Evaluation of Incremental Entity Extraction with Background Knowledge and Entity Linking
IJCKG(2022)
摘要
Named entity extraction is a crucial task to support the population of Knowledge Bases (KBs) from documents written in natural language. However, in many application domains, these documents must be collected and processed incrementally to update the KB as more data are ingested. In some cases, quality concerns may even require human validation mechanisms along the process. While very recent work in the NLP community has discussed the importance of evaluating and benchmarking continuous entity extraction, it has proposed methods and datasets that avoid Named Entity Linking (NEL) as a component of the extraction process. In this paper, we advocate for batch-based incremental entity extraction methods that can exploit NEL with a background KB, detect mentions of entities that are not present in the KB yet (NIL mentions), and update the KB with the novel entities. Based on this assumption, we present a methodology to evaluate NEL-based incremental entity extraction, which can be applied to a "static" dataset for evaluating NEL into a dataset for evaluating incremental entity extraction. We apply this methodology to an existing benchmark for evaluating NEL algorithms, and evaluate an incremental extraction pipeline that orchestrates different strong state-of-the-art and baseline algorithms for the tasks involved in the extraction process, namely, NEL, NIL prediction, and NIL clustering. In presenting our experiments, we demonstrate the increased difficulty of the information extraction task in incremental settings and discuss the strengths of the available solutions as well as open challenges.
更多查看译文
关键词
Incremental Entity Extraction, Entity Extraction, Knowledge Base Population, Named Entity Linking
AI 理解论文
溯源树
样例
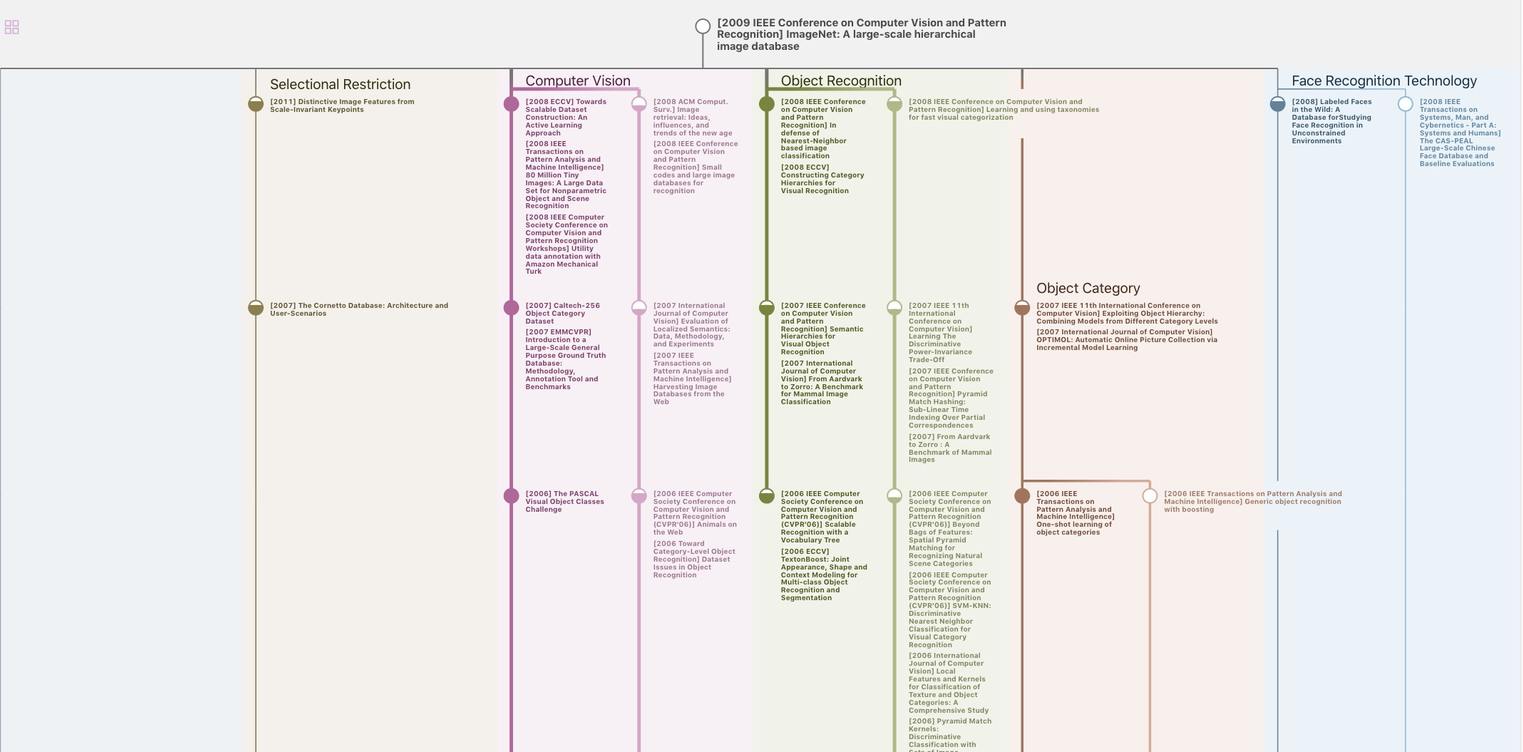
生成溯源树,研究论文发展脉络
Chat Paper
正在生成论文摘要