Multiple Tiered Treatments Optimization with Causal Inference on Response Distribution.
Big Data(2022)
摘要
For many business applications, event driven promotion programs are commonly used means for achieving business targets and performances such as customer experience and revenue etc. Traditional recommendation methods mainly focus on predicting the click-through-rate and can not handle this scenario well since most of driven promotion are multi-tiered programs. Moreover, promotion programs will incur costs, so we need to predict not only the effect of recommending programs to users, but also the cost of programs. So it is essentially an NP-hard optimization problem with a budget constraint. Recently, there are some studies that conducts causal inferences for analyzing the effects of various designed programs on users. However, causal inference models mostly address the expected effects of treatments instead of studying the probabilistic distribution of the heterogeneous effects on users, thus unable to help on accurate estimation of the program costs driven by events. In this paper, we argue that the expected treatment effects can be learned with the response distribution, and proposed a multi-task learning model for composite causal inference on both treatment effects and response distribution, which allows decision makers to make trade-off decisions on the return and cost of programs in an end-to-end manner and to optimize their policy and strategy.
更多查看译文
关键词
multiple tiered treatments optimization,causal inference,response distribution
AI 理解论文
溯源树
样例
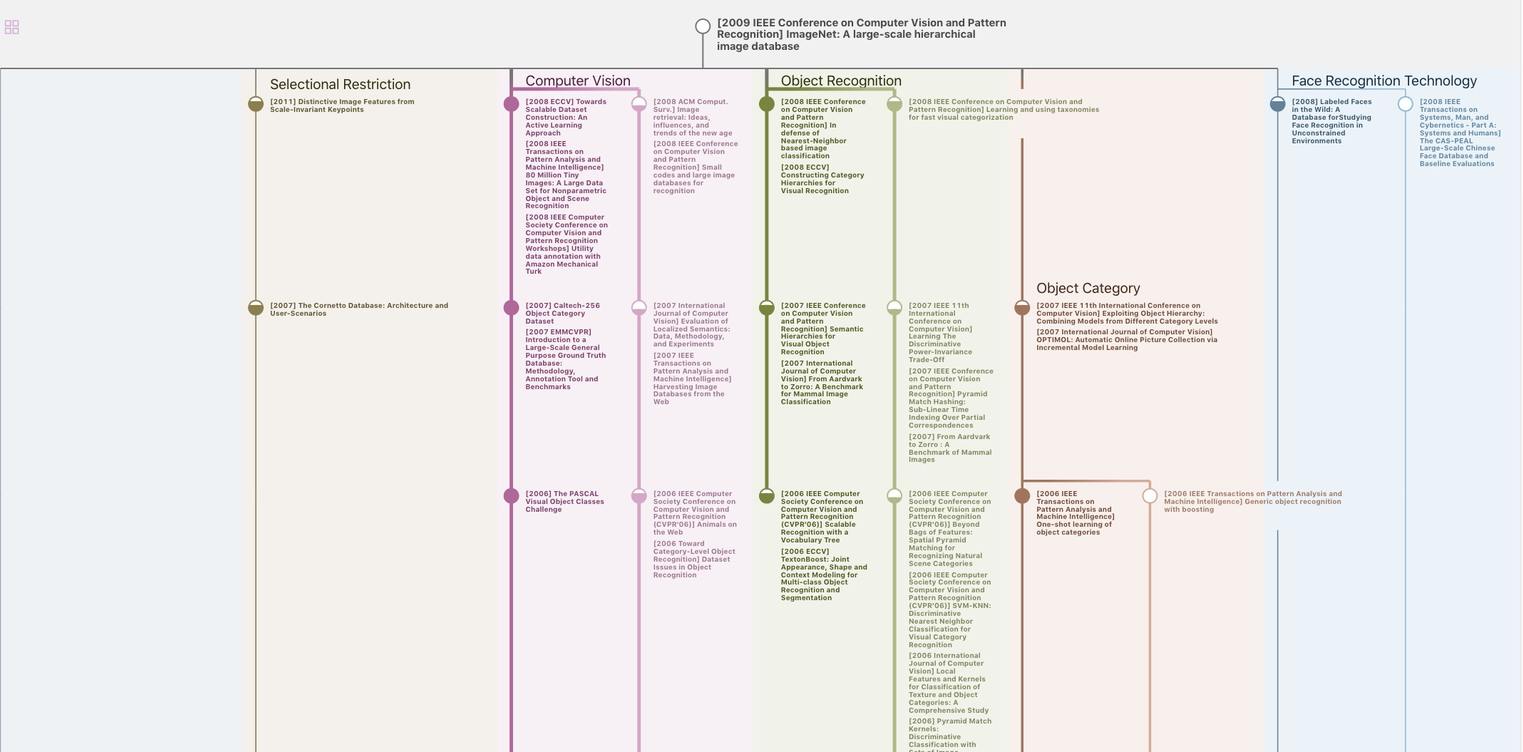
生成溯源树,研究论文发展脉络
Chat Paper
正在生成论文摘要