Layer-wise Knowledge Distillation for Cross-Device Federated Learning.
ICOIN(2023)
摘要
Federated Learning (FL) has been proposed as a decentralized machine learning system where multiple clients jointly train the model without sharing private data. In FL, the statistical heterogeneity among devices has become a crucial challenge, which can cause degradation in generalization performance. Previous FL approaches have proven that leveraging the proximal regularization at the local training process can alleviate the divergence of parameter aggregation from biased local models. In this work, to address the heterogeneity issues in conventional FL, we propose a layer-wise knowledge distillation method in federated learning, namely, FedLKD, which regularizes the local training step via the knowledge distillation scheme between global and local models utilizing the small proxy dataset. Hence, FedLKD deploys the layer-wise knowledge distillation of the multiple devices and the global server as the clients' regularized loss function. A layer-wise knowledge distillation mechanism is introduced to update the local model to exploit the common representation from different layers. Through extensive experiments, we demonstrate that FedLKD outperforms the vanilla FedAvg and FedProx on three federated datasets.
更多查看译文
关键词
Federated Learning, Knowledge Distillation
AI 理解论文
溯源树
样例
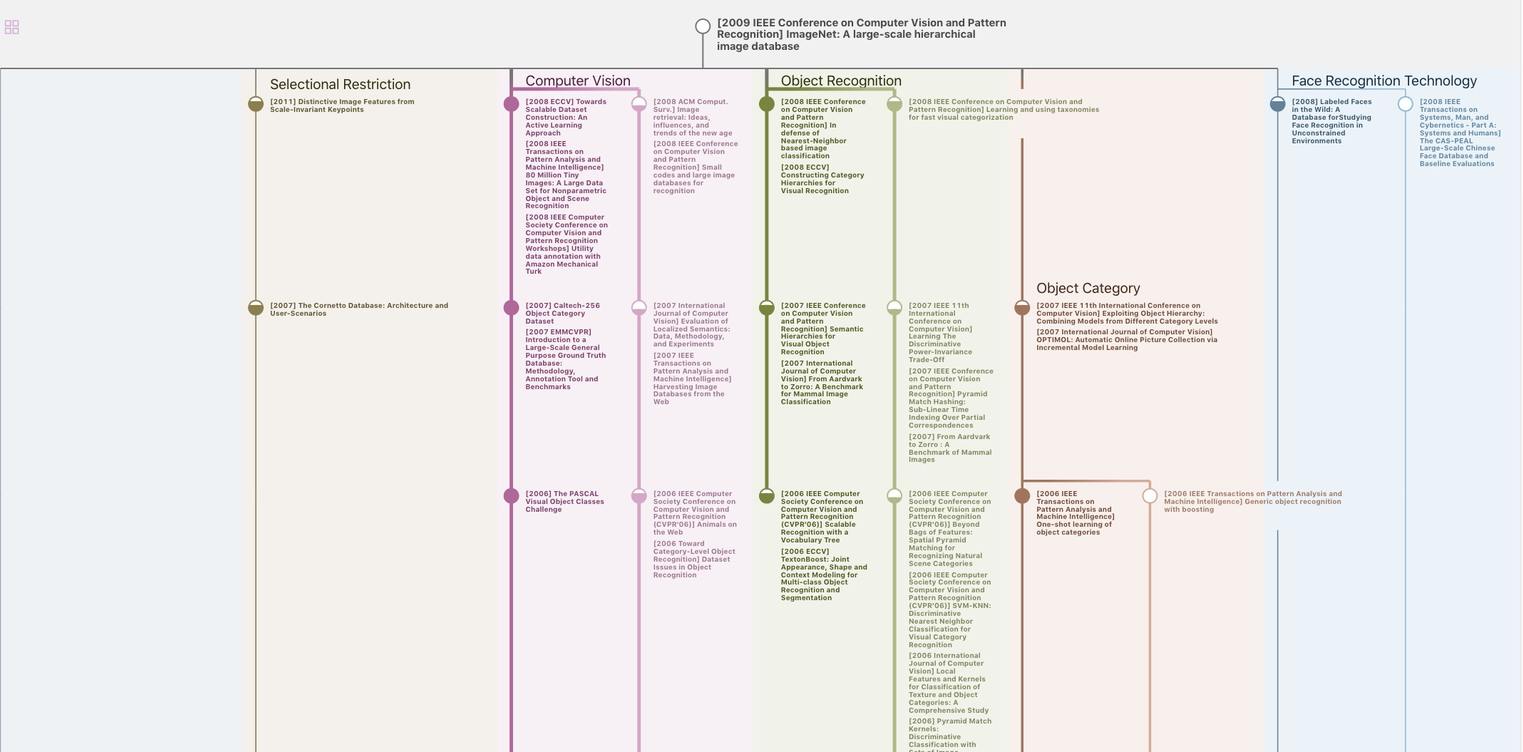
生成溯源树,研究论文发展脉络
Chat Paper
正在生成论文摘要