Topological Data Analysis for Electric Motor Eccentricity Fault Detection.
IECON(2022)
摘要
In this paper, we develop topological data analysis (TDA) method for motor current signature analysis (MCSA), and apply it to induction motor eccentricity fault detection. We introduce TDA and present the procedure of extracting topological features from time-domain data that will be represented using persistence diagrams and vectorized Betti sequences. The procedure is applied to induction machine phase current signal analysis, and shown to be highly effective in differentiating signals from different eccentricity levels. With TDA, we are able to use a simple regression model that can predict the fault levels with reasonable accuracy, even for the data of eccentricity levels that are not seen in the training data. The proposed method is model-free, and only requires a small segment of time-domain data to make prediction. These advantages make it attractive for a wide range of fault detection applications.
更多查看译文
关键词
Electric Machines,Fault Detection,Machine Learning,Topological Data Analysis
AI 理解论文
溯源树
样例
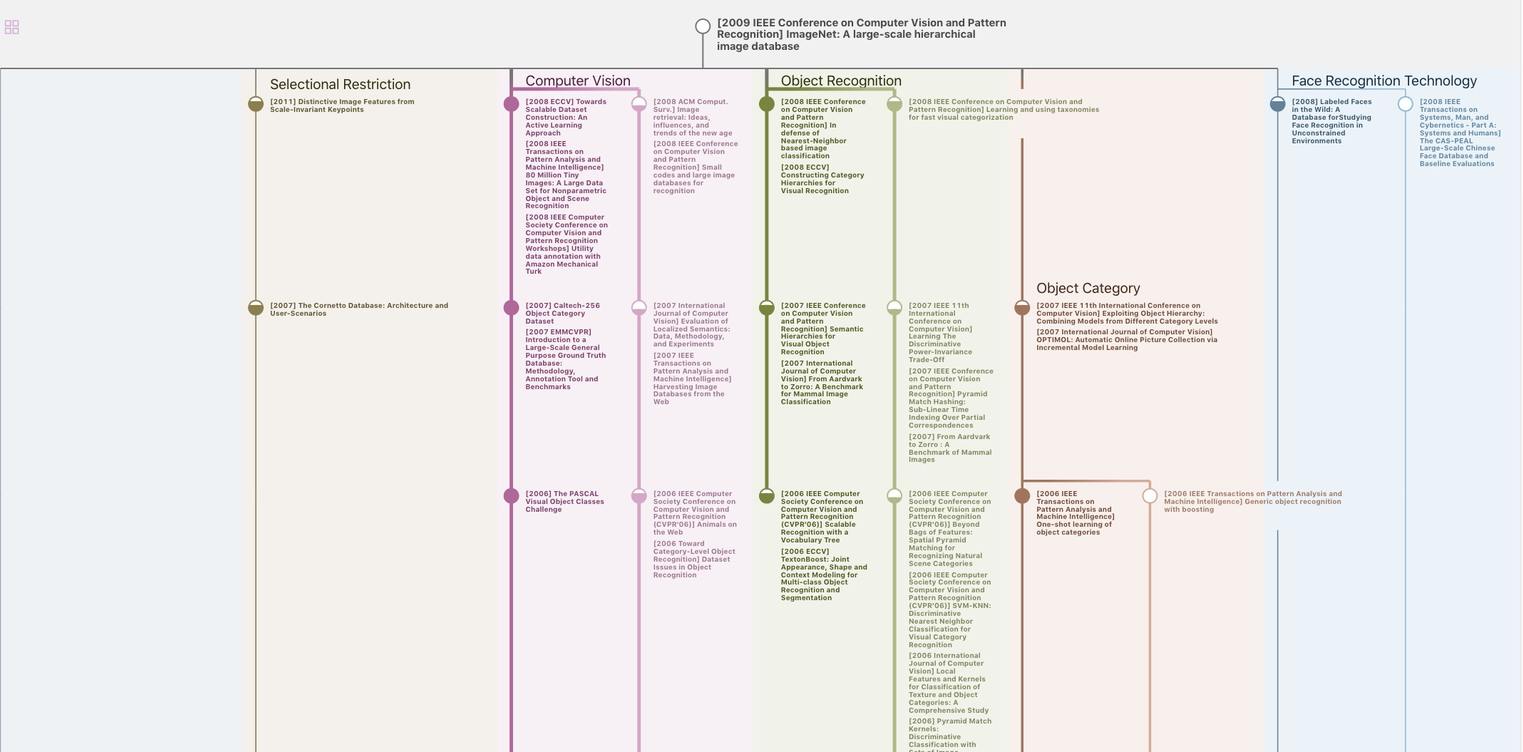
生成溯源树,研究论文发展脉络
Chat Paper
正在生成论文摘要