Compression at the Service of Learning: a Case Study for the Guaranteed Error Machine.
2022 IEEE 61st Conference on Decision and Control (CDC)(2022)
摘要
The scenario approach is a technique for data-driven decision making that has found application in a variety of fields including systems and control design. Although initially conceived in the context of worst-case optimization, the scenario approach has progressively evolved into a general methodology that allows one to keep control on the risk of solutions designed from data according to complex decision processes. In a recent contribution, the theory of compression schemes (a paradigm that plays a fundamental role in statistical learning theory) has been deeply revisited in the wake of the scenario approach, which has led to unprecedentedly sharp generalization and risk quantification results. In this paper, we build on these achievements to gain insight on a classification paradigm called Guaranteed Error Machine (GEM). First, by leveraging the theory of reproducing kernels Hilbert spaces, we introduce a new, more flexible, GEM algorithm, which allows for complex classification geometries. The proposed scheme is then shown to fit into the new compression theory, from which new sharp results for the probability of GEM misclassification are derived in a distribution-free context.
更多查看译文
关键词
complex classification geometries,complex decision processes,compression theory,control design,data-driven decision making,GEM,guaranteed error machine,reproducing kernel Hilbert space,risk quantification,service of learning,statistical learning theory,system design,unprecedentedly sharp generalization,worst-case optimization
AI 理解论文
溯源树
样例
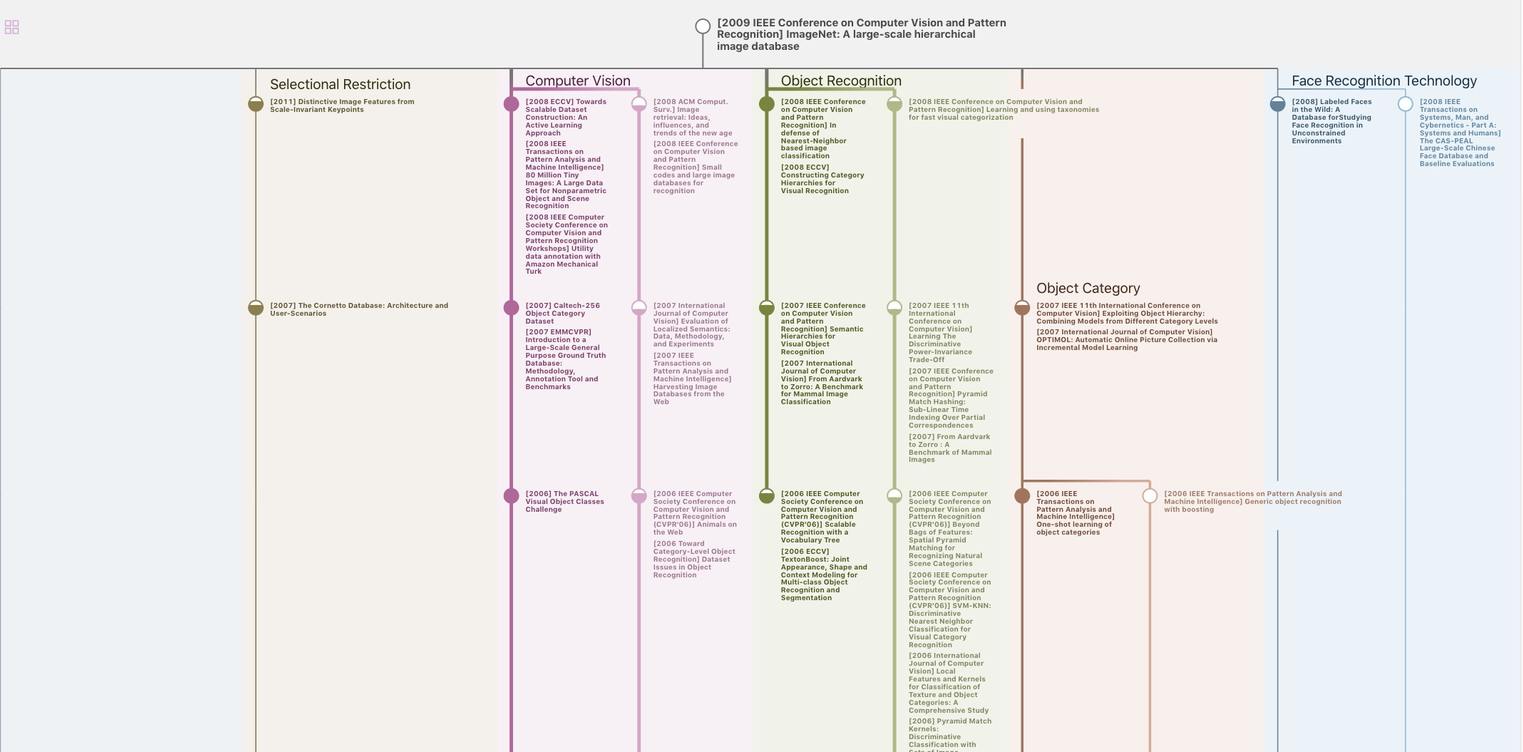
生成溯源树,研究论文发展脉络
Chat Paper
正在生成论文摘要