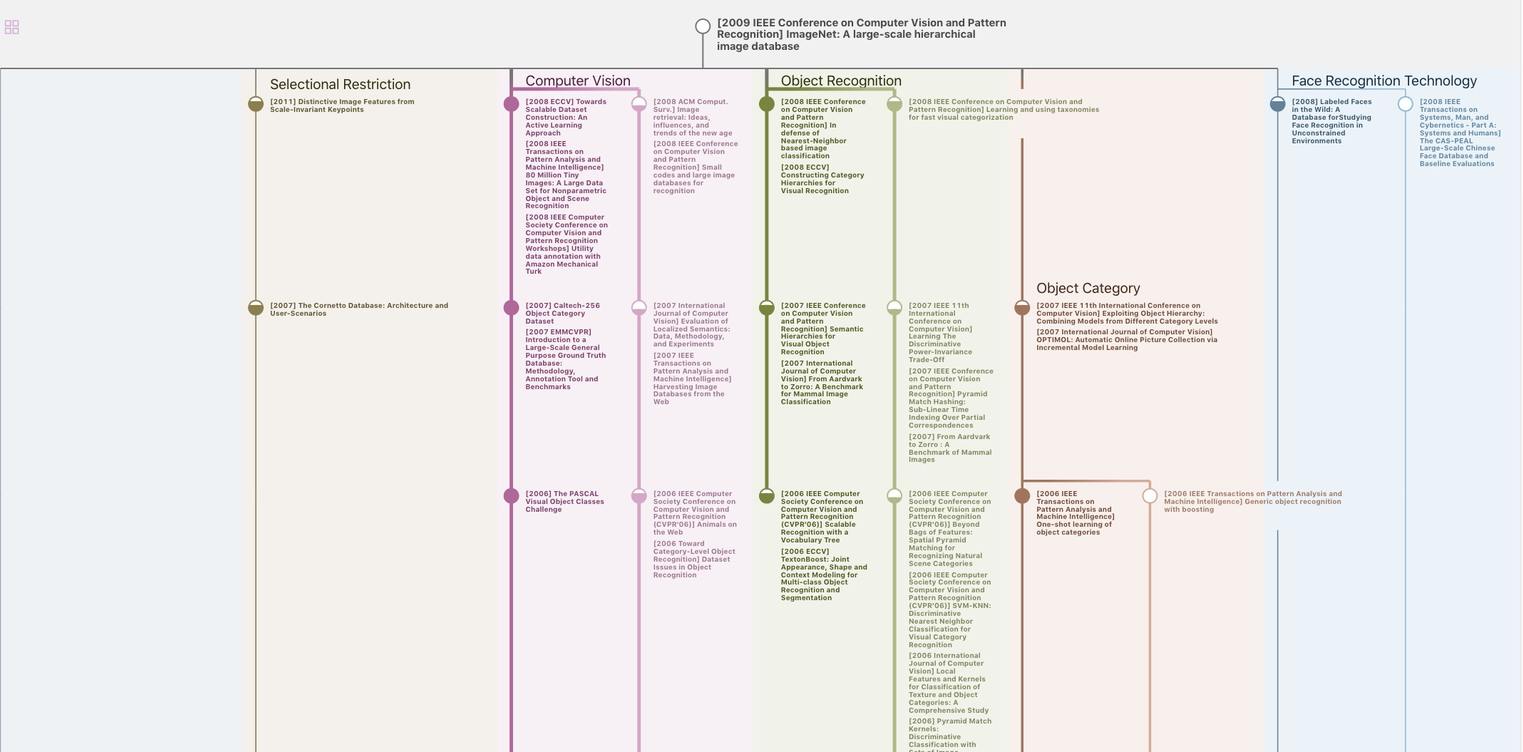
生成溯源树,研究论文发展脉络
Chat Paper
正在生成论文摘要