An Empirical Comparison of DistilBERT, Longformer and Logistic Regression for Predictive Coding.
Big Data(2022)
摘要
In recent years, transformer-based, large-scale language models have greatly advanced deep learning in NLP tasks. These models allow transfer learning to be performed on NLP in similar methods to what was previously done for computer vision. Two of the latest models are DistilBERT and Longformer -- the former is a distilled version of BERT, which enables faster training and inferencing on off-cloud servers, and the latter offers capability of working with long texts. In this paper, we study empirical comparisons of the effectiveness of the two deep learning methods along with a logistical regression method for text classification, using three real-world datasets from legal document reviews. The study shows that Longformer performs better (up to 10%) than or at par with the other two methods. Cross-dataset evaluation is leveraged as well to validate the performance of Longformer as a viable method when labeled data is not available.
更多查看译文
关键词
logistic regression,longformer,empirical comparison
AI 理解论文
溯源树
样例
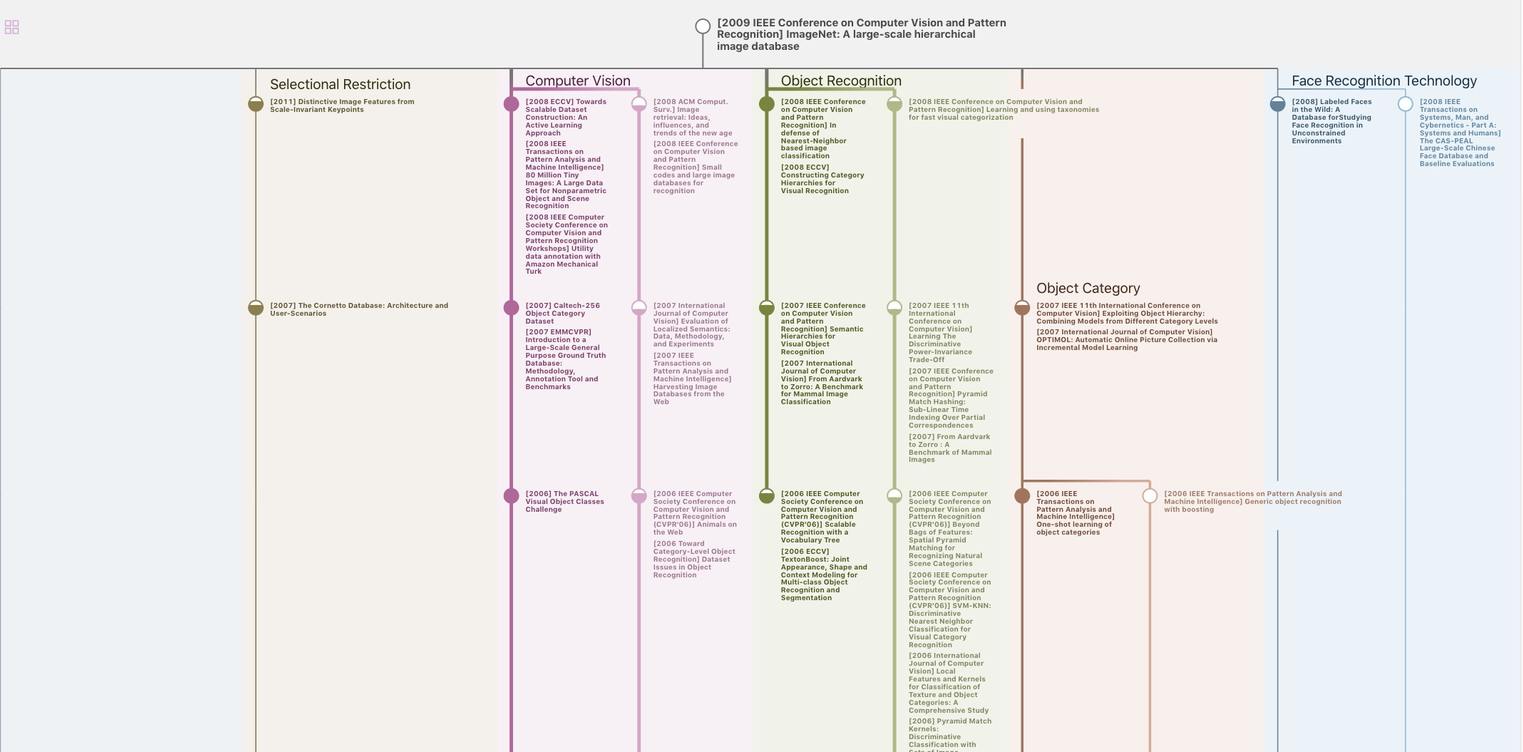
生成溯源树,研究论文发展脉络
Chat Paper
正在生成论文摘要