Secure Multi-party kNN Search in Large-scale Spatial Data Federation
Big Data(2022)
摘要
kNN is a fundamental query in various location based services such as POI recommendation and ride planning. There is an increasing demand to scale such services by querying over a data federation, where the entire dataset is distributedly held by multiple data providers (a.k.a., silos), and each silo keeps its data partition private. However, it is challenging to provide secure kNN queries over a large-scale data federation. Prior secure kNN queries can be are highly inefficient if performed cross silos because they involve excessive secure distance operations, which can be two or three orders of magnitude slower than the corresponding plaintext operations. In this work, we propose a novel threshold based framework for efficient kNN queries over a spatial data federation. The key idea is to rewrite excessive secure distance computations as light-weight secure operations. We further propose an adaptive threshold algorithm to reduce the secure communication rounds and accelerate the query processing. Extensive evaluations on both synthetic and real-world datasets show that compared with the state-of-the-art secure kNN querying methods, our solutions reduce the time cost by up to 104.1 times and communication cost by three orders of magnitude.
更多查看译文
关键词
k nearest neighbor,data federation,secure multi-party computation
AI 理解论文
溯源树
样例
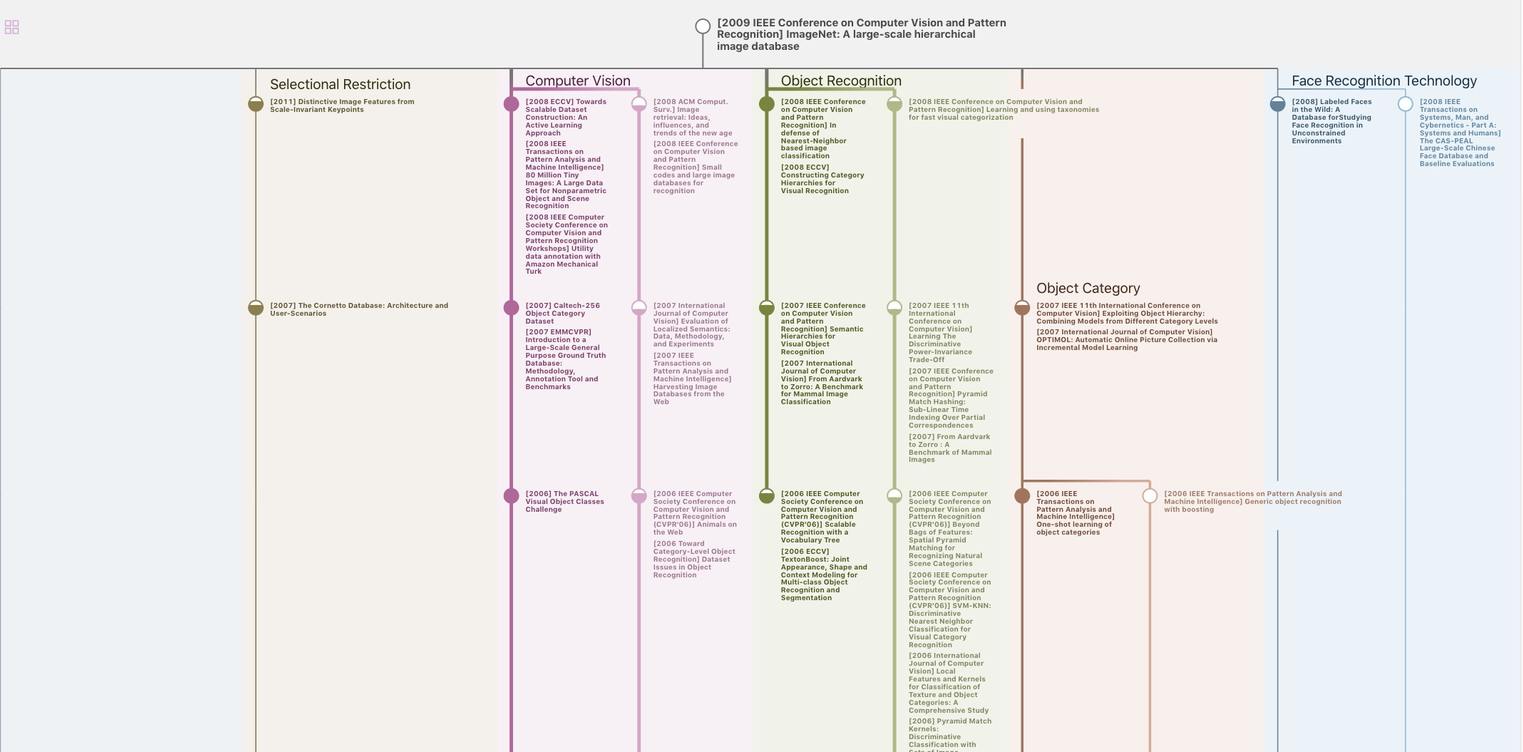
生成溯源树,研究论文发展脉络
Chat Paper
正在生成论文摘要