Study on the incorporation of PCA into EMO for high dimensional multi-objective problems.
SCIS/ISIS(2022)
摘要
It has been known that the dimensionality reduction method is very effective for high dimensional (many design variable) problems in the field of evolutionary computation. Although there are proposed many approaches with this method for single-objective problems, the number of approaches with this method for multi-objective problems is very limited. In multi-objective optimisation, the desgin space to be explored is too large to reduce the number of dimension in the space appropriately. In this research, we focused on principal component analysis (PCA) as a simple dimensionality reduction method and investigated effective ways of incorporating this into evolutionary multi-objective optimization (EMO) algorithms. In this paper, we compared two original incorporated methods and verified the effect of different ways of incorporating; a straight-forward method and a more elaborated method. Because both of our methods are based on MOEA/D, we called these as MOEA/D-PCA and MOEA/D-Modified-PCA(MOEA/D-MPCA). Through applying these to some typical benchmark problems, we confirmed the effectiveness of MOEA/D-MPCA in comparison with the original MOEA/D and MOEA/D-PCA.
更多查看译文
关键词
pca,emo,multi-objective
AI 理解论文
溯源树
样例
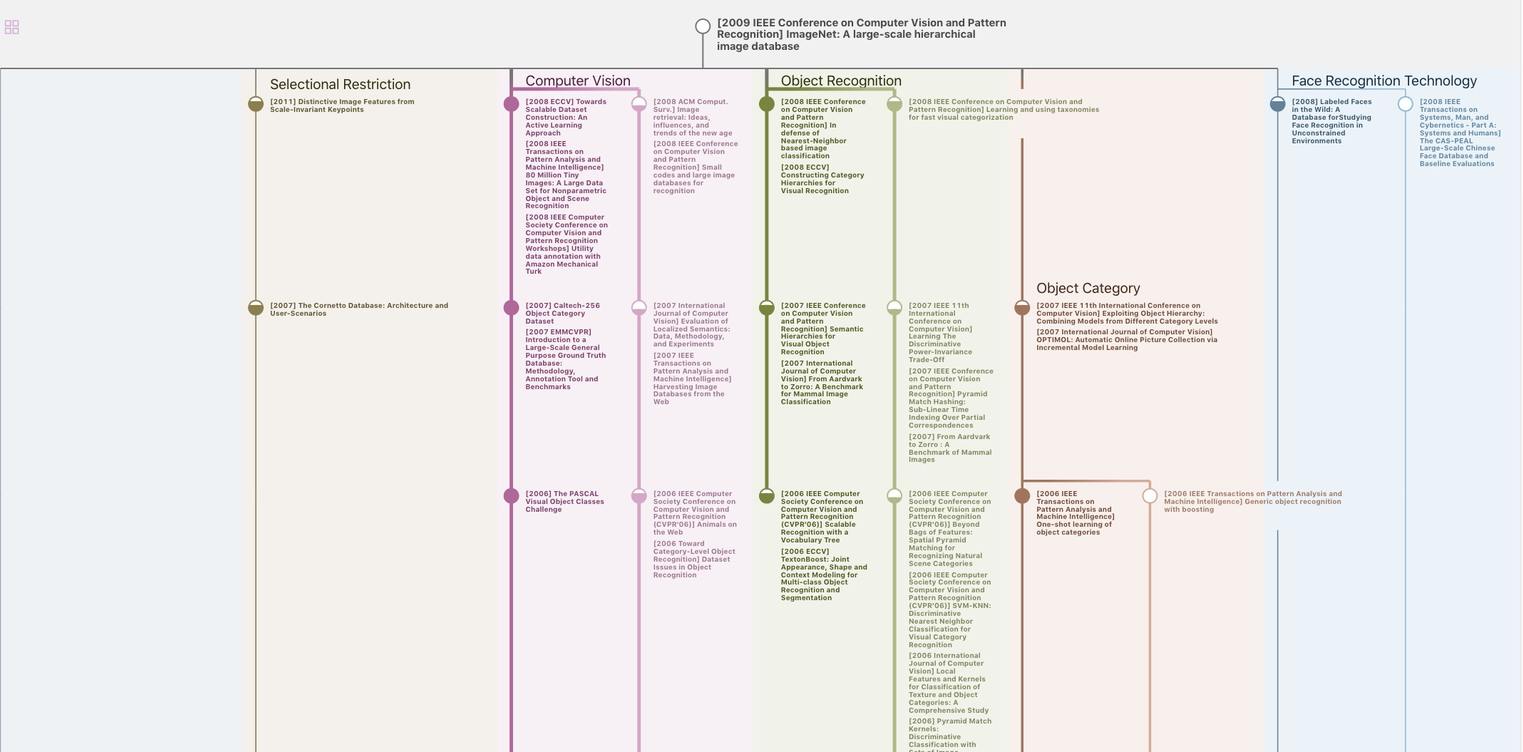
生成溯源树,研究论文发展脉络
Chat Paper
正在生成论文摘要