Accurate Stock Movement Prediction with Self-supervised Learning from Sparse Noisy Tweets
2022 IEEE International Conference on Big Data (Big Data)(2022)
摘要
Given historical stock prices and sparse tweets, how can we accurately predict stock price movement? Many market analysts strive to use a large amount of information for stock price prediction, and Twitter is one of the richest sources of information presenting real-time opinions of people. However, previous works that use tweet data in stock movement prediction have suffered from two limitations. First, the number of tweets is heavily biased towards only a few popular stocks, and most stocks have insufficient evidence for accurate price prediction. Second, many tweets provide noisy information irrelevant of actual price movement, and extracting reliable information from tweets is as challenging as predicting stock prices.In this paper, we propose SLOT (Self-supervised Learning of Tweets for Capturing Multi-level Price Trends), an accurate method for stock movement prediction. SLOT has two main ideas to address the limitations of previous tweet-based models. First, SLOT learns embedding vectors of stocks and tweets in the same semantic space through self-supervised learning. The embeddings allow us to use all available tweets to improve the prediction for even unpopular stocks, addressing the sparsity problem. Second, SLOT learns multi-level relationships between stocks from tweets, rather than using them as direct evidence for prediction, making it robust to the unreliability of tweets. Extensive experiments on real world datasets show that SLOT provides the state-of-the-art accuracy of stock movement prediction.
更多查看译文
关键词
stock price movement prediction,self-supervised learning,Twitter,attention LSTM,time series forecasting
AI 理解论文
溯源树
样例
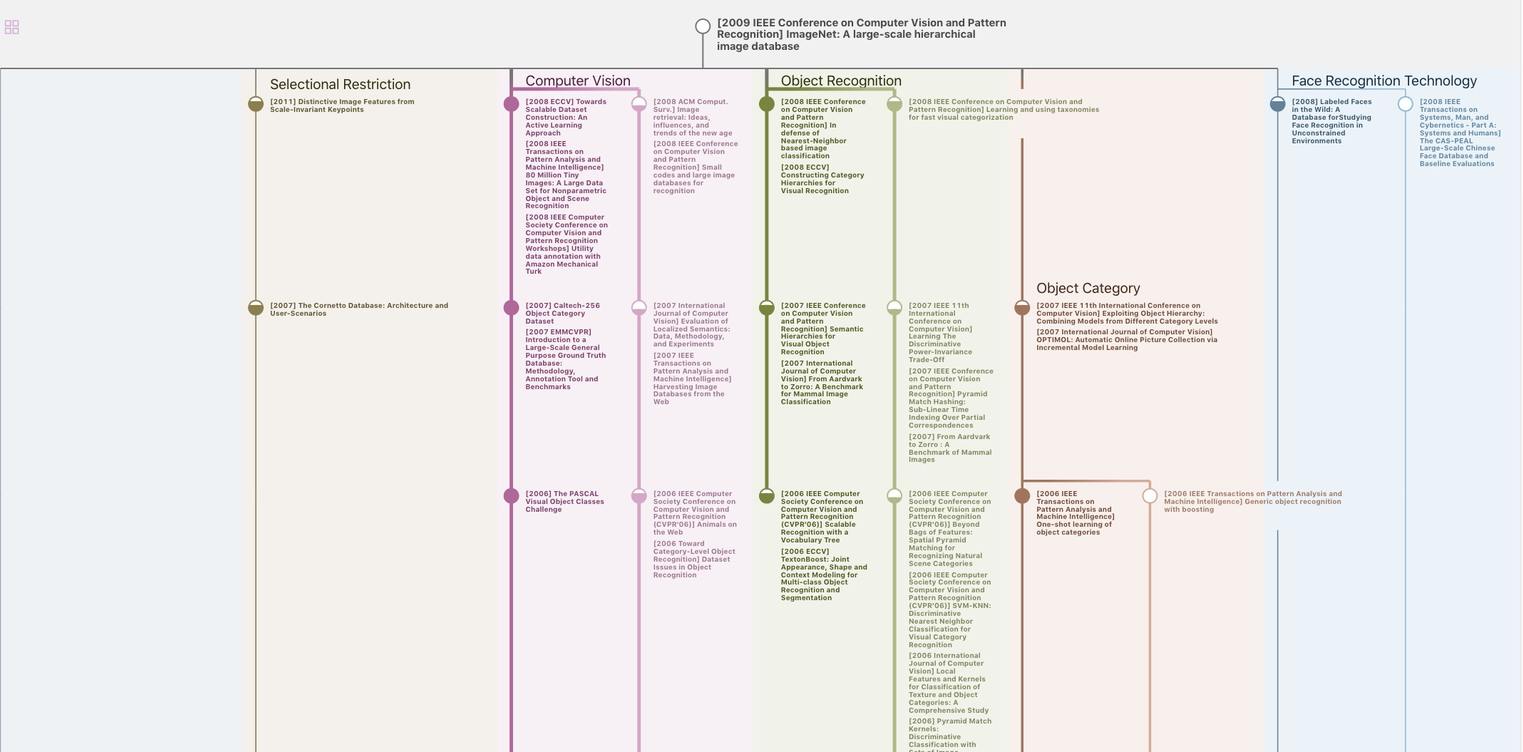
生成溯源树,研究论文发展脉络
Chat Paper
正在生成论文摘要