Semantic-based Attention model for Hospital Readmission Prediction.
Big Data(2022)
摘要
Unexpected hospital readmissions are problematic to both hospitals and patients, and also costly in terms of money and resources as well. Thus, identifying whether or not a patient will be readmitted becomes an important task. Due to the limitation of traditional machine learning techniques, Recurrent neural networks (RNN) and attention mechanisms have been proposed to learn temporal relationships between patient admissions for readmission prediction. Especially, learning accurate representations to represent medical concepts plays a crucial role for generating accurate predictions. Existing works demonstrate that incorporating medical ontologies can be beneficial to learn accurate medical code representations. However, current works ignore the importance of the textual descriptions associated with the medical codes in medical ontologies. In fact, these textual descriptions provide valuable information about the meaning of the medical codes and also the semantic relations among them. In this paper, we propose a new model called Semantic-based Attention model for Medical Concept Embedding (SAMCE). It takes into consideration the semantic information provided in code descriptions to learn medical code representations based on which to generate readmission prediction via RNN and attention mechanisms. Experimental results show that the proposed SAMCE model improves not only the performance of readmission prediction but also the quality of medical code representations.
更多查看译文
关键词
Hospital readmission,medical code representation,medical ontology,semantic,attention mechanism,deep learning
AI 理解论文
溯源树
样例
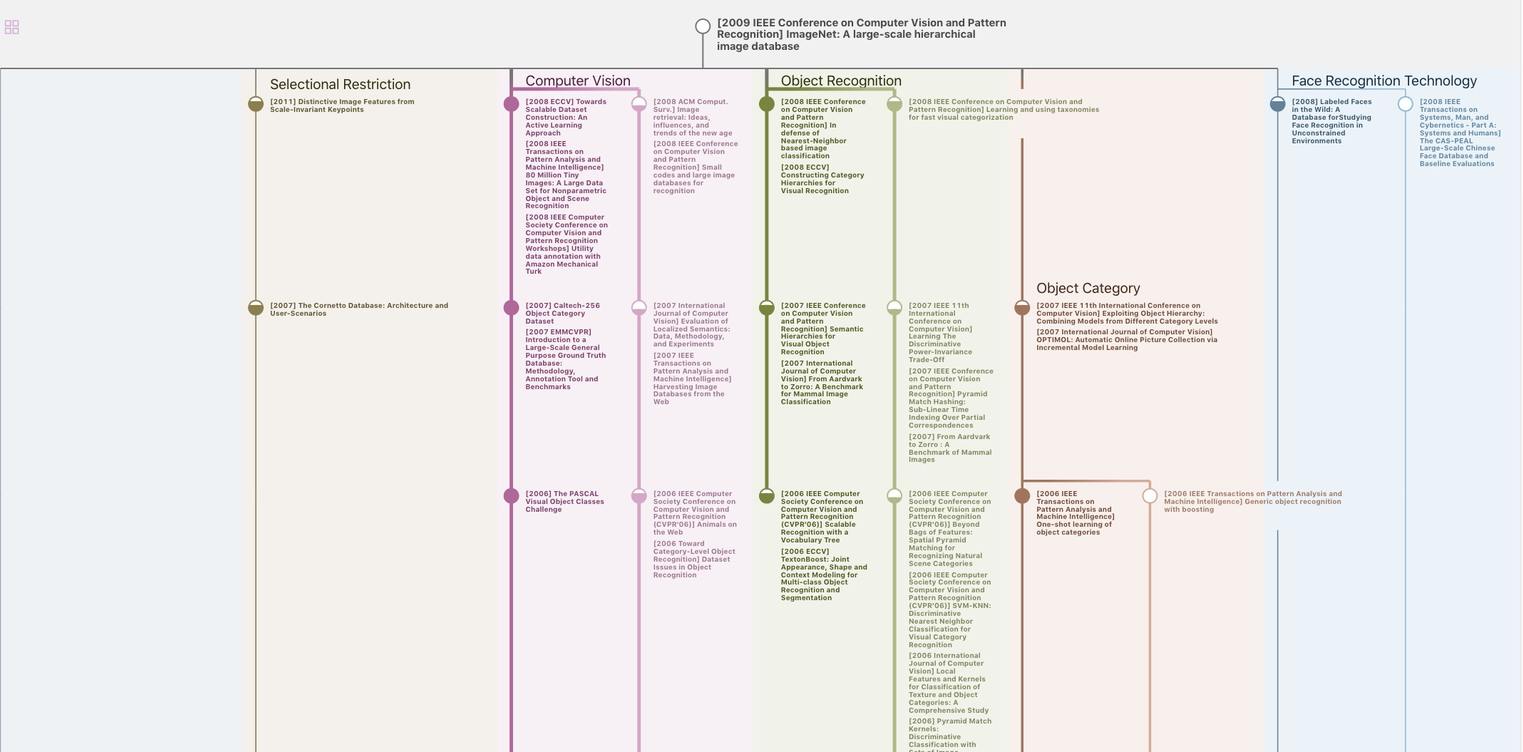
生成溯源树,研究论文发展脉络
Chat Paper
正在生成论文摘要