Exploring Pre-Trained Language Models to Build Knowledge Graph for Metal-Organic Frameworks (MOFs)
Big Data(2022)
摘要
Building a knowledge graph is a time-consuming and costly process which often applies complex natural language processing (NLP) methods for extracting knowledge graph triples from text corpora. Pre-trained large Language Models (PLM) have emerged as a crucial type of approach that provides readily available knowledge for a range of AI applications. However, it is unclear whether it is feasible to construct domain-specific knowledge graphs from PLMs. Motivated by the capacity of knowledge graphs to accelerate data-driven materials discovery, we explored a set of state-of-the-art pre-trained general-purpose and domain-specific language models to extract knowledge triples for metal-organic frameworks (MOFs). We created a knowledge graph benchmark with 7 relations for 1248 published MOF synonyms. Our experimental results showed that domain-specific PLMs consistently outperformed the general-purpose PLMs for predicting MOF related triples. The overall benchmarking results, however, show that using the present PLMs to create domain-specific knowledge graphs is still far from being practical, motivating the need to develop more capable and knowledgeable pre-trained language models for particular applications in materials science.
更多查看译文
关键词
language models,knowledge graph,mofs,pre-trained,metal-organic
AI 理解论文
溯源树
样例
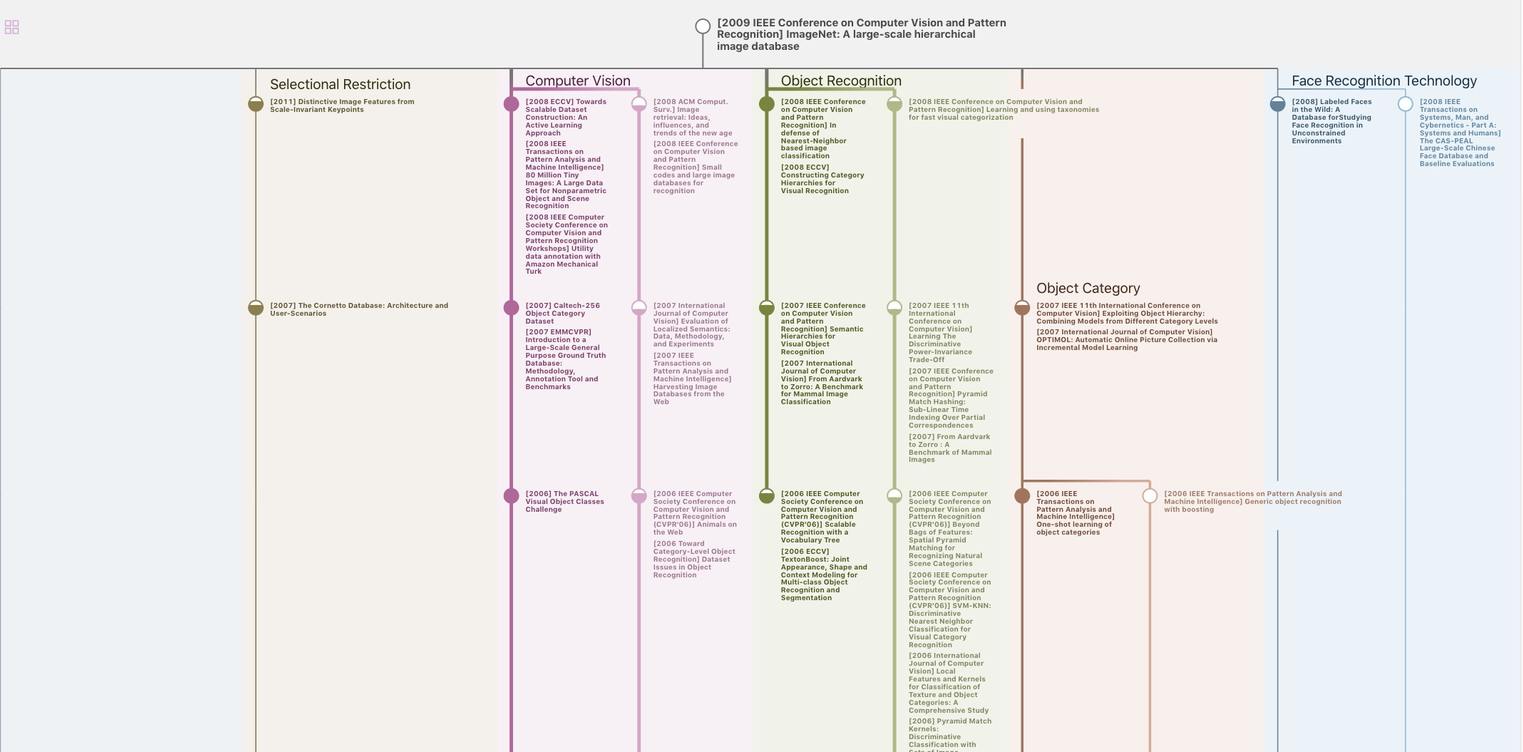
生成溯源树,研究论文发展脉络
Chat Paper
正在生成论文摘要