Generating Minimal Controller Sets for Mixing MMAC.
CDC(2022)
摘要
Multiple model adaptive control (MMAC) is an adaptive control method designed for plant parameter uncertainty given both linear and non-linear plant models. For a system subject to varying operating conditions, the number of controllers necessary to guarantee stable control under nominal-plant uncertainty, or under multiple operating conditions, are both unknown. We propose a learning-based controller synthesis approach that can guarantee stability of a system subject to varying operating conditions. We adopt a convex hull (CH)-based multiple-model controller estimation algorithm that only requires N + M + 1 controllers, where N is the dimension of a compact nominal uncertainty parameter set Theta, and M is the number of additive faults. We empirically validate this result for a quadcopter, which is subject to faults in rotors and sensors as well as to adverse wind conditions.
更多查看译文
关键词
adaptive control method,CH-based multiple-model controller estimation algorithm,compact nominal uncertainty parameter,control stability,convex hull-based multiple-model controller estimation algorithm,learning-based controller synthesis,linear plant model,minimal controller set generation,MMAC mixing,multiple model adaptive control mixing,nominal-plant uncertainty,nonlinear plant model,plant parameter uncertainty
AI 理解论文
溯源树
样例
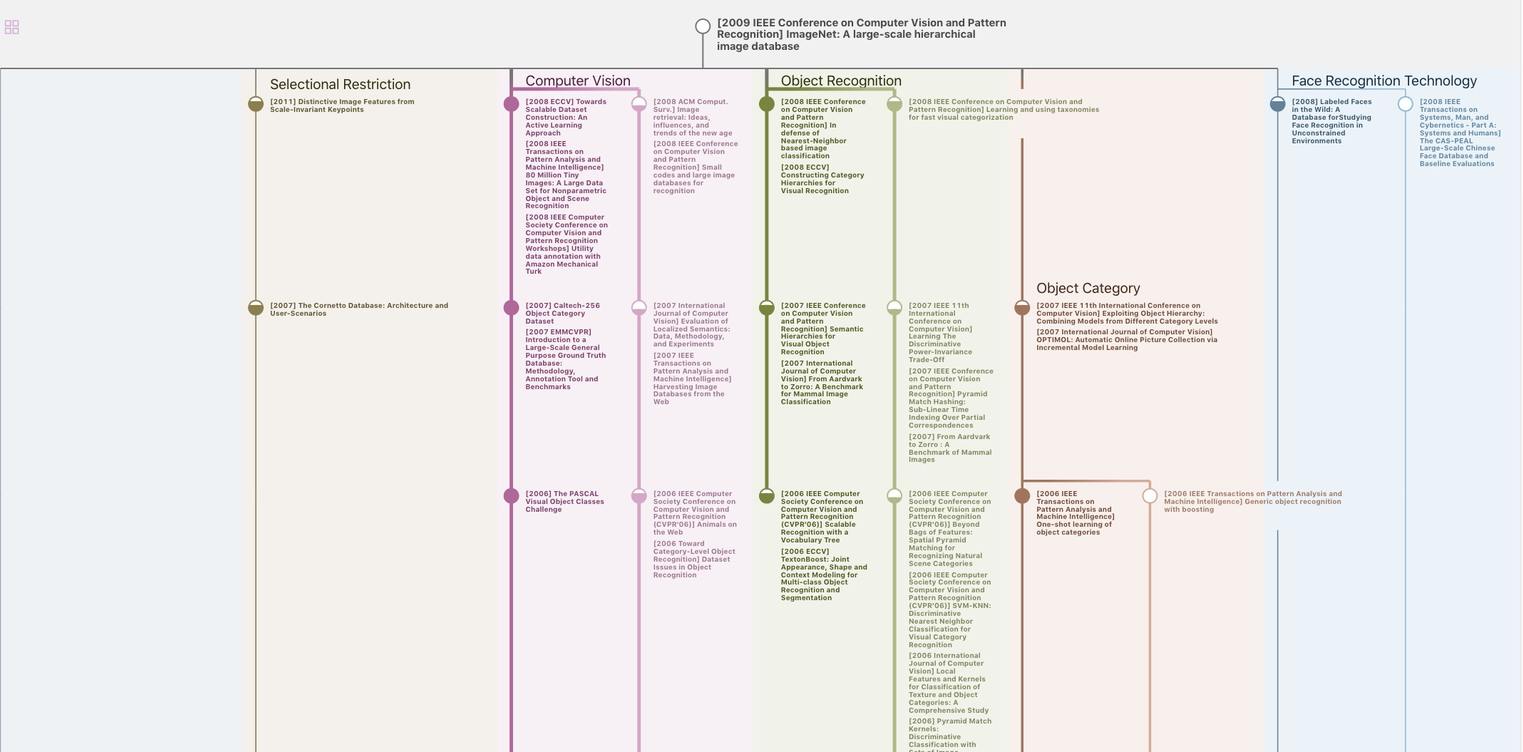
生成溯源树,研究论文发展脉络
Chat Paper
正在生成论文摘要