Robust Personalized Federated Learning under Demographic Fairness Heterogeneity.
Big Data(2022)
摘要
Personalized federated learning (PFL) gives each client in a federation the power to obtain a model tailored to their specific data distribution or task without the client forfeiting the benefits of training in a federated manner. However, the concept of demographic group fairness has not been widely studied in PFL. Further, fairness heterogeneity – when not all clients enforce the same local fairness metric – has not been studied at all. To fill this gap, we propose Fair Hypernetworks (FHN), a personalized federated learning architecture based on hypernetworks that is robust to statistical (e.g., non-IID and unbalanced data) and fairness heterogeneity. We theoretically show that granting clients the ability to independently choose multiple (possibly conflicting) fairness constraints, such as demographic parity or equalized odds, does not break previously proven generalization bounds on hypernetworks used in the federated setting. Additionally, we empirically test FHN against several baselines in multiple fair federated learning settings, and we find t hat F HN outperforms all other federated baselines when handling clients with heterogeneous fairness metrics. We further demonstrate the scalability of FHN to show that minimal degradation to the accuracy and the fairness of the clients occurs when the federation grows in size. Additionally, we empirically validate our theoretical analysis to show FHN generalizes well to new clients. To our knowledge, our FHN architecture is the first to consider tolerance to fairness heterogeneity which gives clients the freedom to personalize the fairness metric enforced during local training.
更多查看译文
关键词
fairness,hypernetworks,personalized federated learning
AI 理解论文
溯源树
样例
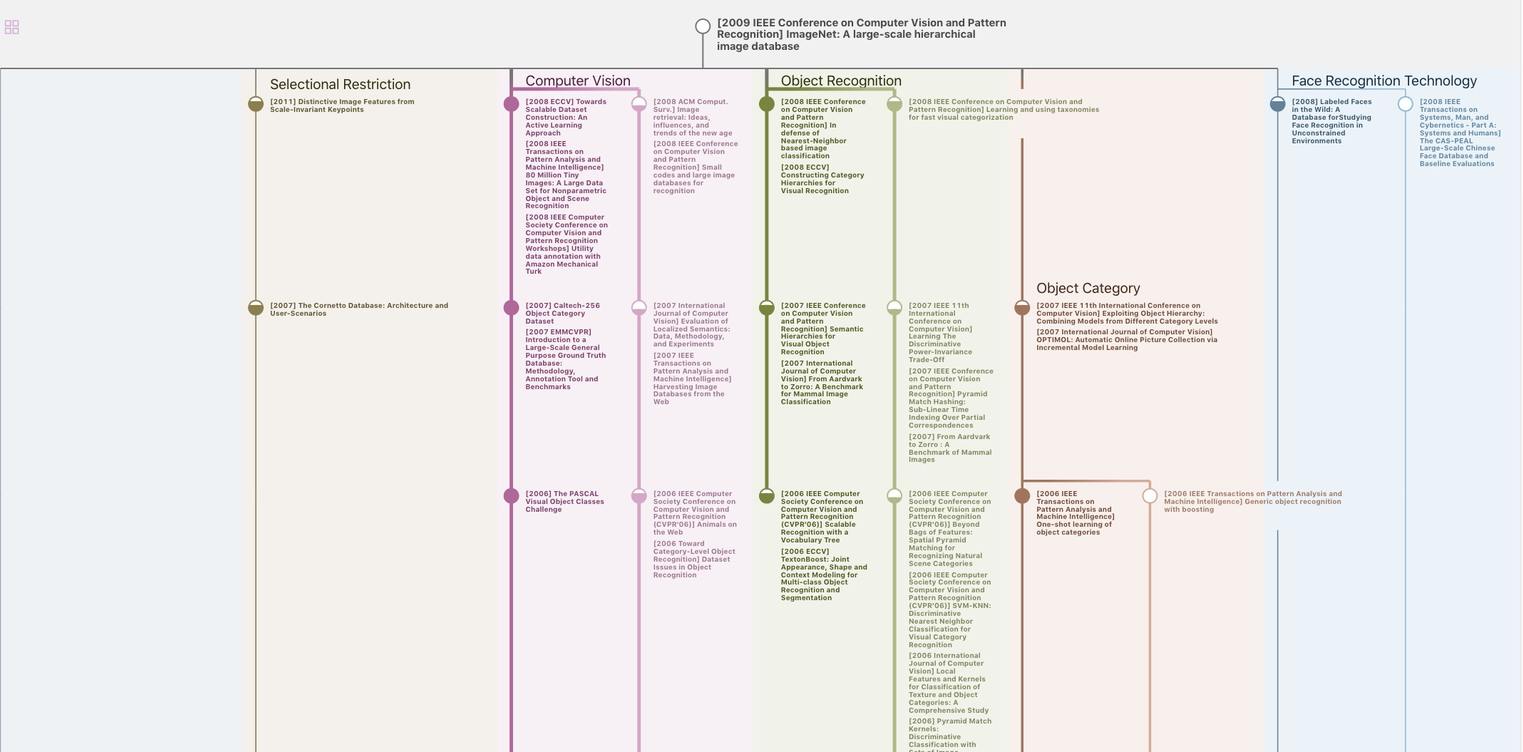
生成溯源树,研究论文发展脉络
Chat Paper
正在生成论文摘要