Robust Unsupervised Domain Adaptation from A Corrupted Source.
ICDM(2022)
摘要
Unsupervised Domain Adaptation (UDA) provides a promising solution for learning without supervision, which transfers knowledge from relevant source domains with accessible labeled training data. Existing UDA solutions hinge on clean training data with a short-tail distribution from the source domain, which can be fragile when the source domain data is corrupted either inherently or via adversarial attacks. In this work, we propose an effective framework to address the challenges of UDA from corrupted source domains in a principled manner. Specifically, we perform knowledge ensemble from multiple domain-invariant models that are learned on random partitions of training data. To further address the distribution shift from the source to the target domain, we refine each of the learned models via mutual information maximization, which adaptively obtains the predictive information of the target domain with high confidence. Extensive empirical studies demonstrate that the proposed approach is robust against various types of poisoned data attacks while achieving high asymptotic performance on the target domain.
更多查看译文
关键词
Poison Data Attack,Robust Learning,Unsupervised Domain Adaptation
AI 理解论文
溯源树
样例
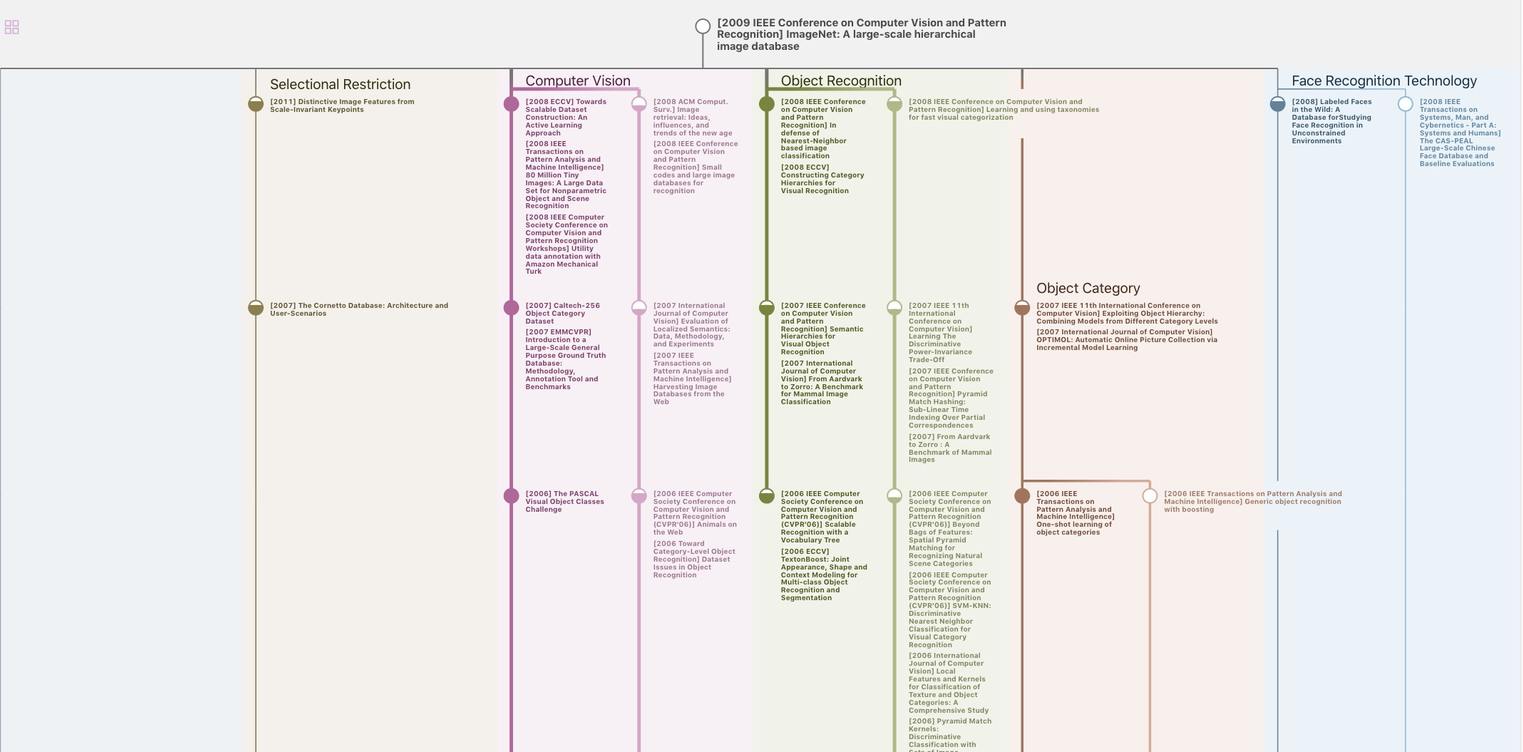
生成溯源树,研究论文发展脉络
Chat Paper
正在生成论文摘要