Wavelet-based Spectral Analysis For Protein Conformation Selection and Prediction Using AI in Drug Discovery Applications.
BIBM(2022)
摘要
Contemporary drug discovery relies heavily on massive high performance computing (HPC) resources from docking and molecular dynamics simulations of proteins interacting with drug candidate ligands, such as recently published form simulations on ORNL’s SUMMIT in covid19 pharmaceutical research. This work presents a unique spectral analysis approach using wavelet transform (WT) to understand the correlation between the time evolution of protein conformations generated by molecular dynamics and specific protein conformations that are selected for binding by ligands. A DWT-based spectral analysis is performed on the unique protein descriptors previously identified to be important in protein: ligand binding. The new protein time-series information from the wavelet-based time-frequency domain analysis is used for a more refined protein conformation selection and improve the deep learning and machine learning (AI/ML) prediction framework to improve the prediction of binding vs. non-binding protein conformations for three target proteins ADORA2A, OPRD1 and OPRK1. In this work, wavelets are used for spectral analysis for their added benefit of simultaneous time-frequency resolution and denoising properties.
更多查看译文
关键词
protein conformation selection,spectral analysis,discovery,wavelet-based
AI 理解论文
溯源树
样例
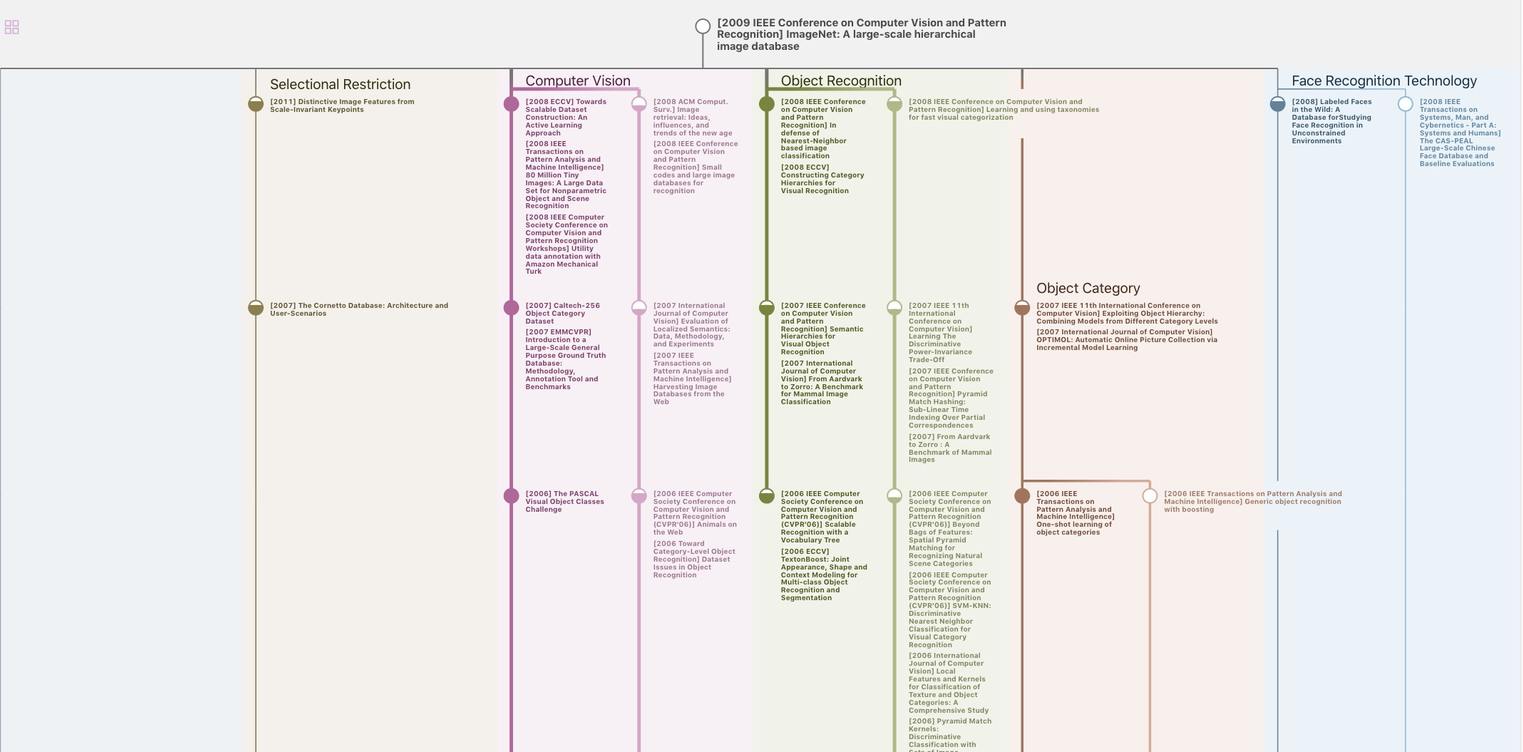
生成溯源树,研究论文发展脉络
Chat Paper
正在生成论文摘要