Physics-guided and Energy-based Learning of Interconnected Systems: from Lagrangian to Port-Hamiltonian Systems
CDC(2022)
摘要
This paper presents a framework for physics-informed energy-based neural network (NN) design to learn models of interconnected systems under the port-Hamiltonian (pH) formalism. In particular, this paper focuses on mechanical systems and incorporates the physical knowledge of Lagrangians into the neural networks to facilitate learning of equations of motion from the data. Moreover, the transformation from the Lagrangian mechanics to the Hamiltonian mechanics is incorporated into the NN architecture and learned from the data such that the learned model is compatible with the pH framework. Then, the structure of input-state-output pH models is imposed on the NN, which guarantees the dissipativity of the learned model. Furthermore, modeling interconnected systems is facilitated by the compositionality property of the pH systems. Additionally, the consistency between the Hamiltonian and Lagrangian is employed for the energy estimation to enable energy-based control. The proposed approach is shown to be computationally more efficient than the existing Lagrangian-based NN design approaches. Furthermore, the learned models with energy estimation are employed for energy-based model predictive control (MPC) design purpose. Experimental results using single (and double) inverted pendulum on carts show that the proposed learning-based approach can achieve an improved performance of model identification compared to the Lagrangian neural networks, accurate estimation of energies and strong control performance.
更多查看译文
关键词
energy estimation,energy-based model predictive control design,Hamiltonian mechanics,input-state-output pH models,interconnected systems,Lagrangian mechanics,Lagrangian neural networks,Lagrangian-based NN design,mechanical systems,model identification,NN architecture,pH systems,physics-guided learning,physics-informed energy-based neural network design,port-Hamiltonian formalism,port-Hamiltonian systems
AI 理解论文
溯源树
样例
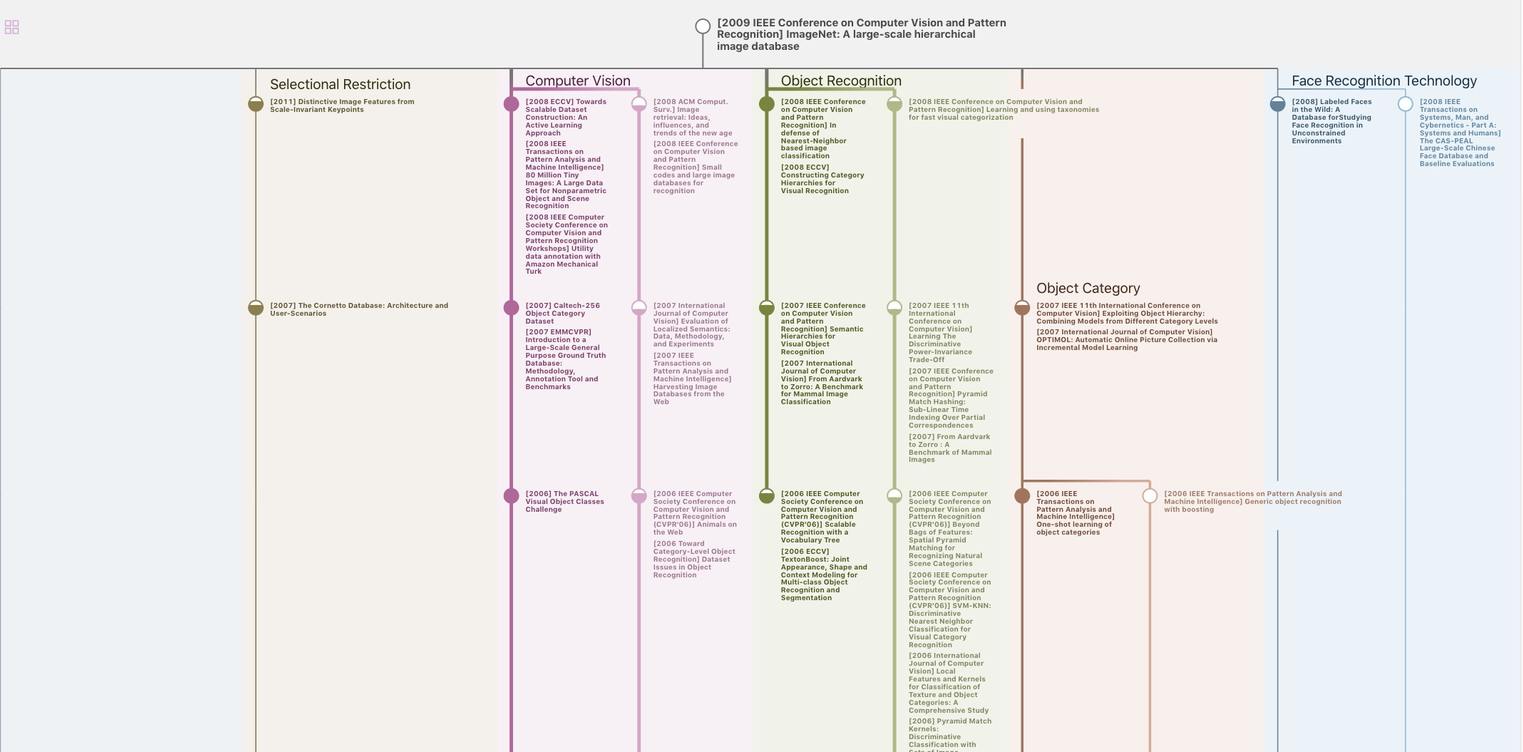
生成溯源树,研究论文发展脉络
Chat Paper
正在生成论文摘要