Automatic Removal of Scalp EEG Artifacts Using an Interpretable Hybrid Deep Learning Method.
BIBM(2022)
Abstract
The application of scalp electroencephalogram (EEG) in brain-computer interface (BCI) is inevitably hindered by the contamination of various physiological artifacts. Artifact elimination plays an important role in EEG signal analysis due to its weak power and low signal-to-noise ratio. Recent studies using deep learning (DL) methods have reported good performance for offline artifact elimination. However, existing methods still neglect two key defects in clinical applications: (1) offline processing may not fit inreal-time BCI application scenarios; and (2) direct and arbitrary usage of conventional neural network structures lacks physiological interpretability. To address these problems, we propose a hybrid method for EEG artifact elimination, combining a canonical correlation analysis (CCA) module–a blind source separation method with a multi-scaled convolutional neural network (CNN) module. Specifically, the CCA module transfers the recorded EEG signals simultaneously from the channel space to the source space and the CNN module detects and eliminates artifacts with explainable processing. Extensive experiments on semi-simulation, resting-state, and motor imagery EEG datasets were conducted and the results showed competitive performance and strong adaptability of the proposed method.
MoreTranslated text
Key words
EEG,artifact elimination,canonical correlation analysis,convolutional neural network,brain-computer interface
AI Read Science
Must-Reading Tree
Example
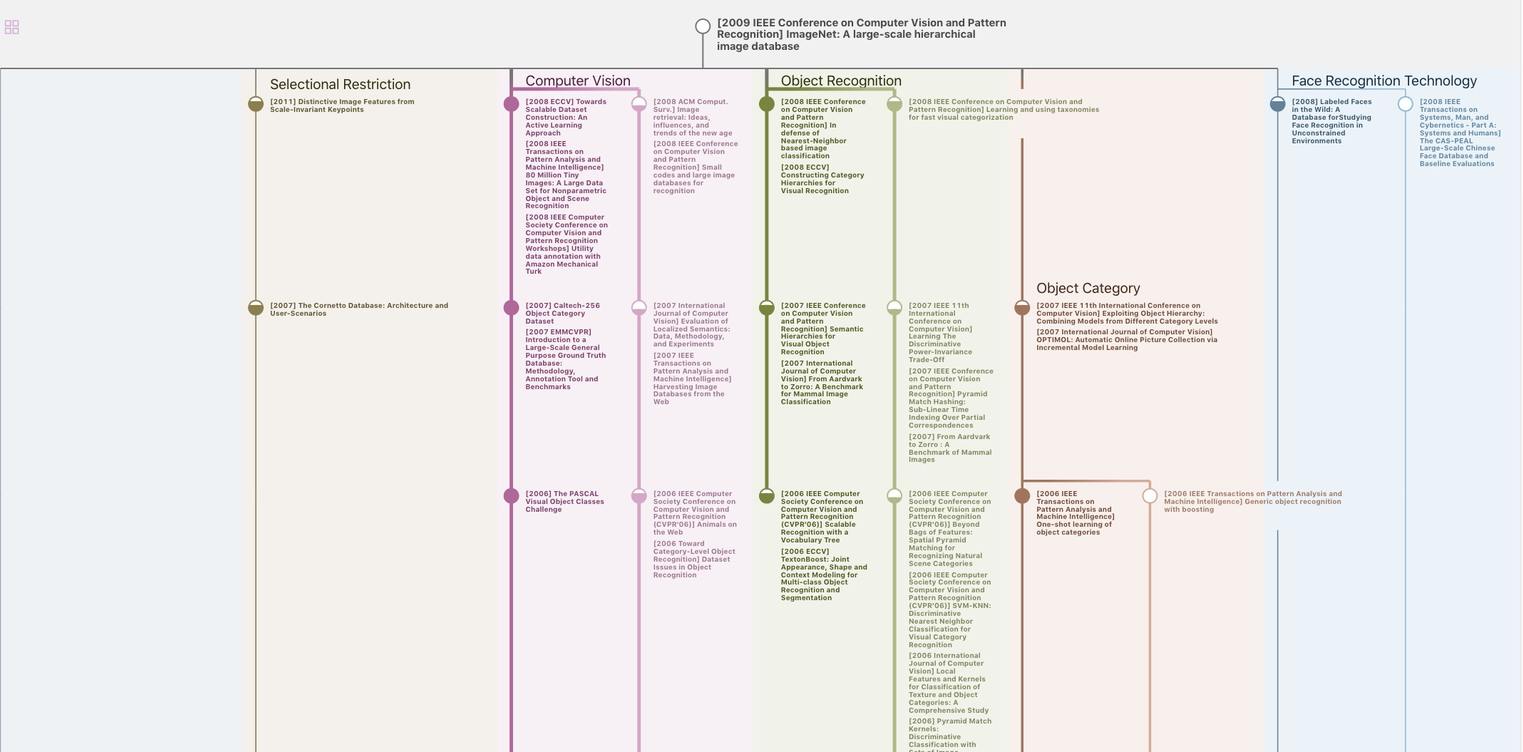
Generate MRT to find the research sequence of this paper
Chat Paper
Summary is being generated by the instructions you defined