Neural Network-Based KKL observer for nonlinear discrete-time systems
CDC(2022)
摘要
For non-autonomous multivariable discrete-time nonlinear systems, we address the state estimation problem using a Kazantzis-Kravaris-Luenberger (KKL) observer. We aim to build a mapping that transforms a nonlinear dynamics into a stable linear system modulo an output injection and to design an asymptotic observer. However, this mapping is difficult to compute and its numerical approximation may be badly conditioned during the transient phase. We propose an algorithm based on ensemble learning techniques to improve the numerical approximation of the mapping and its extension in the transient phase. This ensures a good asymptotic convergence of the observer and avoids peaking phenomena. The algorithm demonstrates good performance in high-dimensional and multi-input-multi-output examples.
更多查看译文
关键词
asymptotic convergence,asymptotic observer,discrete-time nonlinear systems,ensemble learning,linear system stability,neural network-based Kazantzis-Kravaris-Luenberger observer,neural network-based KKL observer,nonlinear dynamics,numerical approximation,state estimation problem
AI 理解论文
溯源树
样例
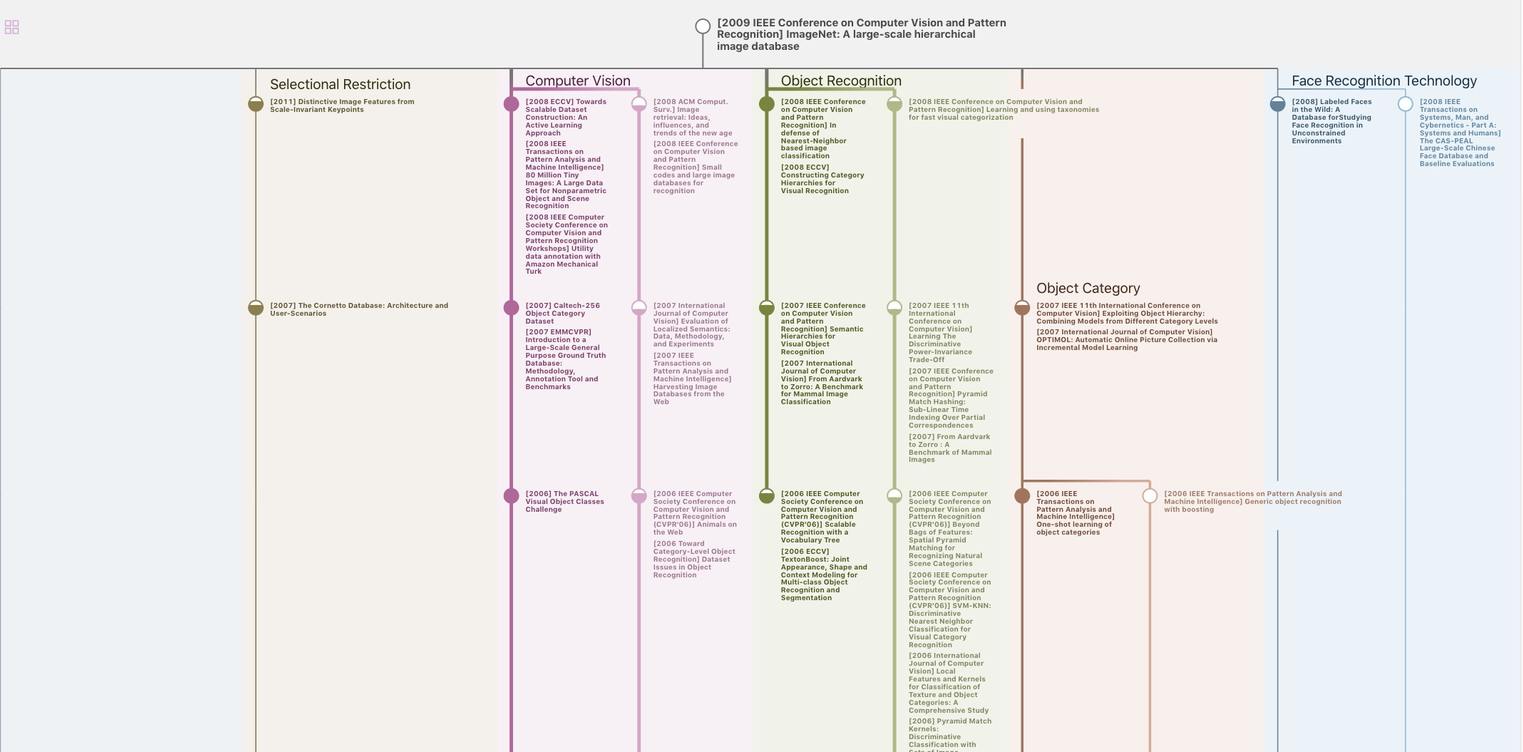
生成溯源树,研究论文发展脉络
Chat Paper
正在生成论文摘要